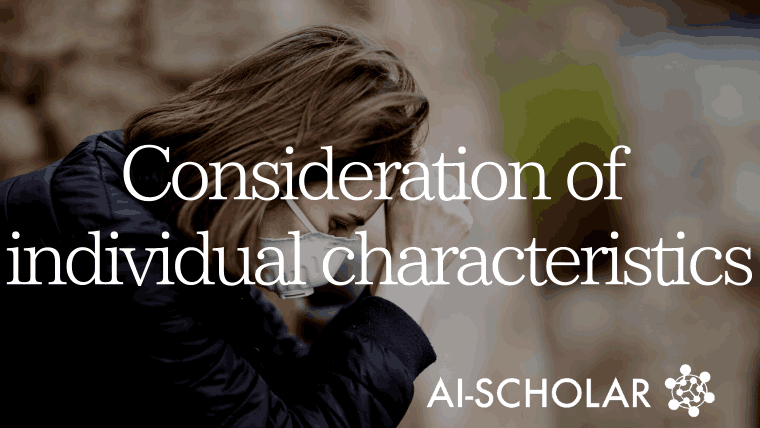
Multiple Treatment Goals And Individual Characteristics Considered Simultaneously! A Proposed Treatment Decision Model For Diabetes Using Deep Reinforcement Learning!
3 main points
✔️ Type II diabetes - T2DM - and complications are likely to be preventable by disease management, but the diversity of treatment targets in T2DM treatment strategies and individual patient differences in decision-making need to be considered
✔️ In this study, we propose a treatment policy derivation model for T2DM based on deep reinforcement learning, considering individual characteristics and diversity of treatment targets
✔️ Results of the evaluation confirmed that the proposed treatment plan led to a treatment plan that was within the appropriate range for blood glucose, blood pressure, and blood lipid control.
Effective Treatment Recommendations for Type 2 Diabetes Management Using Reinforcement Learning: Treatment Recommendation Model Development and Validation
written by
(Submitted on 22 Jul 2021)
Comments: J Med Internet Res
The images used in this article are from the paper, the introductory slides, or created based on them.
backdrop
Is it possible to derive an optimal treatment strategy that takes into account individual characteristics and diverse treatment targets?
In this study, we aim to construct a model for deriving a treatment strategy for type II diabetes based on reinforcement learning, which can be adapted to individual characteristics and various treatment targets.
Type 2 diabetes mellitus - T2DM - is a chronic disease characterized by high glycemic status, leading to multiple complications and increasing the risk of death. While these complications are likely to be preventable with disease management, the impact of individual characteristics and the diversity of treatment targets point to a gap between recommended and actual treatment - making it difficult to determine a uniform treatment strategy and to provide personalized treatment. The need, In addition, diabetes is a chronic condition that requires long-term treatment, making decision-making more complex due to the following factors: a.
(1) the effects of a single treatment are not immediately reflected, and (2) patients have a wide range of options for the treatment program they receive.
In this study, we aim to construct a model that can consider individual characteristics and various treatment goals by using reinforcement learning (RL) to derive optimal policies.
What is Type II Diabetes - T2DM -?
First, a brief description of type II diabetes mellitus, the subject of analysis in this study, is given.
Blood glucose refers to the concentration of glucose - sugar or glucose - in the blood and how much sugar is in the blood. These substances are used as energy for daily activities and spike after a meal and then slowly return to normal. On the other hand, if the blood glucose level remains high - the amount of sugar in the blood - due to factors such as abnormal glucose tolerance, vascular damage can occur - the breakdown of blood vessel walls, the formation and rupture of blood clots, and so on. In addition, due to the effects on internal organs, brain function, and blood pressure, the possibility of major damage to organs with many capillaries - such as the kidneys, brain, and liver - and organs with large blood vessels - such as the heart - increases. The following are some of the reasons for this. This condition of high blood sugar is called abnormal blood sugar - diabetes.
Diabetes mellitus is caused by two factors and is called differently depending on these factors: a condition in which the pancreas is not functioning properly and therefore produces less insulin to get sugar into the cells - insulin hyposecretion, type I diabetes; and a condition in which the doors to get sugar into the cells are not working properly - insulin hyposecretion, type II diabetes. Symptoms of insulin resistance and type II diabetes. Insulin is like a "key" for getting sugar into cells. In the former case, the production of the key decreases and the sugar concentration in blood vessels increases - the cause is thought to be a decrease in insulin secretion mainly in the pancreas, and heredity is pointed out as the cause; on the other hand, in the latter case, excessive Blood sugar causes the locks that open cell doors to fail - often due to lifestyle factors such as overeating and obesity. Type II diabetes is commonly referred to as diabetes mellitus.
research purpose
In this study, we aim to construct a derivation algorithm for the treatment policy that takes into account individual characteristics and various treatment goals by using reinforcement learning.
In the treatment of T2DM, individualized treatment is needed because it is difficult to make uniform treatment decisions due to the influence of diverse treatment goals and individual differences. In this study, we aim to construct a learning model to derive an optimal treatment policy that reflects individual characteristics and can take multiple treatment goals into account by using RL. -The model was constructed by applying the deep RL algorithm to the dataset The model was trained on three treatment targets for T2DM: antihyperglycemic treatment, antihypertensive treatment, and lipid-lowering treatment.
technique
In this section, we give an overview of our model.
data-set
The data used was collected from the Singapore Health Service Diabetes Registry, which encompasses 189,520 T2DM patients, including 6,407,958 outpatient visits from 2013 to 2018. The dataset included study training data on three treatment guidelines - antihyperglycaemic, antihypertensive, and lipid-lowering therapy - 80%, 152,527 patients - and a test to assess the effectiveness of these treatments Data - 20%, 36,993 patients - were split into Each patient's EMR data included demographic information, medical history, physical measurements, laboratory data, and physician prescriptions, as well as laboratory data such as glycated hemoglobin A1c - HbA1c-, low-density lipoprotein cholesterol - LDL-c -, and fasting blood glucose levels are included.
clinical outcome
Two types of clinical outcomes were defined in this model: short-term outcomes, including glycemic control, blood pressure control, blood lipid control, and hypoglycemia-related hospitalizations; and long-term outcomes, including myocardial infarction, heart failure, stroke-ischemic, or hemorrhagic stroke The incidence of diabetic complications and death, including diabetic nephropathy, diabetic nephropathy, and other diabetic complications.
proposed model
The proposed model takes clinical information at the time of presentation as input and derives the optimal treatment strategy with three types of therapies as outputs - antihyperglycemic, antihypertensive and lipid-lowering medications - Input includes demographic information, laboratory data, physical measurements, medical history, and current prescriptions. To build a model that makes comprehensive treatment recommendations, we combine the outputs of the three models.
In addition, to accommodate individual characteristics and multiple treatment goals, this model combines knowledge-driven and data-driven models to build a more flexible derivation model: in the former, a model based on clinical guidelines and experts' experience is applied to select candidate drugs; in the latter, a model based on clinical guidelines and experts' experience is applied to select candidate drugs. In the latter, based on the deep RL, candidate drugs were ranked according to their clinical efficacy to derive various treatment strategies. In these models, the former knowledge-driven model is first applied to select candidate drugs, and then the latter data-driven model is used to rank the candidates according to clinical outcomes - see figure below.
The reward function in the proposed model - equation below - was designed based on the following guidelines: the conditions under which the reward is given are (1) HbA1c reaches the control target - <7% - after 3-6 months and (2) No complications or death until the patient's last visit in the next 6 years; the penalizing conditions were (1) HbA1c not well controlled at 3-6 months, (2) hypoglycemic event in the next 6 months, and (3) complications or death after the current visit. The study was set up to
Evaluation method
This section describes the evaluation methodology in this study - the evaluation defined two perspectives, short-term and long-term.
For short-term evaluation, a multivariate regression model was constructed using the model agreement as an exposure factor, and the degree of agreement between physician prescriptions and model-recommended drugs was evaluated. The short-term outcome was evaluated by comparing the two groups in terms of the rate of achievement of goals for glycemic, blood pressure, lipid control, and hypoglycemic events; while the long-term outcome was evaluated by including model concordance rate as an independent variable to evaluate combination therapy with antihyperglycemic, antihypertensive, and lipid-lowering medications. The results of the study are shown in Table 1. Here, the model concordance rate was calculated by dividing the number of patients with a concordant treatment plan by the total number of patients seen. This measure was intended to quantify the extent to which each patient adhered to the model recommendations.
result
This section describes the evaluation results of this study.
Evaluation of short-term outcomes
For the evaluation of short-term outcomes, the model agreement was used as the exposure variable, and the effect of the treatment recommendation model was assessed at the examinee level. Test data were included for different short-term outcomes - the proportion of patients with HbA1c<7%, SBP/DBP<140/90 mmHg, and LDL-c<2.6 mmol/L after 3 to 6 months of treatment. Results showed that in 43.3% of the patients analyzed, the model-recommended treatment strategy for antihyperglycemic agents was consistent with the actual physician policy; whereas the concordance rates for antihypertensive and lipid-lowering agents were 51.3% and 58.9%, respectively, for the patients overall. In addition, for each treatment strategy and prognostic improvement, glycemic control - odds ratio [OR] 1.73, 95% CI 1.69-1.76-, blood pressure control - OR 1.26, 95% CI 1.23-1.29 OR 1.28, 95% CI 1.22-1.35) -, and blood lipid control - OR 1.28, 95% CI 1.22-1.35) -, confirming the prognostic benefit of the proposed model.
Evaluation for long-term outcomes
For the assessment of long-term outcomes, we evaluated the model concordance rates of patients and the incidence of long-term outcomes for antihyperglycemic, antihypertensive, and lipid-lowering therapy in all patients - see figure below: model concordance rates were negatively correlated with the incidence of complications and death. The higher the model agreement, the lower the rate of complications and death.
We also evaluated the impact of model agreement for the combined antihyperglycemic, antihypertensive, and lipid-lowering treatment on the reduction in risk of complications and mortality for the three types of treatment by multivariate regression. The results confirmed that the predictive model based on XGBoost outperformed the clinical baseline model, showing an area under the receiver operating characteristic curve of 0.71 to 0.87. In addition, the model concordance rate for each treatment was negatively correlated with the occurrence of major complications and death - these results showed that patients who received treatment more similar to the model recommendations were more likely to have diabetic complications - i.g. macrovascular complications and microvascular complications -and a decreased risk of death.
consideration
In this study, we proposed a model for deriving a treatment strategy that takes into account individual characteristics and multiple treatment goals of T2DM, utilizing a large dataset collected from a medical cluster. The evaluation results showed that the antihyperglycemic drugs by the proposed model were identical to the actual prescriptions in 43.3% of the cases. In addition, the evaluation of treatment recommendations showed that blood glucose - OR 1.73-, blood pressure - OR 1.26- and blood lipids - OR 1.28- were controlled by the and the risk of diabetic complications remained low - these assessment results suggest that the proposed model is likely to lead to prescribing drugs that can achieve better outcomes. The results also confirmed a trend toward improved long-term outcomes, including the risk of death, suggesting that the proposed model may be useful in reducing the risk of diabetic complications and improving clinical outcomes.
Two strengths of this study can be identified: first, the quality of the dataset used for model building and evaluation. The dataset utilized in this study consists of the medical records of a large patient population - electronic health records - and includes different types of diabetic complications - macrovascular and microvascular - from a 6-year study. There is limited precedent for learning and evaluation on a data set of this size, which is one of the unique features of this study; second, it covers a comprehensive treatment strategy for diabetes. The study included three types of therapy-antihyperglycemic, antihypertensive, and lipid-lowering-and evaluated two types of outcomes: short-term control of key indicators and long-term development of diabetic complications. These assessments allow for a more general derivation model for diabetes treatment strategies.
On the other hand, there are two main challenges: bias due to EMR; and setting uniform treatment goals. The present study is based on EMRm in patients with T2DM and may lack information that influences the choice of prescribing - i.g. repeating previous prescriptions for patients who are reluctant to change their medication. These patient preferences are not recorded in the dataset utilized in this study and may act as a bias against the treatment strategy - therefore, a possible solution could be additional research to include these biases and design a linear model that accounts for confounding. Second, because uniform treatment targets were used in this study, the HbA1c targets may not be exact - depending on the patient's condition, they may not be serious. A possible solution to this could be to focus the study more on individual characteristics, such as targeting a dynamic regime.
Categories related to this article