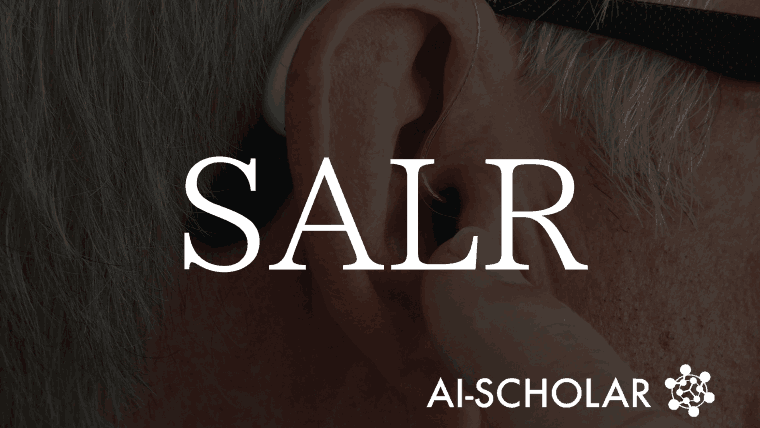
Automated Classification Of Dysarthria Severity! Experimental Introduction Of SALR, Which Outperforms Even Wav2vec2
3 main points
✔️ New Objective Aphasia Severity Assessment Method Using Transformer Model
✔️ Speaker-Agnostic Latent Regularization (SALR)
✔️ Achieves a high accuracy rate of 70.48%, significantly higher than the conventional method
written by Lauren Stumpf,Balasundaram Kadirvelu,Sigourney Waibel,A. Aldo Faisal
[Submitted on 29 Feb 2024]
Comments: 17 pages, 2 tables, 4 main figures, 2 supplemental figures, prepared for journal submission
Subjects: Neurons and Cognition (q-bio.NC); Artificial Intelligence (cs.AI); Computation and Language (cs.CL); Machine Learning (cs.LG); Sound (cs.SD); Audio and Speech Processing (eess.AS)
The images used in this article are from the paper, the introductory slides, or were created based on them.
Introduction
Nice to meet you all!
I am Ogasawara, a new writer for AI-SCHILAR.
The paper presented here is this one
Speaker-Independent Dysarthria Severity Classification using Self-Supervised Transformers and Multi-Task Learning.
It is.
As I summarized the main points at the beginning of this article, it seems that the objective is to automatically evaluate the severity of dysarthria in an objective manner.
I wonder what kind of methods are being used! Let's learn together with me little by little~~!
I will try to introduce them in as concise a manner as possible, so please bear with me until the end.
Outline of this study
Dysarthria is a condition in which the control of speech muscles is impaired and has a significant impact on the patient's communication and quality of life. The disorder is so complex that human evaluation is inevitably lacking in objectivity.
This study proposes a transformer-based framework that automatically evaluates the severity of a disability from raw speech data. This allows for more objective evaluations than those made by human experts.
Let's keep it in mind
What is dysarthria?
It is a disorder in which congenital or acquired factors prevent a person from pronouncing a language correctly, even though he or she understands it. Acquired factors include stroke or neuromuscular disease, for example.
Speech characteristics vary widely from person to person, but in general, speech intelligibility is reduced and the spoken word is difficult to understand. This makes interpersonal communication extremely difficult.
Until now, the severity of a patient's condition has been determined by subjective auditory evaluation by a speech-language pathologist. However, more objective evaluation methods are being sought.
What is self-supervised learning?
The model used in this paper is called wav2vec2.0. The model is characterized by its ability to automatically learn useful portions of a large amount of unsupervised data. It is particularly useful in the speech domain, where large-scale supervised learning is difficult.
What is Transformer?
It is a processing model that uses Attention mechanism and has achieved excellent results mainly in the areas of natural language processing and speech recognition. Because it is able to capture the context of the entire input, it is considered suitable for modeling speech changes such as dysarthric speech.
Do you understand? Reflection on the past
There are only three important things!
Let's just hold on to this!
- Dysarthria is a disorder in which speech is not produced correctly.
- Self-supervised learning is like very large unsupervised learning.
- Transformer is good at modeling minor changes
As long as you have these three things in mind, the rest will be fine!
In the next section, we will look at the experiment.
This is where we start! About the Experiment
Thank you very much for reading this long explanation of the basics.Next, I will finally explain the most interesting part of the paper, the experiment.
Experimental setup
1: Data set
This study uses UA-Speech, an English speech corpus widely used in dysarthria research. This is an English speech corpus widely used in dysarthria research. There is no free dysarthric speech corpus in Japanese, so the large number of English speakers is remarkable. I wish there was a universal corpus for Japanese as well...
2: Model
The wav2vec2,0 model is fine-tuned to the dysarthria severity classification task. This model has been pre-trained on a 960 hour data set! This cannot be replicated on an individual basis...
Well, there are other preparation items, but they are very in-depth and difficult, so I will omit them in this article.
3: Purpose
The goal is to develop a system that automatically classifies the severity of dysarthria.Accuracy and F1 scores are used in this study to evaluate performance.
What are the results of the experiment?
Yes, I am. From here, we will look at the results of the experiment! The paper contains a table summarizing the results of the experiment, but it was difficult to understand at a glance, so I will give a brief explanation in writing this time.
As a result, the model proposed in this paper in the dysarthria severity classification task showed a significant improvement in classification accuracy from the other models. It seems to have performed better than the fine-tuned wav2vec2 and also showed the best performance as it improved the F1 score as well.
The model was also found to be strong in classifying severity classes of dysarthria at the extremes. For example, it was found to be excellent at classifying very low and very high. However, it seems that there are still issues regarding middle class classification. This is considered to be due to the lack of data for the categories and the lack of clear criteria between classes.
Summary of Dissertation
Hmmm. This is an indescribable result. I think that in classifying disabilities, extreme cases can be done without doctors making mistakes and getting lost. Well, it's a subjective judgment... Even experienced doctors get lost in the middle class classification. I think that if this issue is solved, it will help doctors and help patients to make a decision that both parties can agree on, since they can check objective data.
Nevertheless, it is a good approach to have AI do the classification of disorders. This approach could be adapted to disorders other than dysarthria, and image recognition would be even more useful!
A little chat with a chick writer, Ogasawara
On a personal note, I have decided to enter a Ph!
It is often said on the Internet that PhDs cannot get a job, but that is a very extreme example. You should not think that this is the standard.
My school is a regional national university, so not many people go on to doctoral studies, but graduates seem to be able to fulfill their desired career paths, such as working at research institutes or becoming assistant professors at universities.
Well, I'm a little bit nervous about having another entrance exam in a year, but I'm going to enjoy it with the spirit of "Oh well, I'll make it work out," I guess!
So, my greatest thanks to all the readers who have read this far!
Well then, goodbye!
Categories related to this article