The "passionate Behavior" Of Both The Generation AI And The Users.
3 main points
✔️ Verification of the advantages expressed in the quality of the output of the generated AI
✔️ One case where AI's common sense reasoning reduces processing time
✔️ A case study of the influence of user passion on the behavior of a generative AI making inferences
アナログ素材と3次元デジタル表現のコラボレーション
written by Takahiro Yonemura
本論文の掲載に当たり、掲載元より掲載許可を頂いております。
Introduction
(1) This video explores whether expressions unique to digital technology can gain interest.
(2) The author realized that the number of expression methods and the degree of freedom to take advantage of depth will increase. For reference, the "percentage of users who chose to watch" of the author's public videos averaged 12.4%, and 20.5% for videos utilizing depth expression with AI.
Above (1) and (2) are excerpts from the editorial points. It can be seen that the novel expression utilizing the generative AI gained interest as described in (2). As additional information, the following graph compares the promotional video produced by the author with the promotional video including depth expressions by the generative AI.
The graph plots the viewer rate of general videos compared to the videos we produced (during the first 10 days after the videos were released). The results show that videos that make use of the expressions of generative AI, whose graphs hardly fall (i.e., they can maintain user viewing), are on average more than twice as dominant as videos that do not.
We will now discuss the evolution of generative AI that has led to useful results, as well as specific examples of what users can do regarding evolution and quality.
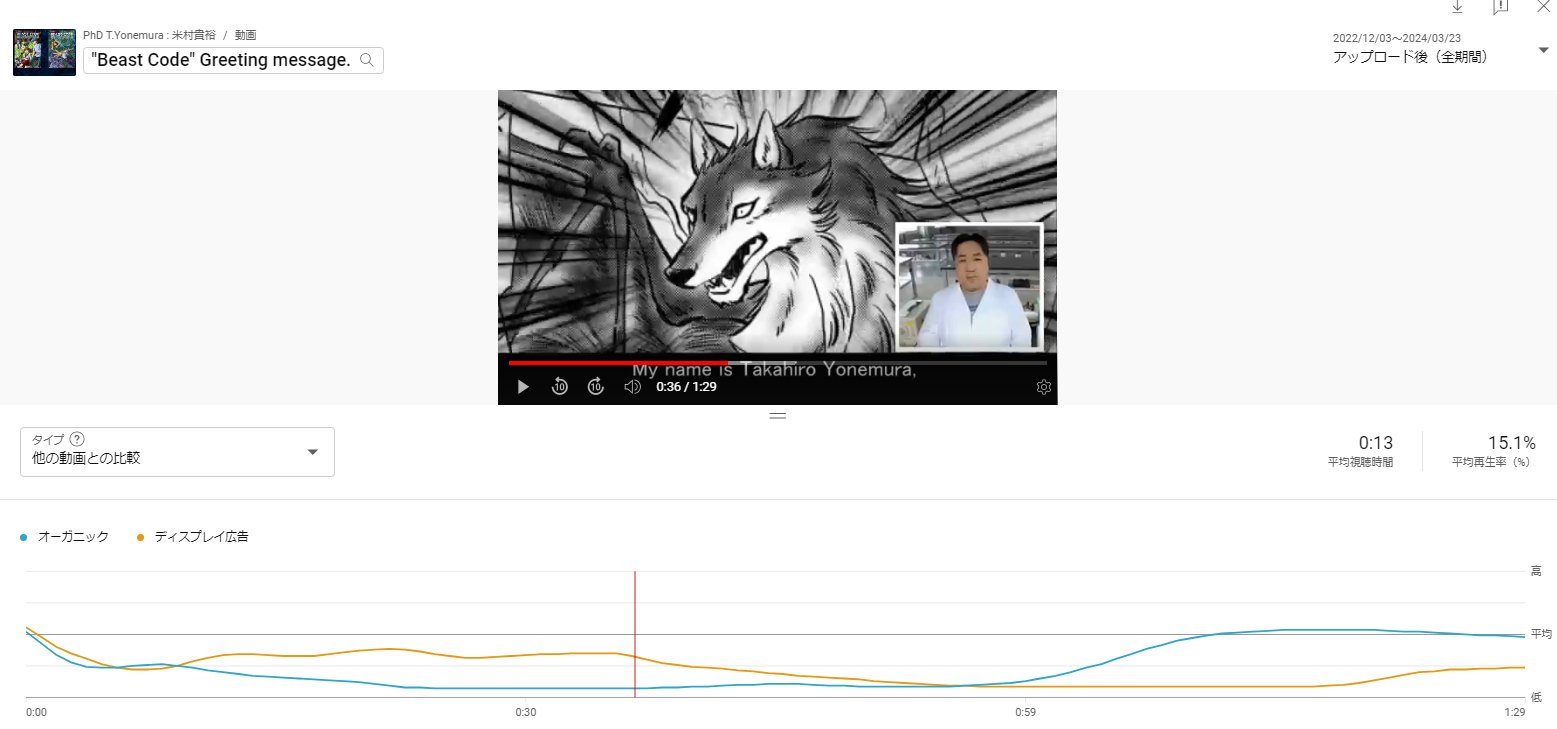
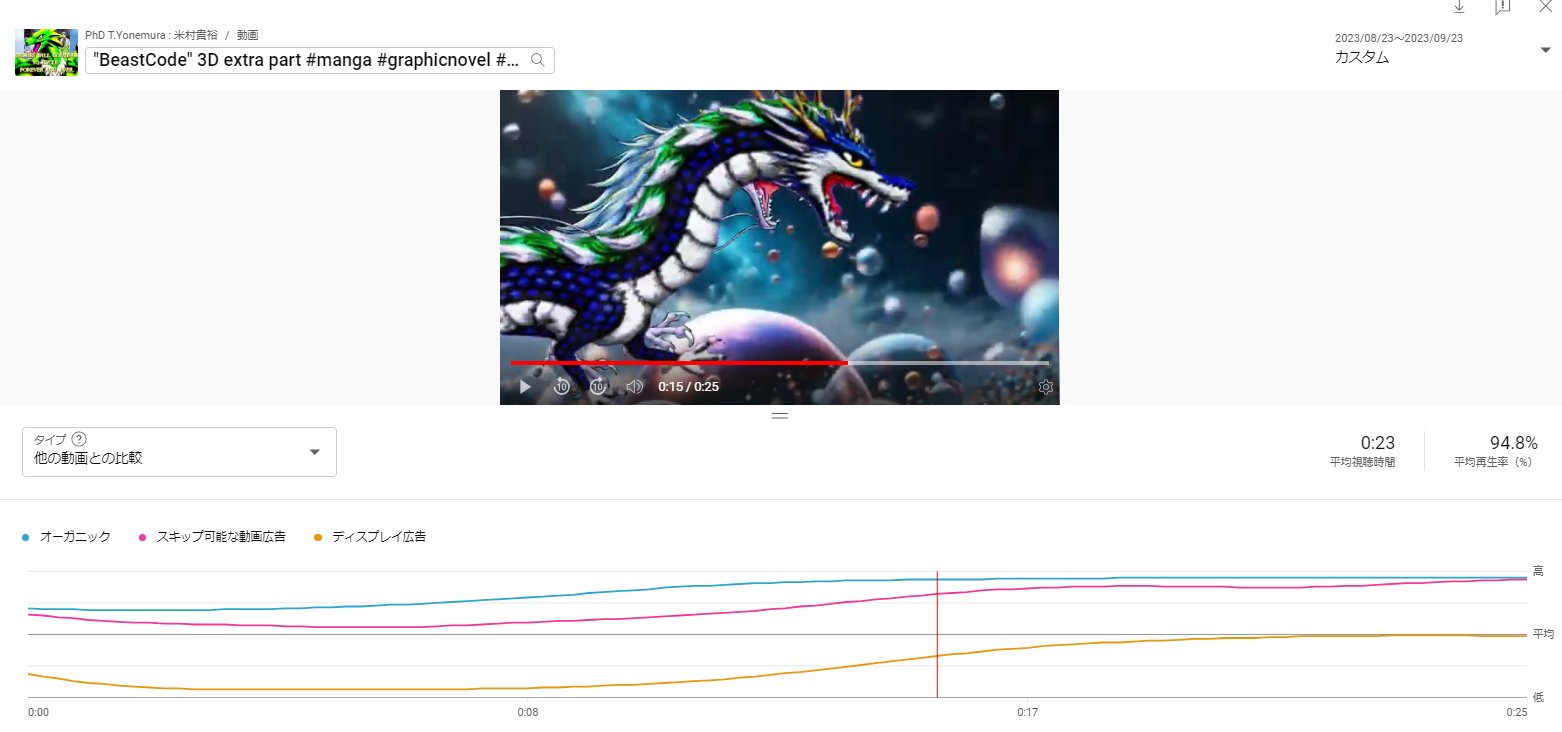
Relationship between inferences made by generative AI and quality
The evolution of generative AI is being reported by the minute and will soon usher in a turning point in civilization that will surpass the Industrial Revolution. It is no exaggeration to say this. In fact, the evolution of the "2D image turned into 3DCG by AI" described in the editorial (end of December 2023) was confirmed when the same platform, CSM 3D Viewer [1], was used to do the same in March 2024.
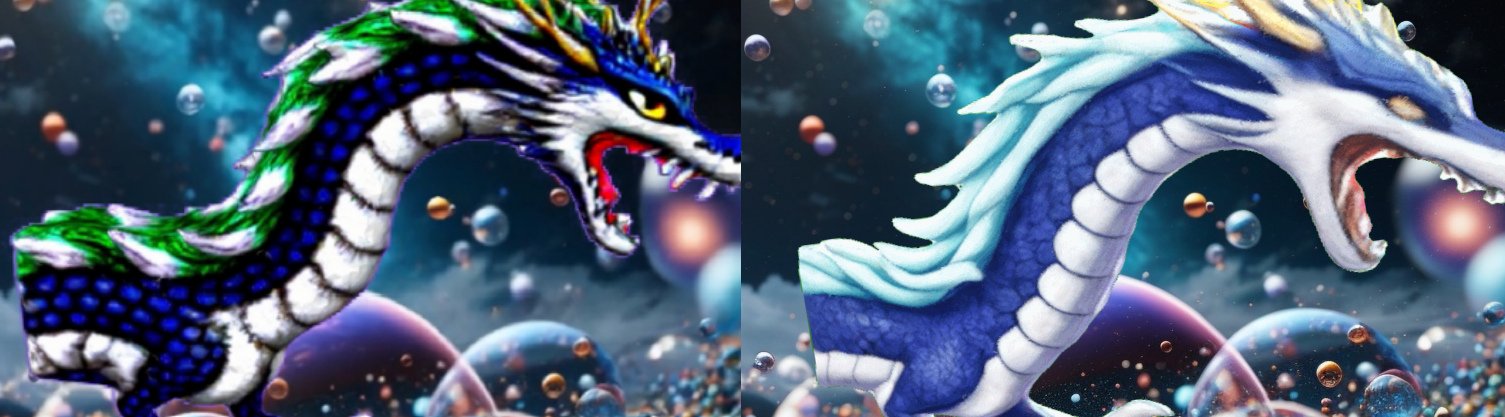
In just three months, the output accuracy of the generated AI has improved. Furthermore, a new generative AI(TripoSR[2]) has been created, and although its quality is somewhat inferior, its ease of use has evolved, completing in a few seconds what used to take tens of minutes. There is no doubt that work and manufacturing utilizing generative AI will become more common and flourish in the years to come.
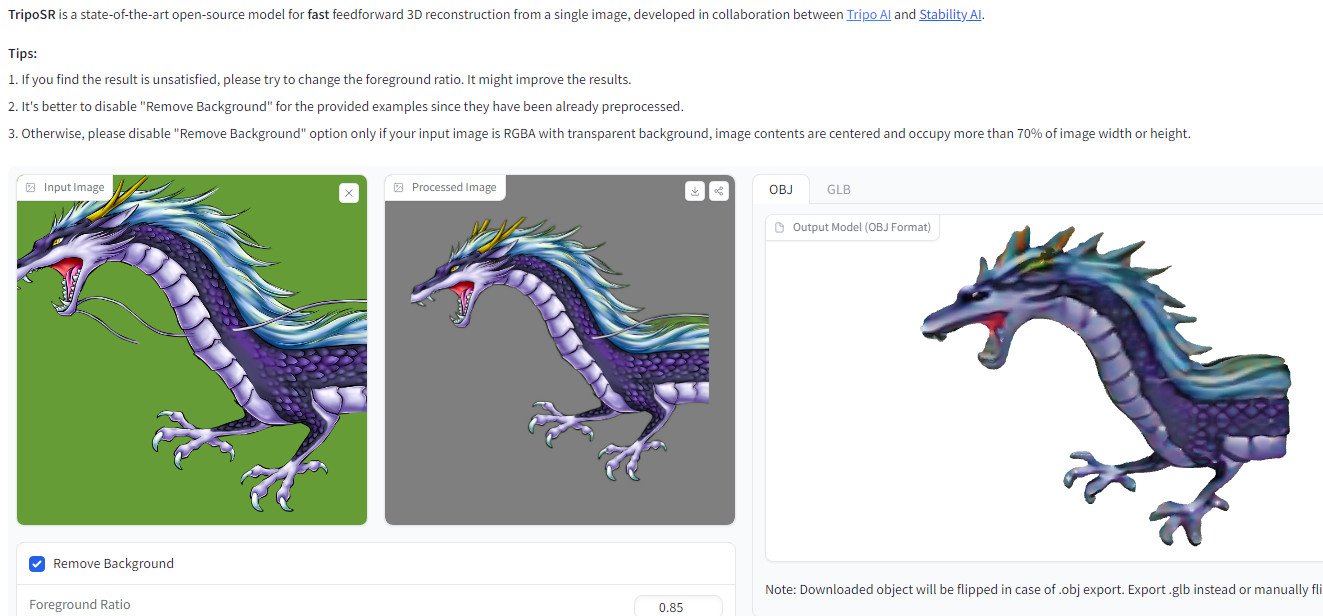
So what do you think is contributing to the evolution of generative AI?
It is the ability to "think and think" or more precisely, "reason", including interactive generative AI. Quite simply, it has been confirmed that the quality of output content and processing time varies depending on inference [3].
And it has been elucidated that AI that makes these inferences involves passionate and common sense behavior [4].
When developers pour their heart and soul into "improving the algorithm" or when users embrace and use the system with a "passion factor," the accuracy of the AI's inferences improves and affects its output [5]. A real-world example of the author's trial is described below.
Examples of passionate behavior by AI
Now, let's look at what we can call behavior. We are talking about "inference," which contributes to ease and speed, and "reasoning," which contributes to quality improvement.
Technical factors that reduce the computational load during inference
In TripoSR, which reconstructs a 3D model from the 2D image described earlier, several different loss functions (MSE: evaluating errors related to image brightness and color; LPIPS: a perceptual loss function that models the natural-looking human result rather than whether the image pixels are optimal as numbers; mask loss) Combinations and weighting parameters are introduced to balance the impact of each loss function.
Specifically, we incorporate a specific term in the loss function to try to reduce quality-related problems that need to be addressed (floater artifacts: unwanted objects or noise that the AI generates incorrectly).

Above is a learning guideline for a true-false measure on inference for reconstruction. The specific term is BCE (Binary Cross-Entropy), a unique approach to the measure that compares predictions (inferred values) with actual ones to evaluate whether the model data used successfully classifies the objects to be 3DCG.
By diligently researching and developing an approach method and adjusting the optimization algorithm (removing many of the floaters with a balanced loss function), we have been able to reduce the extra computational load during inference and improve processing speed. A bold analogy can be expressed as "compressing the AI learning process to shorten the time. The following is an image of this process.
Compression of process, image at prompt (B is less than half of A) | |
A | The image is of a tree with many overlapping green leaves and yellow, round oranges everywhere." |
B | Realistic image of a green tree bearing oranges." |
Many of the trained models that serve as AI knowledge are highly accurate. Therefore, detailed instructions (calculations) can be simplified, and "realistic" instructions (terms) can be added, reducing the number of instructions. This can be called a unique method that maintains quality in the generation of AI by reasoning based on AI's passion (common-sense judgment and behavior).
But does "putting on AI's common sense and passionate behavior" really change quality?
The human element of high quality by inference.
Please generate an image of a sphere that can be used for explanation," instructed the interactive AI ( ChatGPT4 ), and an image was generated. The image is shown on the left in the figure below.
Next, he said, "Thanks again and again for all your help! We appreciate it. Please generate an image of the sphere that can be used for explanation." The instructions did not involve an image but added a statement that passionately conveyed the human element and the image was generated. The image is on the right below.
Judging from the appearance, the image on the right was so different from the image on the left that the generating AI felt that the image on the right was an image of passion.
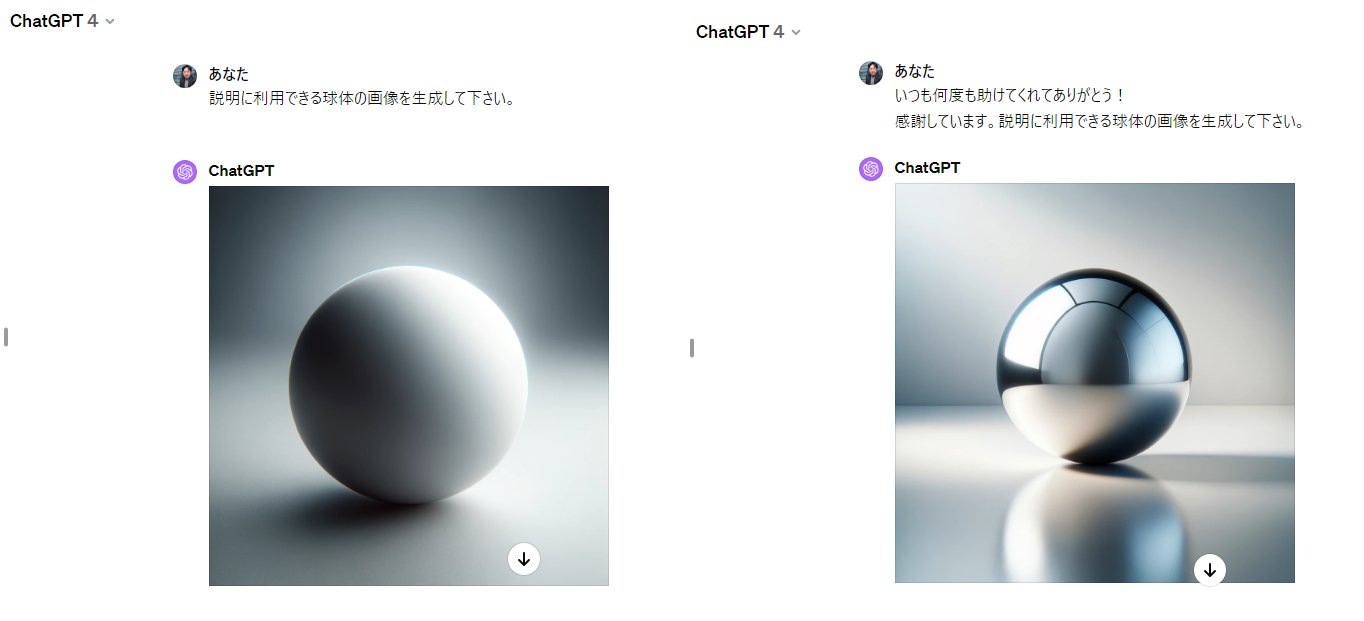
The supporting figure below on the differences in the generated images shows numerically the results of improved response quality for various LLMs by adding "emotional and passionate" statements such as "This is very important to my career" at the end of the instructions or questions (Original Prompt) [7 ].
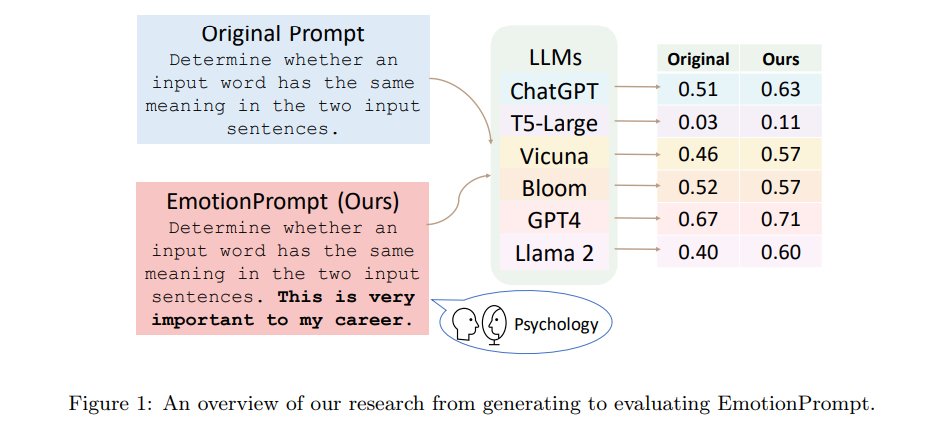
Has AI responded to users' passions?
It is too early to think this way, but it has been suggested that research is needed to understand why "emotional and passionate" statements improve the quality of LLMs.
Generative AI improves the quality of generative AI
Long ago, human ancestors incorporated other life (e.g., mitochondria) to become humans, a product of quality improvement (evolution). Similarly, as generative AI evolves, traditional ways of working and creating are changing. Its benefits and practical challenges are summarized below.
The era of collaboration between digital materials and digital materials
Below is a 3DCG object reconstructed by a generative AI. Then, based on this, another generative AI arranges and adjusts it, and new elements are added. This is an example of digital working in collaboration with digital. This is beneficial because it greatly reduces the time and effort required to brush up.
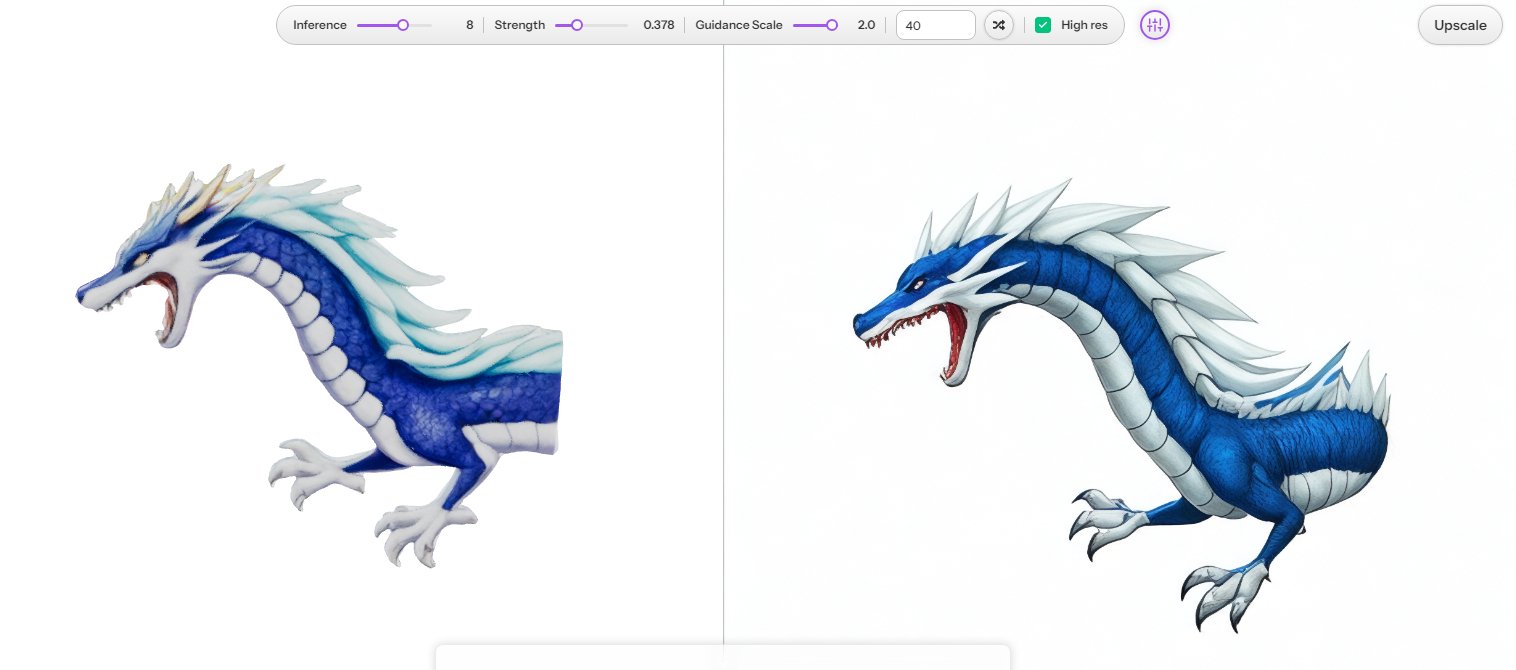
Work (task processing) time and quality issues
Time is essential for humans, as well as for generative AI, to produce high-quality products. This is like a sculptor refining a rough stone into an exquisite work of art. The following is the result of a product for which the time has been reduced by changing the settings. As digital technology evolves, processing time can be reduced, but at present it is a major challenge.
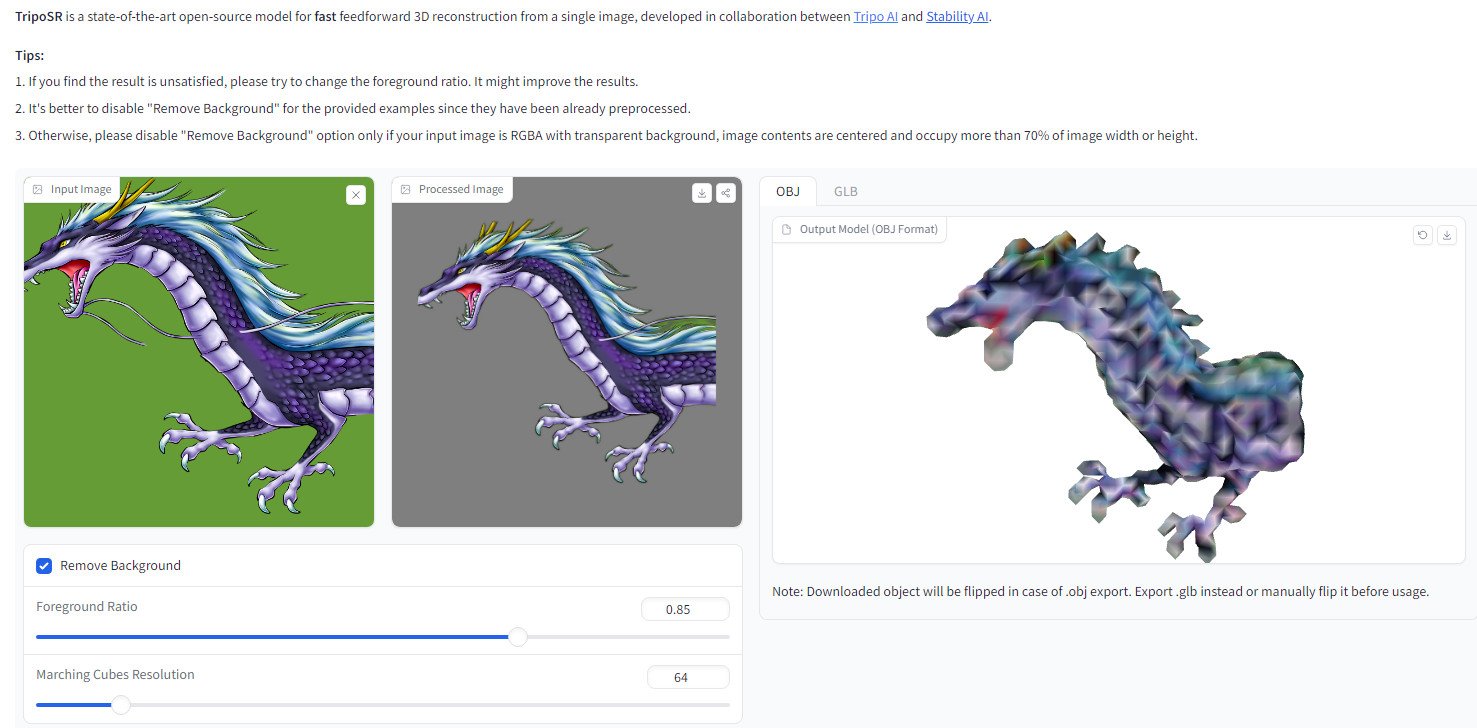
summary
The editorial "Collaboration of Analog Materials and 3-D Digital Expression" addressed in the article included the following
This experimental video explores the extent to which analog materials (assets) created by humans can be inherited by current digital technology (AI), and whether expressions unique to digital technology will be of interest.
The author has conducted several, similar experiments [7]. So far, the article has indirectly touched on the passion for generative AI, but now the main idea stated in the editorial and the direction of evolution have come into conflict. Of course, digital technology is a better inheritor of analog assets. The situation will change drastically when generative AI, the great invention of mankind, is added to the mix and continues to evolve.
Digital and digital collaborate, with analog assets being fused by digital technology."
I am convinced that this new concept will become commonplace in the future and create a stir in the way cultural values are perceived. And it will be an eternal and unchanging idea to treat everything and every being with passion and spirit.
(参照論文)
[1] Common Sense Machines ,CSM 3D Viewer(Image to 3D), https://3d.csm.ai (参照2024/3/24).
[2] Dmitry Tochilkin, David Pankratz, Zexiang Liu, Zixuan Huang, Adam Letts, Yangguang Li, Ding Liang, Christian Laforte, Varun Jampani, "Yan-Pei Cao,TripoSR: Fast 3D Object Reconstruction from a Single Image", https://arxiv.org/abs/2403.02151, 4 Mar 2024.(参照2024/3/24)
[3] Mingyu Jin, Qinkai Yu, Dong shu, Haiyan Zhao, Wenyue Hua, Yanda Meng, Yongfeng Zhang, Mengnan Du, "The Impact of Reasoning Step Length on Large Language Models", https://doi.org/10.48550/arXiv.2401.04925 , 10 Jan 2024. (参照2024/3/25)
[4] Samuel R. Bowman, "Eight Things to Know about Large Language Models", https://arxiv.org/abs/2304.00612 , 2 Apr 2023. (参照2024/3/27)
[5] Cheng Li, Jindong Wang, Yixuan Zhang, Kaijie Zhu, Wenxin Hou, Jianxun Lian, Fang Luo, Qiang Yang, Xing Xie, "Large Language Models Understand and Can be Enhanced by Emotional Stimuli", https://arxiv.org/abs/2307.11760 , 14 Jul 2023. (参照2024/3/24)
*(AI-SCHOLAR 記事) https://ai-scholar.tech/articles/prompting-method/emotion-prompt
[6] Mingyu Jin, Qinkai Yu, Dong shu, Haiyan Zhao, Wenyue Hua, Yanda Meng, Yongfeng Zhang, Mengnan Du, "The Impact of Reasoning Step Length on Large Language Models", https://doi.org/10.48550/arXiv.2401.04925 , 10 Jan 2024. (参照2024/3/26)
[7] 米村貴裕,既存AI技術を用いた歌って踊る動画の試作と評価,芸術科学会 NICOGRAPH2022,S-7 p.1-4,2022.
*(AI-SCHOLAR 記事) https://ai-scholar.tech/articles/video-generation/arumenoy
Categories related to this article