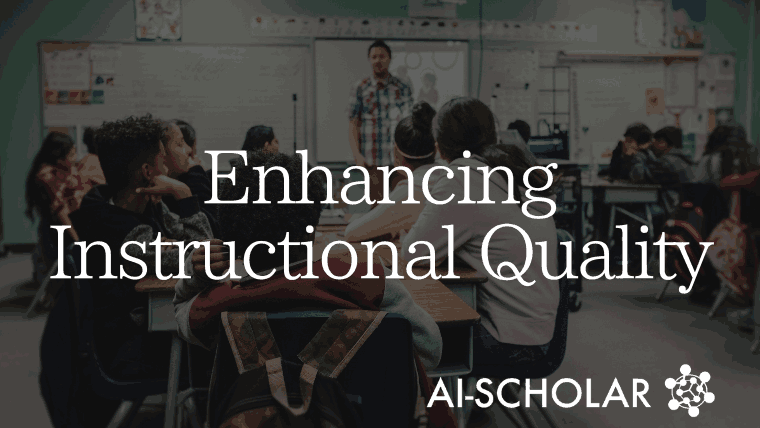
The Future Of AI In Education" Human-Centered Learning And Technological Foundations
3 main points
✔️ Explore ways to improve instruction by leveraging artificial intelligence and machine learning methods to gain insights from educational content and teacher and student interaction
✔️ Text Analysis in Education
✔️ Text analytics and generative AI in education can optimize personalized learning experiences and instruction
Enhancing Instructional Quality: Leveraging Computer-Assisted Textual Analysis to Generate In-Depth Insights from Educational Artifacts
written by Zewei Tian, Min Sun, Alex Liu, Shawon Sarkar, Jing Liu
(Submitted on 6 Mar 2024])
Comments: Published on arxiv.
Subjects: Artificial Intelligence (cs.AI); Computation and Language (cs.CL); Human-Computer Interaction (cs.HC)
code:![]()
The images used in this article are from the paper, the introductory slides, or were created based on them.
Summary
This paper focuses on the potential of computer-assisted text analytics to improve the quality of education. Specifically, it explores how artificial intelligence and machine learning methods can improve instruction by gaining insights from educational content and teacher/student interaction. Based on Richard Elmore's Instructional Core Framework, it is shown that AI/ML can bring significant benefits to teacher coaching, student support, and content development in educational settings.
It is also emphasized that AI/ML contributes to streamlining administrative tasks and providing individualized learning pathways, feedback to educators, and an understanding of instructional dynamics. Finally, it is advocated that a balanced approach is needed that aligns AI/ML technology with educational goals and considers ethical considerations, data quality, and integration of human expertise.
Introduction
This study focuses on the importance of improving instruction and advancing AI in education. The post-pandemic situation and advances in AI have created a need for personalized teaching strategies and new learning methods. However, traditional methods are limited in their ability to interpret complex educational data, and advances in AI and machine learning have enabled effective processing of these data and new approaches to education. In particular, advances in natural language processing (NLP) have played a major role in interpreting and processing linguistic data.
This study also explores the application and benefits of AI/ML in the field of education. It focuses on three key elements in improving instruction in education: teachers, students, and content, each of which simultaneously uses and generates textual data. The usefulness and complexity of AI/ML in textual analysis of educational data and future research directions are discussed. Such research not only contributes to the improvement of education but also provides directions for future instructional improvements.
Instructional Core Framework
The Instructional Core Framework is used to analyze educational data predict future directions and provide insights to support educational practice. The framework transforms instructional approaches to teaching and emphasizes the interaction of teachers, students, and content. In order to improve the quality of instruction, the interaction between teacher, student, and content must be analyzed for improvement strategies. In addition, AI/ML facilitates the analysis of instructional data, enabling educators and administrators to make more effective decisions. Integrating AI/ML at the core of instruction will foster innovation in education and improve the learning experience for students.
Objective
While traditional feedback mechanisms are sporadic and evaluative, technology-based approaches are more comprehensive and effective. In particular, Natural Language Processing (NLP) provides educators with deeper insights by analyzing classroom text data to evaluate teaching practices. Educators can thereby gain a better understanding of their own strengths and room for improvement. In this context, text analytics in education is becoming increasingly important as research is being conducted to demonstrate the effectiveness of AI-driven tools.
Proposed Method
It suggests ways to use AI and machine learning (ML) techniques to enhance student support and assessment. Specifically, three main applications are addressed
1. personalized learning support: AI and ML provide adaptive material to students using models tailored to specific subject matter.
2. coursework analysis and automated grading: To reduce the effort of teachers to grade and provide efficient feedback, AI and ML provide coursework analysis and automated grading.
3. Intelligent tutoring: Using student log data, AI and ML provide personalized instruction by recommending appropriate feedback messages.
These applications provide educators with the flexibility to improve the quality of their feedback and meet the individual needs of their learners.
Data science technology and artificial intelligence also provide innovative opportunities for educational research. With a focus on text data analysis and generation, natural language processing and generative AI can be used by educators to effectively develop instructional materials and assess students. Analysis of textbooks and online educational resources can provide new insights and contribute to the advancement of educational research.
Conclusion
The study suggested that AI and machine learning techniques could be important tools for educators to improve the quality and effectiveness of instruction. In the field of education, the introduction of AI-based text analysis and generative AI will enable personalized learning experiences and optimized instruction. However, the implementation of these technologies comes with several challenges. These include ethical considerations, privacy protection, and data quality.
Therefore, the use of AI in education requires careful consideration and appropriate guidelines. In addition, it is also important to discuss the potential social impacts and educational equity that may result from the introduction of AI. By addressing these issues seriously, AI and machine learning technologies have the potential to bring about real innovation in the field of education and provide quality education to more learners. Ultimately, it is important to keep in mind that AI and machine learning can be key to shaping the future of education, but its realization will require educators, researchers, and technologists to work together to promote ethical and effective implementation.
There is also an emphasis on human-centered learning of AI in education. This approach aims to use AI to promote human creativity and foster skills such as critical thinking and collaboration. Future research should investigate how AI can enhance the learning experience beyond the traditional classroom environment. It also emphasizes the importance of promoting AI literacy and the role of teachers.
The issue of resources and data needed to train AI/ML models is then addressed. Without sufficient training data, AI progress may be limited. It is also important to assess the balance between model size and training resources to lay the groundwork for future development.
Categories related to this article