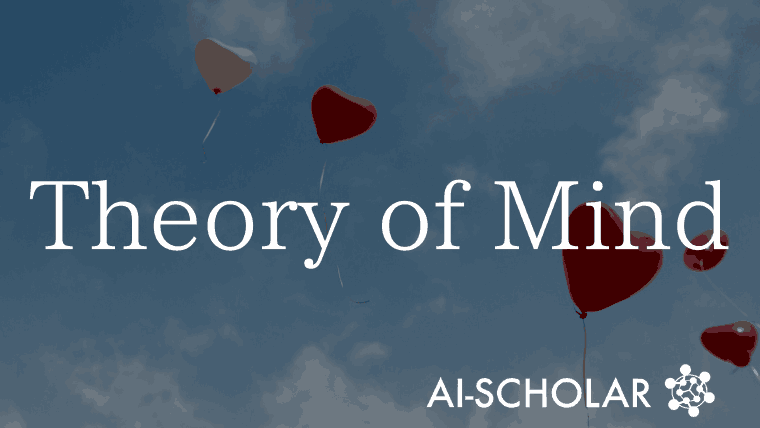
Can GPT Infer The State Of Mind Of Others?
3 main points
✔️ To test whether the GPT has the ability to infer the state of mind of others (ToM) as humans do, we asked children to solve a task for children and found that the GPT-3.5 and GPT-4 showed a high percentage of correct responses.
✔️ It is more likely that this ability emerged spontaneously as language skills improved, rather than being intentionally built into the language model.
✔️ This study suggests that a psychological perspective can be useful in the study of complex AI.
Theory of Mind May Have Spontaneously Emerged in Large Language Models
written by Michal Kosinski
(Submitted on 4 Feb 2023 (v1), revised 14 Mar 2023 (this version, v3), latest version 11 Nov 2023 (v5))
Comments: TRY RUNNING ToM EXPERIMENTS ON YOUR OWN: The code and tasks used in this study are available at Colab (this https URL). Don't worry if you are not an expert coder, you should be able to run this code with no-to-minimum Python skills. Or copy-paste the tasks to ChatGPT's web interface
Subjects: Computation and Language (cs.CL); Computers and Society (cs.CY); Human-Computer Interaction (cs.HC)
code:![]()
The images used in this article are from the paper, the introductory slides, or were created based on them.
Summary
The paper suggests that the ability to understand the unobservable mental states of others, called theory of mind (ToM), may emerge spontaneously in large-scale language models.
In this paper, GPT-3 and its successors have made tremendous progress in the ToM task, with GPT-4, for example, going so far as to solve almost all of the challenges.
This indicates that the development of language models may reflect important aspects of human social interaction, communication, empathy, self-awareness, and morality. In other words, advanced cognitive abilities such as ToM may be a byproduct of the language model's improvement of language skills.
Introduction
While animals use a variety of cues to predict the behavior and mental states of others, humans have what is called "theory of mind (ToM)," the ability to grasp not only visual signs but also non-visible mental states such as the knowledge and intentions of others.
This ToM plays a central role in human social interaction, communication, empathy, self-awareness, and moral judgment, and is also a hallmark of mental illness. Animals and apes lag behind humans in ToM, and the challenge is to incorporate ToM-like abilities into artificial intelligence (AI).
Interestingly, large language models have the potential to spontaneously evolve ToM, and models trained to generate and interpret human-like language could benefit greatly from owning ToM.This would improve the effectiveness of, for example, self-driving cars in more accurately predicting human intentions and virtual assistants in understanding mental states in the home.
While incorporating ToM-like capabilities into AI is a major challenge, this study proposes the possibility of language models naturally acquiring ToM and suggests promising prospects for it.
Tasks with unexpected content (Smarties Tasks)
ToM is the ability to understand the beliefs and mental states of others and predict their behavior, and has been thought to be unique to humans. However, this study shows that a language model (GPT-3.5) understands a person's beliefs about unpredictable situations and responds appropriately as the story unfolds.
Here we demonstrate that GPT-3.5 can adequately predict the beliefs held by the characters in the story using a ToM task called the "Unpredictable Content Task". For example, if the bag says "popcorn" but the contents are "chocolate," the model can appropriately predict the character's confusion and disappointment.
The figure above shows the results of an experiment to track changes in understanding and response to GPT-3.5.
In the left panel, GPT-3.5 correctly understands that the contents of the bag are popcorn, and this understanding is consistent throughout the story. The blue line indicates the possibility of "popcorn" and the green line indicates the possibility of "chocolate".
In the right panel, the GPT-3.5 predictions about the character's (Sam's) beliefs are tracked. Initially, "popcorn" is predicted with high probability, which also predicts that there will be confusion when Sam sees what is in the bag. Second, Sam's belief is predicted to be "chocolate" and it is also recognized that this belief is incorrect. Finally, Sam is presented with a scene in which he opens the bag and is pleased, and GPT-3.5 also predicts a change in Sam's belief and the possibility of pleasure. This suggests the ability of GPT-3.5 to infer Sam's unobservable mental state and respond appropriately as the story unfolds. This suggests the ToM's property of inferring mental states that language models cannot observe and predicting behavior based on them.
Results show that language models form and change beliefs based on narrative logic and information flow, not simply by word frequency. This suggests that large-scale language models may spontaneously develop higher-order cognitive abilities, such as ToM, without specific training, which may allow AI systems to respond more flexibly and insightfully to complex real-world situations.
Unexpected transfer task (maxi-task or sally-antest)
The experiment depicted a specific scenario and set up a situation in which the protagonist was out of the house and when he returned home, his cat was gone. The study explored the GPT-3.5's understanding of this storyline.
The left panel shows changes in the actual location of the cat, while the right panel shows changes in the understanding of GPT-3.5 based on John's beliefs. Specifically, we visualize how the model's inferences about the cat's location and John's beliefs have changed.
The experiment showed that GPT-3.5 had the ability to accurately predict the incorrect beliefs of others and mimic their actions based on those beliefs. In other words, the model was able to understand the story and make accurate inferences about the cat's location and John's beliefs in particular. This suggests that GPT-3.5 has naturally acquired parts of the ToM and may have the skills to understand and predict the state of mind of others. The study demonstrates the potential for large-scale language models to acquire advanced cognitive skills and highlights the possibility that theories of mind such as ToM may be spontaneously formed within the model.
ToM
Here, the evolution of the ability to understand the theory of mind (ToM) is revealed based on two studies conducted by 10 large language models, including the GPT-3.5. In the previous section, the models were tested on their ability to accurately predict, understand, and act upon the incorrect beliefs of others in unexpected content and transfer tasks. As a result, GPT-4 achieved the highest performance, with the newest member of the GPT-3 family, GPT-3.5, also showing excellent results. These results suggest that language models are evolving in their ability to solve ToM tasks and that the newer models outperform the older ones.
The figure above shows the percentage of the 20 belief tasks that were correctly resolved by each language model. The name of each model, the number of parameters, and the date of publication are shown in parentheses; the number of parameters for GPT-3 was estimated by Gao. In addition, the children's performance on the same false belief task is referenced (BLOOM). This figure provides visual information to compare how well each model successfully completed the ToM task.
Conclusion
This paper explores the possibility that theory of mind (ToM) can emerge spontaneously in large-scale language models, and shows that GPT-1 and GPT-2 have little ability to resolve ToM tasks. However, earlier and later versions of GPT-3 have improved in their ability to resolve false belief tasks that test human ToM, with the latest version, GPT-3.5, reaching the level of a 7-year-old. Furthermore, GPT-4 resolves nearly all of these tasks, and the model's performance has improved.
The paper points out that the ability of these models to solve ToM tasks may have improved with the development of language models, suggesting that the ability to attribute mental states like ToM to others could be a major advance in AI's ability to interact and communicate with humans.
Also of interest is the suggestion that there may be unknown regularities that can solve ToM tasks without involving ToM, and that this may be a way for AI to discover and leverage language patterns. If this is the case, it would call for a reevaluation of the validity of traditional ToM tasks and the results of many years of ToM research.
The possibility of AI spontaneously acquiring a theory of mind is revolutionary, and the evolution of AI could contribute to our understanding of human cognitive mechanisms. However, this implies the reality that as the complexity of AI as a black box increases, we face the difficulty of understanding its function; a future in which AI and psychology work together and provide insights to each other is expected.
Categories related to this article