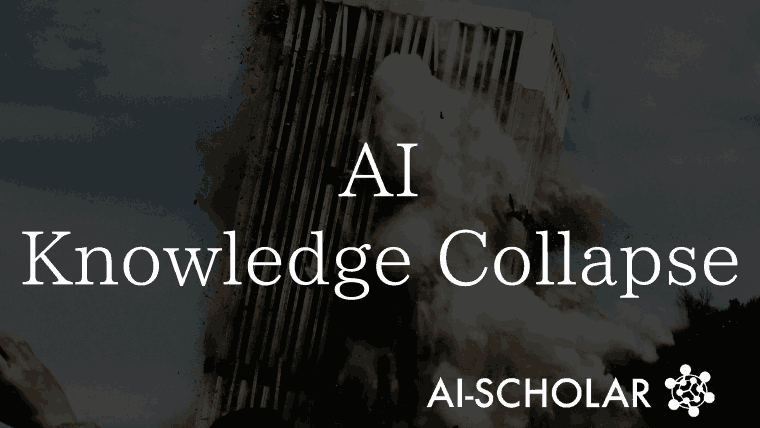
Danger Of "Collapse Of Knowledge" Caused By Evolution Of AI Technology
3 main points
✔️ The development of AI technology may lead to a "knowledge collapse" in which the human knowledge system becomes biased toward middle-of-the-road information as content generated by large language models and other sources becomes the primary source of human information.
✔️ Simulation results show that overreliance on AI-generated information tends to make public knowledge deviate from the truth and neglect long-tailed knowledge.
✔️ If humans can strategically select sources of information and recognize the value of knowledge in the tails, they may be able to prevent knowledge collapse.
AI and the Problem of Knowledge Collapse
written by Andrew J. Peterson
(Submitted on 4 Apr 2024)
Comments:16 pages, 7 figures
Subjects: Artificial Intelligence (cs.AI); Computers and Society (cs.CY)
code:![]()
![]()
The images used in this article are from the paper, the introductory slides, or were created based on them.
Summary
This paper analyzes the potential for the development of artificial intelligence (AI) technology to narrow the human knowledge base.
According to the authors, if AI technologies such as Large Language Models (LLMs) advance rapidly and AI-generated content becomes a large part of the information that humans come into contact with, the long tail of knowledge - that is, minority perspectives and specialized knowledge - may be neglected and lost. This is defined as the "collapse of knowledge.
In the past, new information technologies have had a significant impact on the generation and transmission of knowledge, with the rise of the written word undermining the practice of text memorization and review, and the rise of Internet search algorithms causing extreme polarization in user attitudes and politics. This paper examines the possibility that similar problems may be caused by the rise of AI technologies.
As a specific model, we set up a situation in which an individual is considering whether to choose a traditional or AI-assisted learning method. The individual's choice is based on the gain gained by obtaining a sample from the true knowledge distribution. The public knowledge distribution, on the other hand, is an aggregate of the samples obtained by the individual.
The simulation analysis showed that as humans become overly dependent on AI-generated content, public knowledge will diverge significantly from the truth and converge on a biased and narrow perspective. However, if humans can strategically select information sources and recognize that knowledge in the tail has value, this knowledge collapse can be prevented.
The paper therefore argues that measures are needed to mitigate the impact of AI technologies, such as curbing the growing use of LLM and ensuring access to a variety of information sources. The paper also discusses a detailed analysis of the process of structuring knowledge through AI-human interaction and the importance of consideration in the use of AI in educational settings.
In other words, this paper is a significant study that theoretically and empirically analyzes the risk of shrinking the human knowledge base associated with AI development and proposes measures to avoid it.
Related Research
In examining the impact of AI technology, the paper discusses the following topics as relevant research to date
First, there is the issue of "filter bubbles" or "echo chambers" in social media. This is the extreme polarization of opinion that occurs when users are exposed only to information that aligns with their own beliefs and preferences, and lose access to a wide variety of information.
Next, he mentions a phenomenon called the information cascade model, in which private information of individuals is not efficiently aggregated, creating herd behavior.
In addition, based on the findings of the network analysis, the mechanisms of distortion of information distribution in social media are also discussed.
On the other hand, a problem specific to large language models (LLMs), known as "model decay," or the degradation of output due to AI training on self-generated data, is also addressed.
Based on these previous studies, it can be understood that this paper is attempting to analyze the possibility that human reliance on AI-generated information distorts the entire knowledge system.
Proposed Method
The model decides between the information an individual gets from the true knowledge distribution and the information he or she gets from AI-generated information with a discount rate.
[Figure 1] Individual Information Selection Process
Individuals gain by sampling from the true knowledge distribution, but AI-generated information is cheaper, so individuals have to make a choice in the tradeoff.
The information obtained is aggregated into a public knowledge distribution, but knowledge decay may occur because individual strategic actions do not necessarily lead to public knowledge improvement.
[Figure 2] Formation of public knowledge distribution
Information aggregated by individual choice forms the public knowledge distribution, but the potential for deviation from the true distribution is problematic.The possibility of accelerated knowledge decay is also considered, as new generations learn to consider the knowledge distribution of the previous generation as representative.
Experiment
The paper uses the proposed simulation model to analyze how the degree of utilization of AI-generated information affects knowledge decay.
First, Figure 3 shows the relationship between the discount rate for using AI-generated information and the deviation between the public knowledge distribution and the true distribution (Hellinger distance).
[Figure 3] Discount Rate and Degree of Knowledge Decay
When AI-generated information was available at a low cost (high discount rate), the public knowledge distribution deviated significantly from the true distribution. On the other hand, when AI-generated information was costly to use (low discount rates), knowledge decay was suppressed.
Figure 4 then shows the individual's learning rate and its impact on knowledge decay.
[Figure 4] Learning rate and degree of knowledge decay
It has been shown that knowledge decay is more likely to progress if individuals learn from previous results at a slower rate. However, a faster rate of learning suggests that knowledge decay may be reduced even when the discount rate is high.
Based on these results, the paper argues that if individuals can strategically select information sources and recognize the value of tail knowledge, they can prevent knowledge decay due to overreliance on AI-generated information.
Conclusion
In conclusion, the paper points to the possibility of a "knowledge collapse" in which the human knowledge system becomes biased toward middle-of-the-road information if the majority of data that humans come into contact with is replaced by AI-generated content due to the development of AI technology.
Simulation results indicate that overreliance on AI-generated information leads to a significant deviation of public knowledge from the truth and neglect of long-tailed knowledge. On the other hand, if humans can strategically select information sources and recognize the value of tail knowledge, this problem could be mitigated, according to the study.
The paper therefore argues that measures are needed to mitigate the impact of AI technologies, such as curbing the growing use of LLMs and ensuring access to diverse sources of information.
The report also discusses future prospects, including the need for more detailed analysis of the process of structuring knowledge through AI-human interaction and the importance of consideration in the use of AI in education.
In other words, this paper is a significant study that theoretically and empirically analyzes the risk of shrinking the human knowledge base associated with AI development and proposes measures to avoid it.
Categories related to this article