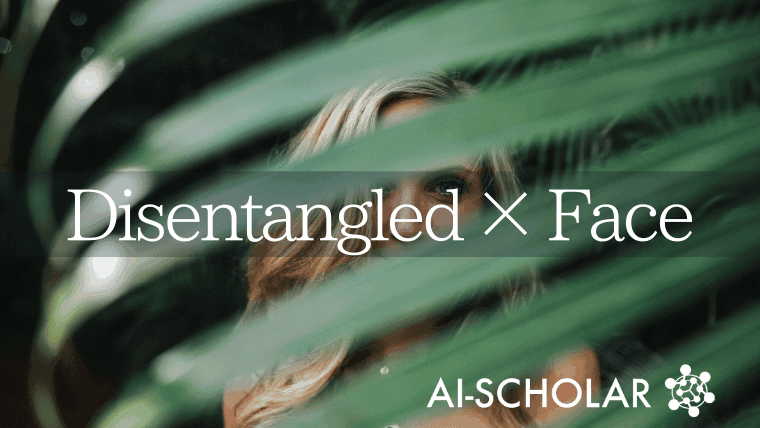
Face Spoofing Detection Using Disentangled Representation Learning
3 main points
✔️ Introduce Disentangled Representation Learning to the Face Anti-spoofing task to decompose latent features into Liveness Feature and Content Feature
✔️ Regularize the Liveness feature space by combining low-level texture information and high-level Depth information
✔️ Conducted experiments with representative benchmarks to validate the effectiveness compared to SOTA
Face Anti-Spoofing Via Disentangled Representation Learning
written by Ke-Yue Zhang, Taiping Yao, Jian Zhang, Ying Tai, Shouhong Ding, Jilin Li, Feiyue Huang, Haichuan Song, Lizhuang Ma
(Submitted on 19 Aug 2020)
Comments: Accepted by ECCV2020
Subjects: Computer Vision and Pattern Recognition (cs.CV)![]()
Outline
As the accuracy of image recognition has improved, face recognition technology has become available with higher performance than that of humans. Nowadays, it is widely used in familiar places such as smart devices, access control, and security.
However, there is a concern about the risk of impersonating others because of the familiarity and easy availability of human face images. Impersonation of others is also called Presentation Attack (PA), and many methods have been reported, such as printed images, videos using electronic devices, 3D masks, and makeup. In order to provide a safe and secure face recognition system, it is essential to have a robust face recognition technology against spoofing.
Since typical PAs contain traces specific to video images, texture analysis-based methods have been proposed. As in other fields, there are handcraft-based and CNN-based methods, and handcraft-based methods include Local Binary Pattern (LBP)/ Histogram of Oriented Gradients (HOG)/ Scale Invariant Feature Transform (SIFT), etc., are used to detect spoofing by focusing on motions such as lip movements and blinking in videos. However, these methods cannot detect PA played on electronic devices.
In recent years, with the advancements in deep learning, convolutional neural network (CNN) based methods have made significant progress in face spoofing detection. In this method, the task of spoofing detection is treated as binary classification using Softmax. However, it is difficult to learn intrinsic spoofing traces, overfitting to specific datasets, and mostly lacking in generalization performance.
Recently, some techniques have been reported to detect spoofing with higher accuracy by using supplementary information such as Depth Map and rPPG, which are useful for spoofing detection. However, this requires predefining features that are useful for spoofing, and it is not realistic to identify all such information.
In face spoofing detection, it is not how to precisely predefine the spoofing pattern, but how to represent the spoofing pattern from high dimensional features that is important.
Here, one of the possible solutions is Disentangled Representation Learning (DRL). This DRL is a method that assumes that high dimensional information can be explained by latent representation variables that are substantially lower-dimensional and meaningful.
In face spoofing detection, the pattern of spoofing can be viewed as one of the attributes of a face, not just a particular irrelevant noise type or combination of noise types. Therefore, we believe that by directly targeting the extraction of Liveness features from all variations of face images, we can learn features that are useful for spoofing detection.
Based on this idea, this paper proposes a method for face spoofing detection using Disentangled Representation Learning, which separates Latent Representation, as shown in the figure below. We assume that the Latent Space of a face image can be decomposed into two spaces, the Liveness Space and the Content Space. Here, Liveness Feature is a feature related to Liveness, and Content Feature is a feature related to ID and lighting conditions.
It extracts Liveness features that represent the characteristics of spoofing and uses more intrinsic information for spoofing detection, which is not influenced by other noise information, and thus can achieve a model with better generalization performance than conventional models.
To read more,
Please register with AI-SCHOLAR.
ORCategories related to this article