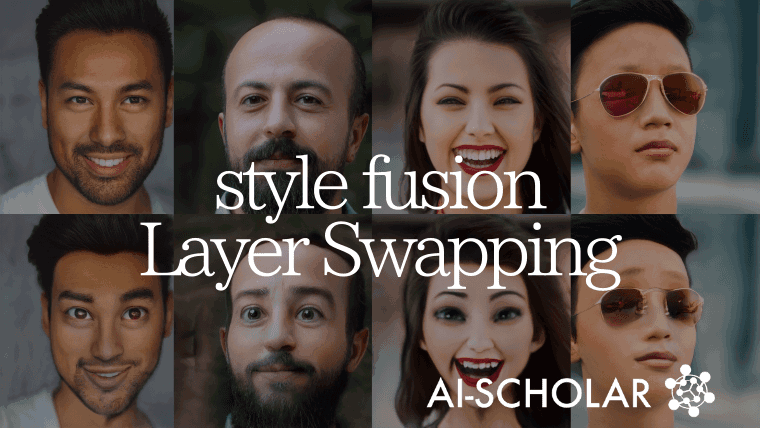
The Easiest Way To Convert Image Styles!
3 main points
✔️ A simple style fusion technique that only replaces the layers of GAN
✔️ Applying the theory of StyleGAN
✔️ Can be developed into a complex fusion
Resolution Dependent GAN Interpolation for Controllable Image Synthesis Between Domains
written by Justin N. M. Pinkney, Doron Adler
(Submitted on 11 Oct 2020 (v1), last revised 20 Oct 2020 (this version, v2))
Comments: Accepted at NeurIPS 2020 Workshop
Subjects: Computer Vision and Pattern Recognition (cs.CV); Image and Video Processing (eess.IV)
Paper Official Code Demo
Introduction
As we all know, GANs can generate realistic images from training data. However, it is inherently difficult to use GANs for completely creative and creative purposes, such as being a painter, because they essentially learn the distribution of training data.
So there are some areas of research that aim to control the ability to generate artistic images. In this article, we're going to show you a technique that allows you to generate a whole new image and be a bit more creative while still having some control over the output.
By interpolating between parameters from different models and relying on a specific layer resolution, it is possible to fuse features from different generators. Another feature of the technique presented here is that it achieves this with one of the simplest techniques in the current control methods.
technique
As an overview, there are only three studies to be done.
- Training GANs on-base datasets
- Transfer training of the GANs learned in 1 with the transfer data set
- Layer swapping of GANs created in 1.2 (Layer Swapping)
To read more,
Please register with AI-SCHOLAR.
ORCategories related to this article