Move The GAN With Your Phone! Combination Of Compression Techniques To Reduce Weight, 'GAN Slimming'
3 main points
✔️ Proposed an optimal framework combining compression techniques for machine learning models
✔️ Applying compression to the GAN model of image-to-image translation
✔️ CartoonGAN compresses models to 1/47th of their original size while maintaining image quality.
GAN Slimming: All-in-One GAN Compression by A Unified Optimization Framework
written by Haotao Wang, Shupeng Gui, Haichuan Yang, Ji Liu, Zhangyang Wang
(Submitted on 25 Aug 2020)
Comments: Accepted at ECCV 2020 spotlight.
Subjects: Machine Learning (cs.LG); Computer Vision and Pattern Recognition (cs.CV); Image and Video Processing (eess.IV); Machine Learning (stat.ML)
Overview
As the number of ways to utilize machine learning models has increased in recent years, there is an increasing demand for deploying them on smartphones, microcontrollers, and other lightweight devices. In the field of image transformation, in particular, there is a lot of talk about apps that allow users to pseudo-change ages, exchange multiple faces, and apply makeup on their phones.
An alternative model for image transformation is Generative Adversarial Networks (GANs), which are famous for their ability to generate high-quality false images. However, at the same time, GANs are known to be very parameter-rich and computationally difficult to learn. (There are other problems as well, such as this one ) As an example given in this paper, it is known that it costs 56 GFLOPs to train the SoTA model of image transformation, CartoonGAN. ( See here for an explanation and comparison of FLOPs.)
Therefore, this study proposes GAN Slimming (henceforth GS), a method for compressing GAN models using all the methods used to compress the size of common machine learning models, such as distillation, pruning, and quantization.
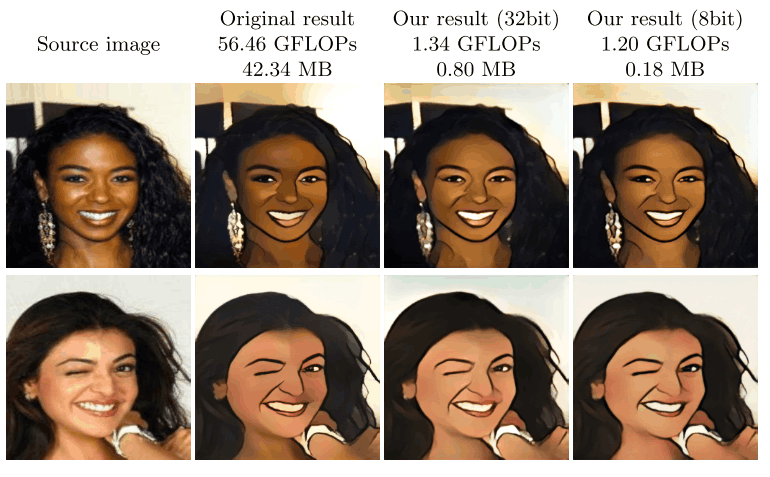
To read more,
Please register with AI-SCHOLAR.
ORCategories related to this article