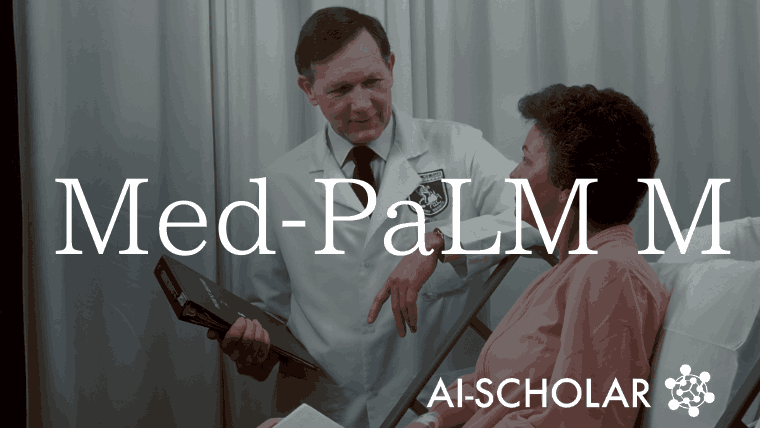
Applying The Large-scale Language Model PaLM! What Is Med-PaLM M, A General-purpose AI Developed By Google To Assist Doctors In Diagnosis?
3 main points
✔️ Introducing MultiMedBench, a new benchmark that includes a variety of medical data, including medical images and genetic information.
✔️ Introduces Med-PaLM M, the world's first general-purpose medical AI model that can handle multiple tasks in a single model.
✔️ Some tasks were shown to reach clinically acceptable levels.
Towards Generalist Biomedical AI
written by Tao Tu, Shekoofeh Azizi, Danny Driess, Mike Schaekermann, Mohamed Amin, Pi-Chuan Chang, Andrew Carroll, Chuck Lau, Ryutaro Tanno, Ira Ktena, Basil Mustafa, Aakanksha Chowdhery, Yun Liu, Simon Kornblith, David Fleet, Philip Mansfield, Sushant Prakash, Renee Wong, Sunny Virmani, Christopher Semturs, S Sara Mahdavi, Bradley Green, Ewa Dominowska, Blaise Aguera y Arcas, Joelle Barral, Dale Webster, Greg S. Corrado, Yossi Matias, Karan Singhal, Pete Florence, Alan Karthikesalingam, Vivek Natarajan
(Submitted on 26 Jul 2023)
Subjects: Computation and Language (cs.CL); Computer Vision and Pattern Recognition (cs.CV)
code:![]()
The images used in this article are from the paper, the introductory slides, or were created based on them.
First of all
The multimodal nature of the medical field, which handles a wide variety of information, requires AI models that can flexibly process a wide range of data formats, including text, images, and genomic information.
The paper presented here develops a versatile AI model, Med-PaLM Multimodal ("Med-PaLM M"), which can interpret multiple types of medical data, including language, medical images, and genetic information, and perform many different tasks with a single model. This is the first example of a single AI model that can handle a wide variety of medical data and shows better results than task-specific models. We have achieved these results without any task-specific adjustments.
To develop Med-PaLM M, we are also building a benchmark called MultiMedBench, which includes 14 diverse tasks.
Med-PaLM M achieves state-of-the-art performance on all MultiMedBench tasks, often significantly outperforming expert models. The MultiMedBench is also an open-source benchmark for evaluating how accurately AI can understand and adequately process complex real-world medical data, and provides a useful tool for building and evaluating the performance of versatile AI systems such as Med-PaLM M Med-PaLM M
What is "MultiMedBench"?
The "MultiMedBench" is a benchmark built to develop and evaluate the Med-PaLM M and contains 12 different datasets and 14 different tasks. The table and figure below provide an overview of the datasets and tasks. The benchmarks contain a total of over 1 million samples.
What is "Med-PaLM M"?
Med-PaLM M is a medical field-tuned version of the existing PaLM-E model, built on the architecture and model parameters of PaLM-ViT.
PaLM is a large-scale language model, trained on a large amount of data (780 billion tokens), including Internet web pages, Wikipedia, source code, social networking posts, news, and books of several sizes (8 billion, 62 billion, and 54 billion parameters). It has shown performance that exceeds average human performance on complex inference tasks. ViT is also an image recognition model called Vision Transformer. Two ViTs with 4 and 22 billion parameters are used in this paper. They are trained on about 4 billion images.
PaLM-E combines this PaLM with ViT and is built as a multimodal language model that can also understand text, images and data from sensors, and this model is the basis for Med-PaLM M. This paper utilizes three combined PaLM and ViT models (12B, 84B, and 562B) with different model sizes.
Med-PaLM M is built by fine tuning this PaLM-E with MultiMedBench. 3 models (12B, 84B, 562B) with different model sizes are also built for Med-PaLM M. Med-PaLM M is dedicated to the medical field and can handle tasks such as medical Med-PaLM M is specialized for the medical field and can handle tasks such as answering medical-related questions, generating radiology reports, image classification, and identifying genetic mutations.
Benchmark
This paper compares Med-PaLM M to two baselines. One is a task-specific model for each task included in MultiMedBench that achieves SOTA, and the other is PaLM-E (84B), which is not fine-tuned to the medical field. Note that PaLM-E uses a model size of 84B instead of 562B due to computational resource constraints.
The results are shown in the table below. As can be seen from the table (in bold), Med-PaLM M outperforms existing SOTA on many of the tasks included in MultiMedBench. It achieves SOTA on multiple tasks using the same model weights without task-specific fine tuning.
We also see that Med-PaLM M performs better on all 14 tasks when compared to PaLM-E (84B), which is not fine-tuned to the medical field. The significant improvement in most of the tasks shows that Med-PaLM M is useful as a versatile AI model.
In addition, the paper examines its applicability (performance against zero-shot) to new, unlearned medical tasks and concepts.
Using the dataset Montgomery County (MC), we evaluated the performance against zero shots on the task of detecting TB from chest X-ray images, and the results are shown in the table below; the performance is competitive with the SOTA model.
In addition, Med-PaLM M has the ability to analyze chest X-ray images and report whether there are any abnormalities there, such as tuberculosis. Therefore, radiologists are checking for oversights and errors in the reports generated by the artificial intelligence; to further understand the clinical applications of Med-PaLM M, radiologist evaluations of chest x-ray reports (and human reference values) generated by Med-PaLM M are being conducted.
We measured the number of oversights and errors in the reports generated by three Med-PaLM M's of different model sizes (12B, 84B, and 562B) and found that, on average, the 12B and 84B models missed the fewest oversights, followed by the 562B model missing slightly more The 84B model was found to have the lowest number of oversights and errors. Regarding errors, the 84B model was found to make the fewest errors, the 12B a little more, and the 562B the most. These error rates are similar to those shown by human radiologists in previous studies, indicating that the error rates of this AI system may be as high as those of human experts.
Finally, the figure below shows specific examples of chest x-ray reports generated by Med-PaLM M for different size models.
In this example, the radiologist rates the report generated by the 12B model as having significant errors and oversights, the 84B model rates the report as having no errors or oversights, and the 562B model rates the report as having one insignificant error but no oversights.
Summary
The paper presents Med-PaLM M, a general-purpose model useful in the medical field, and MultiMedBench, a benchmark used to develop and evaluate it. one model shows performance comparable to SOTA or SOTA on many tasks. In the future, it is expected that such versatile AIs will collaborate with highly specialized AIs and work together with actual clinicians and researchers to tackle major medical challenges.
A versatile AI that can integrate diverse medical data and respond quickly to new situations is essential for building a better healthcare system; Med-PaLM M is an important step toward creating such an AI, and further development and validation for practical use is expected.
Categories related to this article