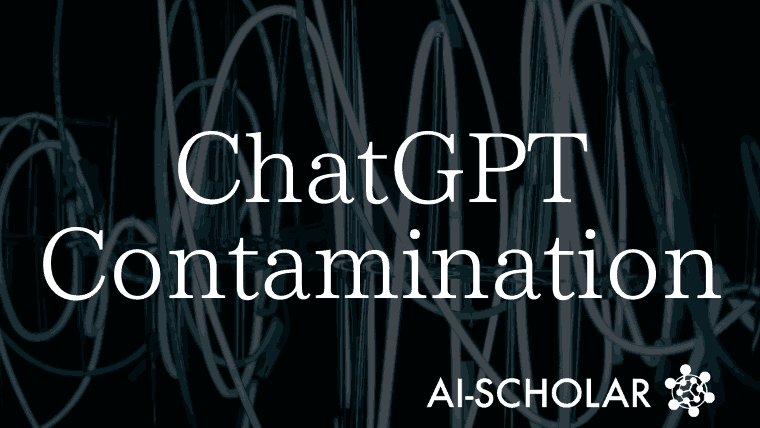
The Future Of Large-Scale Language Models And Scholarly Literature, Opportunities And Concerns Presented By The Use Of ChatGPT
3 main points
✔️ Diffusion and impact of large-scale language models: large-scale language models such as ChatGPT 3.5 are having a significant impact on the academic field, with a 2023 survey reporting that 30% of researchers are using them.
✔️ Identifying Large-Scale Language Model-Generated Texts and Their Impact: A new method of analyzing distinctive terms derived from large-scale language models easily identifies papers suspected of using large-scale language models, especially in 2023.
✔️ Ethics and future implications of large-scale language models: undisclosed use of text generation by large-scale language models raises concerns about "model collapse" and its subsequent impact on people's language choices, requiring appropriate disclosure and monitoring.
ChatGPT "contamination": estimating the prevalence of LLMs in the scholarly literature
written by Andrew Gray
(Submitted on 25 Mar 2024)
Comments: 12 pages, 6 figures
Subjects: Digital Libraries (cs.DL)
code:
The images used in this article are from the paper, the introductory slides, or were created based on them.
Summary
In recent years, large-scale language models have attracted much attention for their ability to automatically generate large amounts of high-quality text in response to human instructions. In particular, ChatGPT 3.5, released at the end of 2022, has quickly gained popularity due to the ease of use provided by its chat interface. And its use is now being actively discussed in the academic communication field. And the initial expectations have gradually shifted to a deeper understanding and appreciation of its capabilities and limitations.
According to a survey conducted in late 2023, 30% of researchers use large-scale language models for manuscript preparation, and many publishers are beginning to provide guidelines for their use; publishers such as Wiley allow the use of these tools as long as authors take full responsibility and are clear about their use. However, it is not easy to get a full picture of the impact of large-scale language model text generation on the quality of the scholarly literature. Some studies have identified papers as having been generated by large-scale language models because they contain phrases that are clearly different from those of humans, but such cases are only a small fraction of the total.
While advances in AI detection tools have made it possible to some extent to identify generated text using large-scale language models, some fields, such as physics and mathematics, have not made much use of these models. However, recent research suggests that large-scale language models may be used for peer review of conference papers, particularly in the field of artificial intelligence. These examples indicate that the use of large-scale language models is beginning to play an important role in academic communication, and future developments will be closely watched.
Identification of Terms Preferred by Large-Scale Language Models
A study by Liang et al.proposes a new approach to finding papers that utilize large-scale language models by identifying terms associated withthe text generated by the models. This method does not require analysis of the entire text and can be evaluated by simply detecting these characteristic terms.
For this approach, Liang et al. select 12 characteristic adjectives (Adjectives) and adverbs (Adverbs) and detect these words. Also available for comparison are 12 neutral words (Controls) that are commonly used in many papers.
Data was obtained from Dimensions on the number of documents matching each keyword in the Full-Text Search. Data were collected between March 18 and 22, 2024. Counts using Blank Search for all "articles" were used as a baseline, and results were calculated as a percentage of documents per year in which the keyword appeared. This baseline rises from about 3.4 million in 2015 to over 5.3 million in 2023; data for 2024 was collected but not analyzed due to incompleteness. The percentage of documents matching each term ranges from 0.02% for "lucidly" (about 1000 articles/year) to over 50% for "after" (about 2.8 million articles/year). Neutral words (Controls)appeared much more frequently than adverbs(Adverbs) and were more common than adverbs.This analysisconfirms the significant increase in the number of distinctive termsused in texts generated bylarge-scale language models and suggests the prevalence of large-scale language models in the academic literature: in articles published in 2023, even allowing for delays in the publication process since the release of ChatGPT, We expect to see this effect beginning to emerge.
Changing Terminology Preferred by Large Language Models
The three graphs below show the relative frequency change from year to year for the 36 selected words. Data are only shown from 2019 to 2023.The annual change forneutral words (Controls) was slight, as expected. Over time, some terms have gradually increased. For example, the three colors "blue," "red," and "yellow" each increased slightly from 2015 to 2023. On the other hand, other terms have either remained stable or have shown a slight decrease. These changes suggest that wording preferences in academic literature are gradually changing over time.
The change in adjectives is a bit more complex, with some adjectives increasing steadily between 2015 and 2022, while others are slowly declining. However, in 2023, the year after the release of the large-scale language model, the change is particularly pronounced: 12 adjectives (Adjectives) increased on average 33.7% between 2022 and 2023, with terms such as "intricate," "commendable," and "meticulous" notably The terms "intricate," "commendable," and "meticulous" have increased notably.
Similarly, some adverbs (Adverbs) showed a decrease from 2015 to 2022, while others showed an increase; in 2023, "meticulously" increased by 137%, while "methodically" and "innovatively" each increased by 26%. In particular, "compellingly" will increase by 26%, and "meticulously" by 26%. In particular, "compellingly" is again on the rise for 2023.These results suggest that large-scale language models are having a marked impact on language use in the academic literature.
The effect of combining terms is also much more pronounced than when using a single term. For example, in 2023,articlescontaining one or more of the first four terms considered "strong" indicatorsincreased by 83.5%. The second group containing "medium strength" indicatorsincreases by16.3%.The third group of "weak" indicators has increased by 9.3%. Finally, the fifth group of 12 combined terms, the "Strong, medium, & weak" indicator, represents over 1 million articles per year, or one-fifth of all research articles.
If the text generated by a large-scale language model tends to favor certain terms, it is possible that those terms are used more than once. using the Dimensions database to find papers that use multiple indicator terms revealed a dramatic increase in the number of results for certain pairs The results of certain pairs were found to be dramatically increased. For example, articles containing both "intricate" and "meticulous" increased sevenfold, while the combination of "intricate" and "notable" increased fourfold.
A similar trend is seen in the frequency of papers that combine two or more terms, particularly in the eighth group, which includes two "weak" terms, with a 35% increase over the previous year. Thus, analysis of term combinations also provides a more accurate picture of the scale of the impact of large-scale language models.
By leveraging the combined terminology data, we can estimate the overall number of articles that may contain text generated by the Large Scale Language Model. 2014-2022, i.e., before the Large Scale Language Model becomes widespread, the number of articles in the "Strong+Medium terms" includingThefourthgroupofarticles shows an average annual increase of 1.1%, while thefifthgroup, whichincludes "all terms,"shows an increase of 2.1%. The maximum annual change for these groups is approximately 5%. Thus, without external factors, one would expect the number of articles in these groups to increase by about 5%. Based on this estimate,666,573 and1,050,914 articles are projected for Group4andGroup5,respectively, while the actual numbers exceed 85,761 and 65,772, or 1.63% and 1.25% of all articles published in 2023, respectively.
For papers containing more than two terms,Group9(twoStrong/Mediumterms) andGroup10(two Strong/Medium/Weak terms) show the largest annual increases of about 10-11% from 2014 to 2022, and in 2023 they show the largest increases of 79.8% and a remarkable increase of 45.7%. Assuming an 11% increase in these groups,103,232 and230,338 papers are projected forGroup9andGroup10,respectively, while the actual numbers exceed 60,514 and 65,735, or 1.15% and 1.25% of the total, respectively.
However, these terms are not the only indicators used to identify ChatGPT-generated text. For example, terms such as "groundbreaking" showed a 52% increase in 2023, a higher rate of increase than other tested terms. Additionally, "outwith," a term typically used only in Scottish English, was also unexpectedly found to be preferred by ChatGPT: it almost tripled in 2023, rising 185%; "outwith" was also found to be preferred by ChatGPT, rising by 18%; and "outwith" was found to be preferred by ChatGPT, rising by 18%.Other words not tested here also show "ChatGPT style" as well and are very likely to be found in articles, which could further boost the numbers.
Summary
An analysis of papers published in 2023 indicates that an estimated 60,000+ likely contain text generated by large-scale language models. While this is not necessarily a direct indication that individual papers were generated by large-scale language models, it doessuggest that the use oflarge-scale languagemodels is widespread.
The paper states that this fact has two major implications: first, it raises the question of whether large-scale language models are used purely for appearance purposes. While a more in-depth analysis is needed, the paper states that it ispossible that large-scale language models are being used for more thansimplestylisticadjustments.
The secondconcerns the impact on thelarge-scale language modelitself. Academic literature isan important learning resource forlarge-scale languagemodels, and themore texts generated bylarge-scale language modelsare used, the greater the risk of "model collapse.This means that the quality offuturetext generation bylarge-scale language modelsmay deteriorate.
The report states that this situation requires a proactive response by publishers and reviewers. In particular, thereport suggests that rules need to be developed to clarify when the use oflarge-scale language model-generatedtext is not properly disclosed.Authors who use text generated by large-scale language models should either properly disclose their use or reconsider whether their use is indeed appropriate.
In the future, further research will be needed to accurately identify the scale of this problem, andit will be important to develop ethical guidelines for the use oflarge-scale languagemodels and to monitor the impact of their use on the academic community.It is hoped thatthis study willprovide a first step toward a deeper understanding of the impact of the use of large-scale language models on scholarly communication and the appropriate measures that can be taken.
Categories related to this article