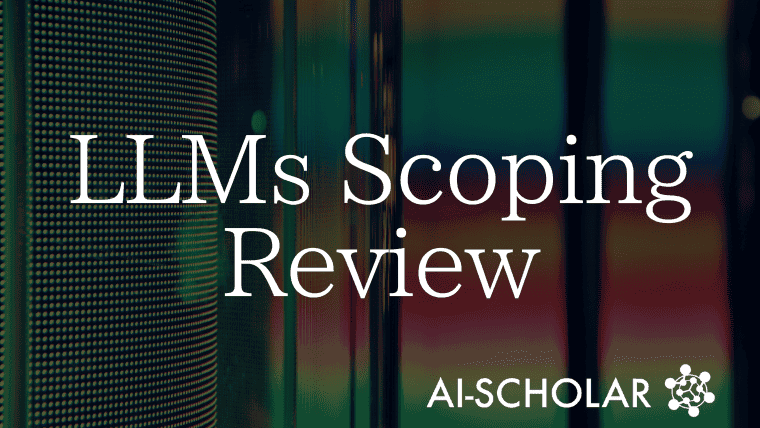
Healing The Heart With Words, The Potential Of Large-Scale Language Models In Mental Health Care
3 main points
✔️ Mental health importance and the role of large-scale language models: mental health disorders are a global health problem, and large-scale language models contribute to mental state identification and emotional support.
✔️ Comprehensive Review of Large Scale Language Models: provides the first comprehensive review of the evolution oflarge-scale languagemodels and their impact on mental health care sincethe introduction of the T5 model in 019
✔️ Identifies areas in need of improvement: notes that improving data quality, enhancing reasoning and empathy, and appropriately addressing privacy, safety, and ethics/regulation are critical to the effective use of large-scale language models in mental health care.
Large Language Models in Mental Health Care: a Scoping Review
written by Yining Hua, Fenglin Liu, Kailai Yang, Zehan Li, Yi-han Sheu, Peilin Zhou, Lauren V. Moran, Sophia Ananiadou, Andrew Beam
(Submitted on 1 Jan 2024)
Comments: Published on arxiv.
Subjects: Computation and Language (cs.CL); Artificial Intelligence (cs.AI)
code:
The images used in this article are from the paper, the introductory slides, or were created based on them.
Summary
Mental health is one of the most important areas of public health. According to the National Institute of Mental Health (NIMH), 22.8% of American adults will experience some form of mental illness in 2021. Globally, mental health disorders account for 30% of the nonfatal disease burden and are noted by the World Health Organization (WHO) to be a leading cause of disability. In addition, depression and anxiety disorders are estimated to cost the global economy $1 trillion annually. These data demonstrate how important it is to prevent and manage mental health problems.
Verbal communication is essential to mental health management, including symptom assessment and talking therapy. The analysis of such communication can be facilitated by a technique called natural language processing (NLP), a branch of computer science that processes free-form textual information in a meaningful way. In particular, advances in large-scale language modeling (LLM) are expanding the potential for innovation in mental health care. Large-scale language models efficiently summarize data from electronic health records and social media platforms, offering benefits as diverse as identifying mental states and building emotional support chatbots.
However, a comprehensive review of the use of large-scale language models in mental health care does not yet exist. This paper seeks to fill this gap by providing the first comprehensive review in this area. In particular, it examines the evolution of large-scale language models and their impact on mental health care over the past four years, focusing on models developed since the introduction of T5 in 2019.
In the field of mental health care, large-scale language models have the potential to assist with a wide range of tasks, such as interpreting behavior patterns, identifying psychological stressors, and providing emotional support, by leveraging their ability to process large amounts of text data and mimic human-like interactions. With appropriate regulatory, ethical, and privacy protections, large-scale language models are also expected to contribute to clinically oriented tasks such as supporting the diagnostic process, facilitating mental disorder management, and enhancing therapeutic interventions.
Technique
The study follows the guidelines of the 2020 edition of the Recommended Reporting Items for Systematic Reviews and Meta-Analyses (PRISMA), a rigorous and transparent process. The figure below outlines the process.
The selection of reference articles focuses on the most recent studies based on the criteria that they use at least one large-scale language model published since the publication of T5 and that these models directly address research questions in mental health care settings.
Early research revealed that there is limited published research related to this topic, particularly in PubMed. Given the rapid evolution of large-scale language models, we are expanding the scope of research beyond the traditional peer-reviewed literature.We include both peer-reviewed and non-peer-reviewed studies (e.g., preprints) to capture the latest advances inrapidly evolving, often non-traditional forms oflarge-scale languagemodeling. We included original research in any format published between October 1, 2019 and December 2, 2023. No language restrictions were set.
Multiple databases and registries (ArXiv, MedRxiv, ACM Digital Library, PubMed, Web of Science, Google Scholar) were searched extensively using the keywords "Large Language Model" and "mental OR psychiatry OR psychology", and when possible, we limit our search to titles and abstracts, and for databases that do not have this capability, we search for full text.
After removing duplicates and non-abstracted articles for the retrieved articles, 281 articles remained for initial screening. Recent studies have shown that GPT-4 can aid in article screening and perform as well as humans. For this reason, we are implementing GPT-4 as an auxiliary reviewer for this process. Prior to use, we are trying different prompts to maximize GPT-4 screening efficiency.
YH and GPT-4 independentlyreview the title and abstract ofeacharticle to assess whether the study should be included or not. There are three options: 1 (included), 0 (excluded), and 2 (uncertain). Any disagreements that arose were resolved through discussion with other members of the review team (YH, KY, ZL, FL). To quantitatively assess the level of agreement between the human reviewer (YH) and the AI (GPT-4), Cohen's Kappa coefficient was calculated, yielding a high score of approximately 0.9024. This indicates that there was strong agreement between the two sides; the GPT-4 is generally more inclusive,classifyingmorearticles as relevant to mental health carethan thehumanreviewers.However, the "uncertain" option, while slightly lowering the Kappa coefficient,is important for the comprehensive inclusion ofrelevantpapers and helps to balance thoroughness and accuracy.
Forty-threepapers were selected forthe final full-text review.Team members YH, KY, ZL, and FLscrutinizedall of thesepapersandexcluded9papers because they were oflow quality, treated mental health only as a test case, or did not meet the model size criteria. Specifically, one paper was excluded due to low quality, three papers were excluded due to treating mental health only as a test case, and five papers were excluded due to inadequate model size.
During the review process, studies were grouped into the following categories based on their respective research questions and objectives
- Datasets and Benchmarking: studies that use standardized test or benchmark datasets to evaluate and compare the performance of different methods, systems, or models under controlled conditions.
- Model Development and Fine Tuning: research to propose new large-scale language models and to improve and adapt existing large-scale language models for mental health care using methods such as fine tuning and prompting.
- Application and Evaluation: Studies evaluating the performance of large-scale language models on mental health-related tasks in real-world applications. This includes the case of evaluation of large-scale language models in specific tasks (inference only).
- Ethical, privacy, and security considerations: a study that examines the potential risks, ethical dilemmas, and privacy issues associated with deploying large-scale language models in sensitive mental health contexts and proposes frameworks and guidelines to mitigate them.
Thirty-four papers that met these criteria are included in the subsequent analysis. To focus on applications to the research problem and ensure a thorough analysis, the "Data Sets and Benchmarks" studies are summarized separately.
Summary of Results
The figure below shows the timing and types of papers submitted and published included in the final analysis. As shown in this figure, research on large-scale language models in mental health care appeared in September 2022, with a gradual increase in publication volume, particularly a marked spike in October.
The majority of these studies focused on "Prompt Tuning and Applications" and began increasing in July. On the other hand, studies on "Model Development and Fine Tuning" were few and far between at the beginning of the year, with a noticeable increase in October. Only two studies on "Data Sets and Benchmarking" were published during the year, and only one study dealing with ethics, privacy, and other issues was published in the middle of the year.
Areas of Application and Associated Mental Health Conditions
Throughout the review, strong associations have been found between the scope of the studies and the data sets used in them. This section provides an overview of the areas of application throughout these studies and the mental health conditions they are intended to target. The table below provides a detailed summary of the datasets utilized in these studies, detailing their intended use.
Areas of Application and Associated Mental Health Conditions
Research on large-scale language models (LLMs) in the mental health care field took shape in September 2022. The number of published studies gradually increased, with a particularly noticeable spike in October. Figure 2, which illustrates this trend, shows that those studies were concentrated in "Prompt Tuning and Applications," and their numbers began to increase in July. In contrast, studies on "Model Development and Fine Tuning" were virtually absent at the beginning of the year and showed a significant increase in October. In addition, only two studies focused on "Data Sets and Benchmarking" appeared later in the year. Only one study dealing with ethics, privacy, and other concerns was published in the middle of the year.
Research onlarge-scale languagemodelsrelevant to mental health carespans three major areas: the first is the development of conversational agents aimed at improving the ability of models to generate empathic and contextual responses. These agents address a wide range of mental health needs without being specific to a particular mental disorder. Also included are studies aimed at interacting directly with people seeking support through a variety of platforms, such as personal digital companions, on-demand online counseling, and emotional support. Some studies extend to specific applications such as couples therapy. Other studies provide specific recommendations and analyses to assist care providers and alleviate the problem of provider shortages.
The second area of research is aimed at resource enrichment. This includes multitasking analysis and the development of educational content, including the creation of virtual case vignettes and personalized psychoeducational materials related to social psychiatry.In addition, we are working on data augmentation and fine-tuning of clinical questionnaires to enrich the symptomology of depression, utilizing synthetic data provided bylarge-scale languagemodels.
In the third area,large-scale linguisticmodels are utilized as classification models for detailed diagnosis. Thisfrequently involvesbinary classification, which detects the presence or absence of a single condition in a given context, andmulti-class classification, which includesmore detailedinformation about thecondition, its severity and subtypes.
Examples of multiclass classifications include predicting severity of depression (minimal, mild, moderate, and severe according to DSM-5), subtypes of suicide (support, indicators, thoughts, behaviors, and attempts according to the Columbia Suicide Severity Rating Scale (C-SSRS)), and identifying sources of stress (school, financial, family, social relationships, and other categories established in the SAD based on the SAD) included.
Of the 34 articles reviewed, 23 focused on specific mental health issues, while the remainder explored general mental health knowledge and dialogue without specific conditions. Studies on specific mental health issues cover a variety of mental health conditions, including frequently studied conditions such as stress, suicide, and depression.
Models and Learning Techniques
To gain insight into the evolution and application of large-scale language models in mental health care, the focus here is on models and training techniques. The effectiveness of a pre-trained model is highly dependent on the fundamental factors of training data, size, and whether it is open source. Together, these determine how representative or potentially biased a model is for a particular task or population.
In the table below is a summary of existing large-scale language models developed for mental health care. This summary includes details on the base model, its size as indicated by the number of parameters, transparency of the base model training data, strategies employed during training, and information on accessibility if it is open source. The "B" stands for billion. TFP and IFT standfor"tuning-free prompting" and "instruction fine-tuning," respectively.
Many studies are directly prompted by models such as GPT-3.5 and GPT-4 and are dedicated to mental health applications such as depression detection, suicide detection, cognitive distortion detection, and relationship counseling. These models act as intelligent chatbots that provide a wide range of mental health services, including analysis, prediction, and support. To increase effectiveness, methods such as fourshot prompting and chain-of-thought (CoT) prompting are used. These are novel approaches for generating cognitive inferences about human emotions in large-scale language models.
Some studies have also focused on further training or fine-tuninggenerallarge-scale languagemodelswith mental health-specific text.This approachaims to infuse mental health knowledge intoexisting basedlarge-scale languagemodels for more relevant and accurate analysis and support. projects such as MentaLLaMA and Mental-LLM use social media data to train LLaMA-2 ChatCounselor is using the Psych8k dataset, which includes actual interactions between clients and psychologists, to fine-tune LLaMA models. .
Given the high cost and extensive time involved in training large-scale language models from scratch, existing studies have consistently adopted the approach of fine-tuning existing models on mental health data. The goal is to enhance existing models on mental health data. This approach allows models to acquire specialized domain knowledge andevolve into large-scale language modelsfocused on mental health.All of the studies that have employed fine tuning have employed Instructional Fine Tuning (IFT) technology. Instructional Fine Tuning (IFT) technology is a new type of fine tuning that instructs a model to perform a task. This methodinjects domain knowledge intolarge languagemodels to improve their ability to follow human instructions. For example, ChatCounselor gives GPT-4 instructions based on a conversation between a client and a psychologist to generate specific inputs and outputs. In this way,large-scale languagemodels could be more appropriately used in the field of mental health care.
Dataset Characteristics
Data integrity plays an important role in research in the mental health care field. In particular, the representativeness, quality, and potential bias of data sets can significantly impact research outcomes, so an accurate understanding of the sources and characteristics of data sets is essential for fair research results. This paper provides a detailed review of the datasets used and their associated tasks, data sources, sample sizes, annotation methods, humanreviewerexperience, and licensing as listed in the table below.
Thirty-six datasets were identified for the 34 studies reviewed, and these contain a wide variety of data applicable to mental health care tasks. Most of the datasets are dedicated to detection and classification tasks, including detection of depression and post-traumatic stress disorder (PTSD), identification of stress causes, and prediction of interpersonal risk factors. Another group focuses on text generation tasks, such as simulating counseling sessions, responding to medical inquiries, and generating empathic dialogue. More specialized applications include user argument analysis of large language models of emotional support and dialogue safety exploration.
Datasets are often collected from social media platforms such as Reddit, Twitter, and Weibo, with some datasets coming from controlled locations, but also data synthesized by LLMs, existing sentiment dictionaries, crowdworker-simulated simulated conversations, etc., from other sources.
Dataset sizes and units vary by source and annotation method, and datasets consisting of expert content tend to be small samples. Most datasets are created by manual collection and annotation, andsome studies use weak supervised learning. The majority of datasets have been peer reviewed, with many studies relying on publicly available datasets, and some are independently constructed datasets, but released under a license that limits their use to non-commercial purposes.
Validation Index
The selection of validation metrics is crucial for effective and unbiased evaluation of large-scale language models (LLMs). In this paper, we analyze two categories: automatic evaluation and human evaluation. The table below summarizes the metrics for automated assessment and details the attributes used for human assessment. The metrics are further categorized from the two perspectives of language proficiency and mental health applicability, and the appropriateness of each is discussed.
With respect to mental health applicability, the different forms of the F1 score are the most commonly employed indicators. Similarly, Accuracy is also widely used as a basic indicator. Recall (Sensitivity) and Precision (Precision) are also widely used, often together with the F1 score and accuracy. Diagnosis-specific studies employ additional metrics, such as the receiver operating characteristics (AUROC) and Specificity, to achieve a comprehensive understanding of the diagnostic validity of large-scale language models.
BLEU, ROUGE, Distinct-N, and METEOR are widely used to assess human-like language conformity, expressive diversity, and quality of produced text; advanced metrics such as GPT3-Score, BARTScore, and BERT-Score are designed to assess the semantic coherence and relevance of text in a given context. Perplexity is used to assess the predictability of the model and the naturalness of the text, while Extrema and Vector Extrema reflect the linguistic creativity and depth of the model. The use of these traditional language evaluation metrics is driven by the lack of efficient and understandable automated metrics to assess the quality of free text generation in large-scale language models in mental health care. As a result, many studies frequently employ human assessment.
Of the 34 studies reviewed, 19 used a combination of automated and human ratings, 5 employed only human ratings, and the remaining 10 relied solely on automated methods. However, there is no widely accepted uniform rating framework, and while some studies apply or adapt published rating criteria or attributes discussed in previous studies, these frameworks have not been widely adopted. Frequently overlapping attributes such as empathy, relevance, fluency, comprehension, and usefulness are used to evaluate aspects such as user engagement and technology adoption, especially in intervention applications. Some attributes, while sharing a name, may have different definitions across studies. For example, "informativeness" may relate to the richness of responses in a large-scale language model, or it may measure the degree to which an individual provides a detailed account of emotional distress. Expert ratings focus on direct analysis of model outputs and expert questionnaire ratings. The use of reliability metrics is important to validate the research methodology, andthe number ofreviewersranges from 3 to 50.
Matter of Concern
Privacy issues associated with the use of large-scale language models (LLMs) in mental health care are of particular concern throughout the study. In particular, the nature of the sensitive data handled by mental health care applications underscores this. Several studies highlight the risks of exposure of sensitive data and call for strict data protection and ethical standards. Security and reliability are also cited as fundamental requirements, with emphasis on preventing the generation of harmful content and ensuring the provision of accurate and relevant responses.
Focus is also on the critical balance of ensuring safety while leveraging the benefits oflarge-scale languagemodels, with attention to the ongoing pursuit of risk assessment, reliability, and consistency in mental health support Concerns about the potential for neglect and overreliance on real-world interaction caused by increased reliance on AI The report also includes a discussion of the importance of the use of AI in the context of the public health system. Content containing inaccuracies or bias can seriously impact perceptions and decision-making in mental health contexts.
Technical and performance challenges range from model limitations and generalization issues to memory and context limitations. These issues particularly impact the reliability and effectiveness of AI applications in complex real-world settings. The need for performance variability, robustness, and transparency is an area that demands continuous innovation and scrutiny.
Moving to real-world applications brings additional complexity, especially in mental health, where accuracy and sensitivity are required. The challenges of long-term effects, differences between laboratory and real-world settings, and accessibility and digital disparitiesrepresent challenges in bridging the gap between the potential oflarge-scale languagemodels and their practical implementation.
It also emphasizes the importance of diverse and extensive data sets, specialized training, and data annotation. These are key elements in moving the field forward in a responsible manner. Large-scale computational resources and expert participation are also cited as critical to this progress.
The reviewincludes benchmark studies to objectively assess the effectiveness oflarge-scale languagemodels inmental health careand to identify areas in need of improvement.Two benchmark studies have been conducted to date, in which models such as GPT-4, GPT-3.5, Alpaca, Vicuna, and LLaMA-2 were comprehensively evaluated on a variety of tasks ranging from diagnostic prediction to emotion analysis, language modeling, and question answering. Data were obtained from social media and therapy sessions. The Qi et al. study focused specifically on classification of cognitive distortions and prediction of suicide risk using Chinese social media data, with models such as ChatGLM2-6B and GPT-3.5 being evaluated.
Summary
This is the firstcomprehensive review ofthe evolution of large-scale language models (LLMs) in the field of mental health care since the introduction of the T5 model in 2019; it scrutinizes 34 relevant studies and provides an overview of the various characteristics, methodologies, data sets, validation metrics, application areas, and specific mental health issues are comprehensively summarized. This comprehensive review is intended to serve as a bridge between the computer science and mental health communities and to share the insights gained widely.
Large-scale languagemodels are algorithms that demonstrate exceptional capabilities in the area of natural language processing (NLP). These models closely match the requirements of mental health-related tasks and have the potential to be foundational tools in this area.However, while it has been suggested thatlarge-scale languagemodels may contribute to improved mental health care, there remains a significant gap between the current state of the art and their actual clinical applicability.
Therefore, this paperoffers thefollowingdirections for improvement needed to maximize the potential oflarge-scale languagemodelsin clinical practice.
- Improving Data Quality: The quality of the data used to develop and validate large-scale language models has a direct impact on their effectiveness. Prompt tuning is currently the predominant method employed, but models such as GPT-3.5 and GPT-4 occasionally fail to meet expectations in complex mental health contexts. To address these challenges, there is a need to explore fine-tuning techniques to open-source large-scale language models.
- Enhanced reasoning and empathy: Dialogue-based tasks in mental health care require advanced reasoning and empathy skills to analyze statements from users and provide appropriate feedback. The lack of a unified framework for assessing these abilities has affected the development of the entire field.
- Privacy, Safety, and Ethical/Regulatory Compliance: Strict adherence to patient privacy, safety, and ethical standards is essential when applying large-scale language models to mental health applications. Compliance with data protection regulations, model transparency, and informed consent must be ensured.
This reviewhighlights the current state of the art and future possibilities for the use oflarge-scale languagemodels inmental health care.Technological advances, standardization of assessment criteria, and collaboration toward ethical use are key to facilitating further progress in this area.It is hoped that this will enable large-scale language models to realize their full potential in supporting mental health care.
Categories related to this article