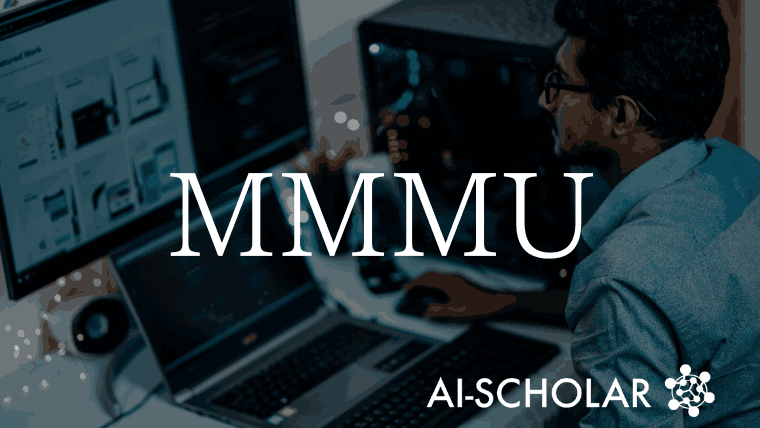
Tackling College-Level Liberal Arts: MMMU, A New Benchmark For Large-Scale Multimodal Models
3 main points
✔️ Raises the importance of methods to assess progress in "expert AGI," defined as level 3 of general-purpose artificial intelligence (AGI).
✔️ Proposes a new benchmark, MMMU, for assessing multimodal understanding at the university level to evaluate the expertise and reasoning capabilities of AI models.
✔️ Noted that current AI models (including GPT-4V) perform poorly on MMMU and need further improvement to achieve expert AGI.
MMMU: A Massive Multi-discipline Multimodal Understanding and Reasoning Benchmark for Expert AGI
written by Xiang Yue, Yuansheng Ni, Kai Zhang, Tianyu Zheng, Ruoqi Liu, Ge Zhang, Samuel Stevens, Dongfu Jiang, Weiming Ren, Yuxuan Sun, Cong Wei, Botao Yu, Ruibin Yuan, Renliang Sun, Ming Yin, Boyuan Zheng, Zhenzhu Yang, Yibo Liu, Wenhao Huang, Huan Sun, Yu Su, Wenhu Chen
(Submitted on 27 Nov 2023)
Comments: Published on arxiv.
Subjects: Computation and Language (cs.CL); Artificial Intelligence (cs.AI); Computer Vision and Pattern Recognition (cs.CV)
code:
The images used in this article are from the paper, from the introductory slides, or were created based on them.
Summary
Rapid progress in large-scale language modeling has stimulated discussion of Artificial General Intelligence (AGI), for which Morris et al. have proposed a clear definition and hierarchical classification. Of particular importance is Level 3, "Expert AGI," which refers to AI that is comparable to the top 10% of skilled adults in many tasks. job losses and economic impact. It is important to keep a close eye on the progress of expert AGI.
However, the question is how to measure that "expert AGI" progress. As a benchmark, a college-level exam is useful. While previous benchmarks have focused on text-based questions, humans can solve a wide variety of problems involving images as well as text. Therefore, the focus is on large-scale multimodal models that understand both text and images. These have performed well in existing multimodal benchmarks. However, these benchmarks focus on common sense and everyday knowledge rather than expert knowledge and are therefore insufficient for evaluating expert AGI.
To solve this problem, the paper proposes a new benchmark called MMMU. It is dedicated to multidisciplinary multimodal comprehension and reasoning at the college level and covers six disciplines: arts and design, business, science, health and medicine, humanities and social sciences, and technology and engineering. It contains approximately 11,500 diverse questions drawn from college exams and textbooks, which span 30 subjects and 183 subfields and include various types of images (e.g., charts, maps, sheet music).
The MMMU includes questions that require expert-level reasoning and in-depth knowledge. It also tests your understanding of different image formats and your ability to solve problems that combine text and images.
Fourteen open source models and GPT-4V were evaluated on this benchmark, with GPT-4V achieving only 56% accuracy at best, indicating the need for significant improvements in AI models MMMU offers a new approach to measuring the progress of expert AGI. With this benchmark, we aim to facilitate the development of more professional and advanced artificial intelligence.
What is MMMU Benchmarking?
The dataset included in MMMU covers 30 subjects and 183 subfields in six disciplines (Arts and Design, Business, Science, Health and Medicine, Humanities and Social Sciences, and Technology and Engineering), with detailed subjects and statistics as follows Benchmark questions are manually collected from online sources, textbooks, and lecture materials by 50 university students (including co-authors).
In the process of collecting data, we are looking at the major majors at the college to determine which subjects to include. The selection criterion is whether the subject requires visual information. Based on this criterion, we exclude subjects such as law and linguistics, which have little relevant visual material. As a result, we have selected 30 subjects from six different disciplines. Next, we employ more than 50 college students from these majors as annotators to collect questions. They collect diverse questions from textbooks and online resources and create new questions based on their own expertise. They are instructed, however, to avoid data from sites where copying and redistribution are prohibited. Ultimately, we have collected over 13,000 questions from a variety of sources.
Next, we conduct a two-stage data cleaning process to improve data quality. In the first stage, potential duplicate issues are identified and eliminated. In the second stage, the co-authors check the formatting and typos of the issues and correct them as needed. Finally, the questions are categorized into four difficulty levels: very easy, easy, normal, and difficult, and approximately 10% of the very easy questions are eliminated to ensure the quality and difficulty of the question set.
Unlike other benchmarks, this benchmark covers college-level knowledge. Traditional benchmarks focus primarily on everyday knowledge and common sense, and the types of images are limited. However, this benchmark aims to cover a wide range of content, including 30 different image formats, such as diagrams, tables, charts, chemical structures, photographs, paintings, geometric shapes, musical scores, and medical images. Also, whereas traditional benchmarks require general knowledge and simple theoretical reasoning, this benchmark requires more advanced reasoning using college-level subject knowledge.
Experimental results
The results of the comparative validation of the Large Scale Language Model (LLM) and the Large Scale Multimodal Model (LMM) using the MMMU benchmark are shown in the table below. It can be seen that this is a very advanced benchmark for current large-scale language models (LLMs) and large-scale multimodal models (LMMs). Even GPT-4V, which is considered the most advanced, is only 55.7% accurate, indicating significant room for improvement. This is reflected in the fact that the benchmark has high requirements aimed at AGI.
The large performance difference between proprietary models such as GPT-4V and open source models shows that major open source models such as BLIP2-FLAN-T5-XXL and LLaVA-1.5 reach an accuracy of about 34%, which is significantly lower than the GPT-4V's about 56 Accuracy.
Comparing the performance of the datasets by field, the performance is relatively high in fields where the images are more "natural" and require reasoning with relatively few problems, such as art and design and the humanities and social sciences. Conversely, it performs poorly in areas such as science, health and medicine, and technology and engineering, where many tasks require complex perception and complex reasoning.
In addition, we have also conducted an error analysis on GPT-4V, examining 150 randomly sampled error cases from the GPT-4V predictions. These cases are analyzed by specialized annotators. The distribution of errors is shown in the figure below, with Perceptual Error being the most common GPT-4V error.
Summary
This paper proposes a new benchmark, MMMU, to assess the capability of large-scale language models (LLMs) and large-scale multimodal models (LMMs). Proposed as an important benchmark for assessing the progress of expert AGI, MMMU can not only indicate the limits of the basic perceptual abilities of current large-scale language models (LLMs) and large-scale multimodal models (LMMs), but also their ability to handle complex reasoning and deep knowledge. It requires the expertise and reasoning skills expected of adults with expertise in a variety of specialized fields and is highly useful as a benchmark for assessing progress in expert AGI.
Categories related to this article