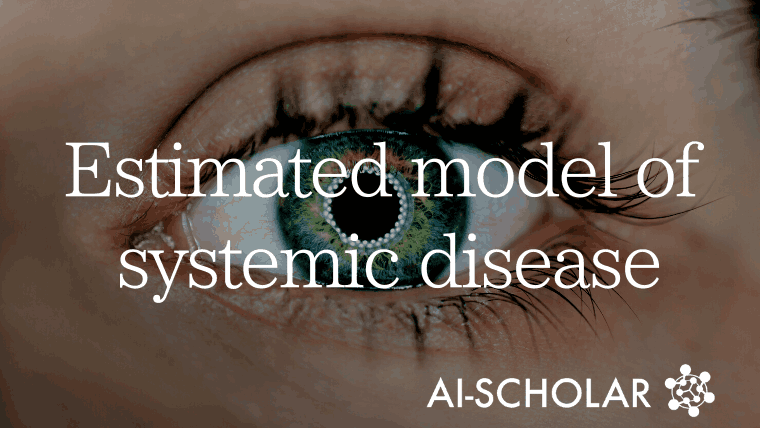
Systemic Diseases From Eye Images! A Proposed Model For Estimating Systemic Disease Using Fundus Images!
3 main points
✔️ Early detection is more important than a diagnosis in chronic diseases such as diabetes because it is often difficult to improve the disease after onset.
✔️ In this study, we used deep learning to combine fundus images and clinical metadata - age, gender, height, weight, body mass index, and blood pressure - to identify chronic kidney diseases (CKD -✔️ This study uses deep learning to build a learning model to discriminate between chronic kidney diseases (CKD) and type 2 diabetes mellitus (T2DM) by combining fundus images and clinical metadata - age, gender, height, weight, index, and blood pressure.
✔️ The evaluation results confirmed that the estimation performance -Area under ROC curve: AUC- of the proposed model achieved 0.85-0.93.
Deep-learning models for the detection and incidence prediction of chronic kidney disease and type 2 diabetes from retinal fundus images
written by Kang Zhang, Xiaohong Liu, Jie Xu, Jin Yuan, Wenjia Cai, Ting Chen, Kai Wang, Yuanxu Gao, Sheng Nie, Xiaodong Xu, Xiaoqi Qin, Yuandong Su, Wenqin Xu, Andrea Olvera, Kanmin Xue, Zhihuan Li, Meixia Zhang, Xiaoxi Zeng, Charlotte L. Zhang, Oulan Li, Edward E. Zhang, Jie Zhu, Yiming Xu, Daniel Kermany, Kaixin Zhou, Ying Pan, Shaoyun Li, Iat Fan Lai, Ying Chi, Changuang Wang, Michelle Pei, Guangxi Zang, Qi Zhang, Johnson Lau, Dennis Lam, Xiaoguang Zou, Aizezi Wumaier, Jianquan Wang, Yin Shen, Fan Fan Hou, Ping Zhang, Tao Xu, Yong Zhou, Guangyu Wang
(Submitted on 1 Jul 2021)
Comments: Nature Biomedical Engineering
The images used in this article are from the paper, the introductory slides, or were created based on them.
backdrop
Is it possible to estimate systemic diseases from fundus images?
In this study, we aim to construct a prediction model for CKD and T2DM based on a time series analysis of CNN by utilizing fundus images.
Systemic diseases such as CKD and T2DM are strongly associated with mortality risk, along with cancer and cardiovascular disease - not only do they require dialysis and transplantation, but they are also strongly associated with the development of cardiovascular and other diseases and mortality. In many of these cases, the disease is irreversible and difficult to correct once it has developed, making early diagnosis and treatment important. Regular health check-ups are a common measure for early detection, diagnosis, and prevention of progression of CKD and T2DM; however, these check-ups require resources, such as a measurer with specific skills, to perform them - and therefore, in an environment with limited resources, there is a need for, Therefore, new methods of early prevention are needed to replace medical checkups in environments with limited resources. In this context, attention has focused on fundus imaging, which allows direct observation of blood vessels from outside the body: the retina of the eye allows noninvasive observation of blood vessels, nerves, connective tissue, and the dynamic movement of blood vessels, and the fundus is likely to show specific symptoms of a systemic disease if it is present. On the other hand, based on these images, there are limited investigations on the prediction of pathogenesis related to CKD and T2DM and models for early diagnosis of CKD using deep learning, and the estimation performance is currently unclear.
This study aims to build a learning model to detect CKD and T2DM by analyzing fundus images: specifically, a regression task to predict continuous values-i.e., estimated Glomerular Filtration Rate (eGFR)-and to validate the model. To construct and validate an analysis model of fundus images based on deep learning against binary classification for diagnosis: validation suggests that fundus images can be used to achieve high accuracy in predicting disease onset and risk stratification.
What is chronic kidney disease (CKD)?
First, a brief description of CKD, the subject of this analysis, is given.
CKD is a collective term for diseases that cause a chronic decline in kidney function - i.g. diabetes, hypertension, and chronic nephritis. It is characterized by decreased renal function due to increased risk factors for atherosclerosis such as blood glucose and LDL cholesterol - in other words, decreased vascular function due to lifestyle-related vascular dysfunction, resulting in decreased function of the glomerulus, a mass of capillaries, and decreased renal function. In general, the disease is asymptomatic in the early stages, and once it worsens, it is difficult to improve, making early detection and treatment important.
technique
proposed model
Here is an overview of the proposed model utilized in this study - see figure below.
The proposed model is based on ResNet-50, pre-trained on ImageNet, and built by transition learning: ResNet-50 has one convolution and four blocks, and introduces skip connections - this allows us to learn at deeper layers while avoiding gradient loss. This allows us to learn at deeper layers while avoiding gradient loss. For the regression task of continuous value prediction - i.g. fasting blood glucose - we defined a Dense layer with one scalar as the final layer of the ResNet-50 model, and for binary classification, we added Dense + Softmax. In the re-training, we set the weights in the CNN layer and initialized the layers for regression and binary classification. We also introduced a three-layer MLP in the Dense layer - two of the hidden layers have 128 nodes and a ReLU activation function. As loss functions, we set MSE loss for continuous value prediction and cross entropy loss for binary classification. The error backpropagation method was performed on 32 images resized to 512×512 pixels in batches of 50 epochs with a learning rate of 10-3. Adam optimizer was used as the optimization function and the weight decay was set to 10-6. The dataset was randomly split into 7/8 for training and 1/8 for validation. The model based on clinical data consisted of age, gender, blood pressure, height, weight, BMI, hypertension, and T2DM, and the combined model combined with fundus images to predict the risk of progression. The predicted risk scores were categorized into three groups, low-risk, intermediate-risk, and high-risk, based on upper and lower quartiles, according to the risk score at the initial visit - risk scores were addressed as categorical variables, based on quartiles.
Pathogenesis analysis of CKD and T2DM
For the incidence analysis in these diseases, we included data from the time point of no CKD at baseline: all participants who had a negative urine test at baseline and met the criteria for CKD- or advanced+ CKD- during follow-up were included. We defined subjects who met the criteria for CKD- or advanced+ CKD- during follow-up as incidents. Similarly, for T2DM, we defined the onset of T2DM for subjects who were T2DM-naïve at the initial visit and met the criteria at follow-up.
result
This section describes the evaluation.
Accuracy of CKD estimation
We tested whether fundus images and clinical data - i.g. age, gender, height, weight, and blood pressure - can predict the early detection and severity of CKD: Here, we constructed and tested a baseline model using clinical data - the Random forest-, a proposed model using fundus images, and a learning model utilizing both clinical data and fundus images were constructed and validated.
The estimation performance-AUC- of these learning models was as follows: Random forest: 0.861; fundus images only: 0.918; combined clinical data and fundus images: 0.930-see the figure below. In addition, to demonstrate the generality of these models, they were separately validated in an external cohort, and the estimated performance-AUC-were as follows: clinical data: 0.842; fundus images: 0.885; combined model of clinical data and fundus images: 0. 898.
T2DM Prediction
A T2DM detection model was constructed by utilizing fundus images and deep learning models. The dataset was partitioned and trained in the following ratio - training: validation: testing = 7:1:2. data only: 0.828; fundus images only: 0.923; combined model: 0.929 - see figure below. In addition, the evaluation using external test data was as follows: clinical data only: 0.796; fundus images only: 0.854; combined model: 0.871. In addition, the evaluation of external test data using images captured by a smartphone camera was as follows: clinical data only: 0.828; fundus images only: 0.923; combined model: 0.929 - see figure below. In addition, external test data using images captured by a smartphone camera were evaluated as follows: clinical data only: 0.762; fundus only: 0.820; combined model: 0.845. Therefore, we also validated the results in patients who did not have DR. We divided the T2DM patients into two subsets-DR and NDR-and evaluated the subsets. The results showed that the performance of the proposed model was similar in estimating T2DM with and without DR: suggesting that the performance of the proposed model is largely independent of DR; therefore, these results suggest that the proposed model can detect T2DM based on fundus images before the onset of DR - with or without DR. -, suggesting that the proposed model can detect T2DM. In addition, we evaluated a model that predicts mean blood glucose levels from fundus images alone, suggesting that it can predict and quantify blood glucose levels from fundus images alone.
consideration
In this study, we developed an estimation model for predicting the onset of CKD and T2DM using fundus images - we speculate that the estimation model using such data can be applied not only to fundus images taken by dedicated fundus imaging cameras but also to fundus images taken using smartphones and other devices The estimation model using such data can be applied not only to images taken with a camera dedicated to fundus imaging but also fundus images taken with smartphones and other devices. Based on the evaluation results of this study, these findings have a high potential to realize a non-invasive and low-cost screening method for the early detection of CKD and T2DM.
On the other hand, challenges in this study may include racial bias; model building to account for rapid renal disease; and additional clinical data. Since the present study was trained and tested based on a dataset with a large proportion of patients from China, it may be necessary to derive the estimation performance in other racial groups - i.e., Western and Western - to validate the generalizability of the proposed model. In this study, as an additional validation, the model is evaluated in two other external multiethnic validation cohorts, Kashi-Kashgar in Xinjiang Uygur Autonomous Region and Macau-Portugal, and it is shown that the model achieves high estimation performance. Therefore, we speculate that additional datasets for a larger number of clinical and demographic cohorts could be leveraged to expand the diagnostic accuracy and clinical utility in a wider population; second, the challenges associated with the measurement of eGFR measurement: In diseases with rapid deterioration of renal function - i.g. acute kidney injury (AKI) - the progression of the disease is rapid - a different behavior from the usual slow progression of CKD - and therefore the estimation performance for these diseases is poor. Therefore, the estimation performance for these diseases is likely to be poor. Therefore, in this study, patients with AKI comorbidities - or with a high probability of developing AKI - were excluded from the evaluation; thirdly, the addition of clinical metadata - i.g. blood pressure trends, smoking status, alcohol intake levels, family history The accuracy of prediction needs to be examined to see if the addition of clinical metadata - i.e., blood pressure history, smoking status, alcohol intake level, and family history - would improve the accuracy of prediction.
Categories related to this article