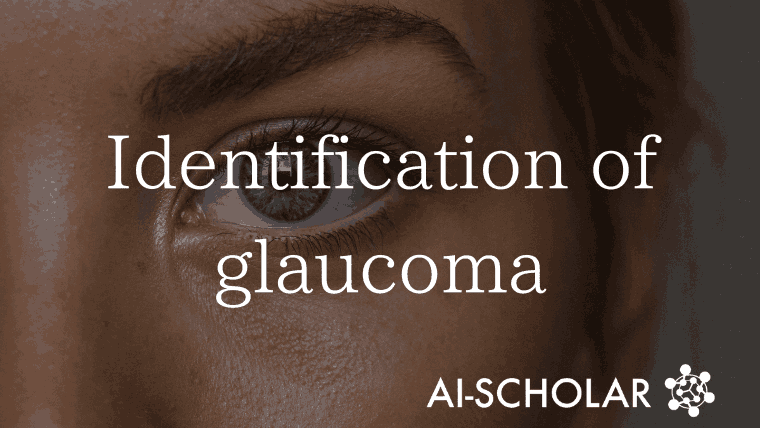
AI Goes Beyond Ophthalmology Specialists! A Proposed Model For Automated Diagnosis Of Glaucoma Using Fundus Images!
3 main points
✔️ Since the diagnosis of glaucoma requires expertise and years of experience, there is a need to develop algorithms to automate these processes.
✔️ This study aims to construct an automated glaucoma diagnosis algorithm using a convolutional neural network - convolutional neural network: CNN-.
✔️ The evaluation results confirmed that the algorithm achieved 93.86% accuracy, 85.42% sensitivity, and 100% specificity.
Identification of glaucoma from fundus images using deep learning techniques
written by S Ajitha, John D Akkara, M V Judy
(Submitted on 25 Sep 2021)
Comments: Front Public Health
The images used in this article are from the paper, the introductory slides, or were created based on them.
background
Can deep learning go beyond ophthalmology specialists?
This study aims to build an algorithm for automatic diagnosis of glaucoma using deep learning based on fundus images.
Glaucoma is a disease in which elevated intraocular pressure causes the nerve fibers responsible for transmitting information to the brain to become constricted, resulting in optic nerve damage- these symptoms can lead to permanent blindness and extreme visual field loss. In this disease, peripheral visual field defects occur while subjective symptoms are minimal in the early stages of the disease.
In addition, since it is difficult to restore the optic nerve with current medical care, once the disease becomes severe, it is extremely difficult to improve it thereafter - therefore, early detection and prevention are important in view of improving prognosis. On the other hand, these diagnoses require specialized knowledge - highly specialized training and knowledge - and are difficult to evaluate by inexperienced people - i.g., patients and residents.
In addition, evaluation methods for glaucoma rely heavily on manual work by ophthalmologists using slit-lamp biomicroscopy and depend on clinical expertise - which can lead to high inter-examiner variability and high potential for misdiagnosis and waste of clinical information.
Therefore, a new method for the early diagnosis of glaucoma - one that is less dependent on specialized technology - is needed. Against this background, this study aims to construct an automatic glaucoma diagnosis algorithm using CNNs. In building the algorithm, we will train the model on four different datasets to improve the versatility of the model.
What is glaucoma?
First, an overview of glaucoma, the subject of this study's analysis, is given.
Glaucoma is a disease that causes damage to the optic nerve due to increased intraocular pressure, resulting in a narrowing of the visual field and eventual loss of vision. The incidence of glaucoma tends to increase with age, with 5% of those over 40 years of age and more than 10% of those over 60, and the cause of the disease is currently unknown.
It is believed that it is difficult to restore the optic nerve once it has died due to increased intraocular pressure; on the other hand, early detection and early intervention are important because it is possible to maintain visual field and vision with early detection and appropriate treatment.
In addition, intraocular pressure does not cause clear symptoms until it becomes considerably elevated, and in addition, in many cases, visual field constriction is not noticed in both eyes, so the disease often progresses without subjective symptoms.
technique
This section describes the proposed model for this study - see figure below.
The training dataset consisted of 1113 fundus images from various ethnicities, age groups, and illumination levels. 512 pixels. The data set was also randomly divided into three parts: 70% - 462 normal and 317 glaucomatous images - for training, 20% - 132 normal and 88 glaucomatous images - for validation, and the remaining 10% % - 66 normal and 48 glaucoma - were used for testing.
In the architecture of the CNN model - shown below - it was constructed based on 10 convolutional layers and 3 fully coupled -Dense- layers, with normalized images as input. We used RELU applied to all coupled and convolutional layers except the last layer, and utilized SoftMax as the activation function. In addition, regularizations such as data balancing and augmentation were applied to develop an efficient and successful DL model - especially for medical data, where it can be difficult to prepare a large set of labeled data due to annotation costs, These strategies will also be considered.
In the proposed model, a data balancing method - Synthetic Minority Oversampling Technique - was applied to generate a composite sample and balancing was performed to match normal and glaucoma images: As a result, 12012 fundus images were generated and trained. Adam Optimizer was used as the optimization function, with a default learning rate of 0.001 and a batch size of 32. Categorical cross entropy was applied to the loss function to calculate the error.
result
This section describes the evaluation.
Specificity, sensitivity, accuracy, and area under ROC curve-AUC-were used to evaluate model performance: sensitivity is the probability of a positive diagnosis of glaucoma; specificity is the probability of a positive diagnosis in a non-glaucomatous person; accuracy is the classification result from the diagnosis Overall probability. The hyperparameters were trained with batch sizes of 8, 16, 32, 64, and 128 and 50 epochs - see figure below: these curves show that the proposed model does not over-learn - it maintains the same prediction performance on new test data. These curves show that the proposed model is not overtrained - it maintains the same prediction performance on new test data.The accuracy was 99.26% when the batch size was set to 32.
From the confusion matrix in the classification model - a table summarizing the correctness of predictions due to true positives, false positives, true negatives, and false negatives - we compared performance with SoftMax and SVM classifiers: the results showed that CNNs using SVM classifiers The results show that the CNN using the SVM classifier outperformed the CNN using the SoftMax classifier - see table below.
summary
This study aimed to build an automated diagnostic model for glaucoma based on fundus images. In building the model, multiple datasets consisting of 1113 retinal images were used, with approximately 70% of the dataset used for Train, 20% for Validation, and 10% for testing: 114 images for testing - 48 glaucoma, 66 normal - data were used. The performance of the models utilizing deep learning was as follows: for the SoftMax classifier, accuracy 93.86%, specificity 100%, sensitivity 85.42%, AUC 0.927; for the SVM classifier, accuracy 95.61%, sensitivity 89.58%, specificity 100%, AUC 0.948, These results suggest that it is possible to generate a classification model for glaucoma with accuracy equal to or better than that of a specialist.
One of the strengths of this study is that while it consists of a simple algorithm composed of a CNN, it is able to detect normal cases with high accuracy; the SoftMax and SVM classifiers achieve a high specificity. In addition, 89.58% of the positive cases were classified. This suggests that both glaucoma and normal subjects - especially normal subjects - can be classified with high accuracy.
On the other hand, challenges include: cost of annotation; black box nature of deep learning. In order to build models with high estimation accuracy, it is necessary to prepare large datasets of annotated - labeled - retinal images - these annotations currently require diagnosis by a medical specialist, These annotations currently require expert diagnosis and tend to have high training costs from the labeling of the images.
Possible solutions include the use of pre-training models, such as transfer learning, or the introduction of algorithms suitable for few labels, such as weakly supervised learning; the latter challenge is that the black box nature of deep learning-what criteria the model uses to make decisions The latter challenge is that the black box nature of deep learning - what criteria the model uses to make decisions - tends to be unclear. A possible solution is to utilize heat maps, such as Grad-CAM, to leverage the clarification of the model's diagnostic criteria in image always, and to give them clinical significance.
Categories related to this article