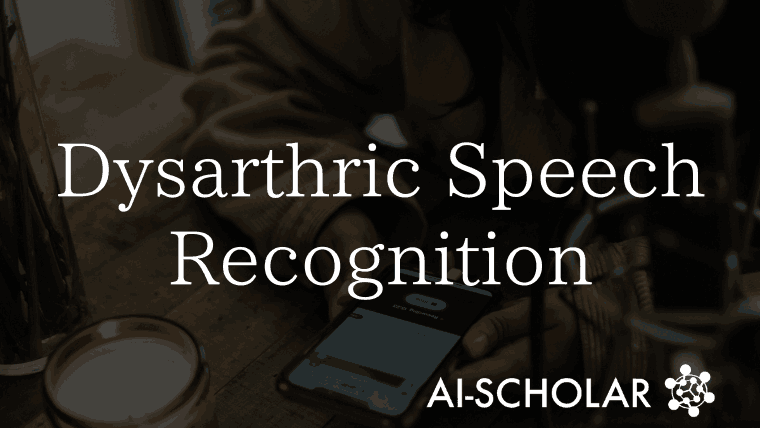
Generating Dysarthric Speech! What Is The Magic Data Extension Technology To Solve The Shortage Of Training Data?
3 main points
✔️ Comparison of different data extension techniques in fine-tuning to dysarthric speech
✔️ New model of GAN that can separate speaker and speech content characteristics
✔️ Combining different data augmentation and pre-training models to achieve a 16% word error rate
Enhancing Pre-trained ASR System Fine-tuning for Dysarthric Speech Recognition using Adversarial Data Augmentation
written by Huimeng Wang, Zengrui Jin, Mengzhe Geng, Shujie Hu, Guinan Li, Tianzi Wang, Haoning Xu, Xunying Liu
[Submitted on 1 Jan 2024]
comments:To appear at IEEE ICASSP 2024
subjects:Sound (cs.SD); Audio and Speech Processing (eess.AS)
The images used in this article are from the paper, the introductory slides, or were created based on them.
Introduction
Nice to meet you all!
I am Ogasawara, a new writer for AI-SCHILAR.
The paper presented here is this one
"Countervailing Data Extensions to Improve Fine-Tuning of Self-Supervised Learning (SSL) Pre-Learned Automatic Speech Recognition (ASR) Systems to Benign Dysphonic Speech."
It is.
As I summarized the main points at the beginning of this article, the goal seems to be to compare various data enhancement technologies to solve the chronic data shortage in dysarthric speech.
I wonder what kind of methods are being used!Let's learn together with me little by little~~!
I will try to introduce them in as concise a manner as possible, so please bear with me until the end.
Significance of this study
Before I begin to explain this study, there are a few things I would like to make you aware of.
That is why research on speech recognition for people with disabilities is difficult, because of the lack of data and the fact that pronunciation trends vary widely among speakers with the same disability.
The first is easy to understand. So many dysarthric people have dysarthria due to accidents or other acquired reasons. These people have difficulty moving and speaking in laboratory facilities and other places where the act of speaking is physically demanding.
The current situation is that data collection is not progressing well because of the following reasons.
Second, it is difficult to construct generalized models because pronunciation tendencies vary among speakers. This is another major reason why disability speech research is not generalized.
These are two of the most important reasons for the lack of progress, and there are many other problems that are difficult to solve.
Not being able to communicate satisfactorily with others is a real struggle. It also lowers self-esteem and keeps us away from social participation.
Speech research for the disabled is very important in order to help even one such dysarthric person develop a sense of self-esteem and promote their participation in society!
Proposed Method
Figures (a) through (d) above show the method proposed in this paper.
I know it is difficult to understand this all by looking at it, so let's unpack it one at a time.
The goal of this article is that after reading it, you should be able to see and understand these four models.
I'm going to chew it up and explain it to you as I mentioned earlier, so please follow me to the end!
Let's look at (a) first.
This method (a) is more of a conventional DCGAN data expansion method.
DCGAN can be simply explained by thinking of it as an upgraded model that adds a CNN-like layer to the traditional GAN model.
In this method, parallel data of normal and disabled utterances are prepared. After matching the lengths of the utterances, the generator G generates a pseudo-disabled utterance from the normal utterance, and the discriminator D is trained to distinguish between its output and the actual disabled utterance.
Let's look at (b).
This method is a model that adds speaker-dependent speed performance changes.
Even a speaker-dependent speed change is not sufficient, since each speaker speaks at a different speed.
Therefore, normal speech with adjusted speech rate for each speaker is input to the model in (a) to generate speaker-dependent pseudo-disabled speech.
Let's look at (c).
Now next, let's talk about the spectral basis GAN method.
The previous methods required parallel data. However, this method can be applied to non-parallel data.
The spectrogram of normal and disabled speech is SVD decomposed and the generator G is trained to the eigenvector U and the discriminator D to distinguish between its seed strength and actual disabled speech.
As an additional note, SVC classification is a classification task using support vector machines.
Let's look at (d)
So this model is a method using a speaker-dependent spectral basis GAN.
The method in (c) is extended to a speaker-dependent version, where the eigenvector U of normal speech with performance variation for each speaker is fed from the generator, and the result is used in the time basis to generate the final pseudo-impaired speech.
Looking at four methods
Yes, I have. I have introduced four methods so far, but have you all been following along?
A thesis looks very difficult, and in fact, it requires a very high level of knowledge to try to understand it from the text alone.
But researchers also do a lot of things to make their ideas and results well known.One of them is the figure in the paper.
If you take a closer look at a given diagram, you will learn many things. It could be a mathematical formula, a model you want to propose, or something that is difficult to understand only with the text. When you read a paper by yourself, please pay attention to the figures as well!
Do you understand? Reflection on the past
There are only three important things!
Let's just hold on to this!
- Dysarthric speech is rare and training data is lacking
- To solve this lack of training data, data expansion methods
- Goal is to improve speech recognition accuracy as well as eliminate data shortages
As long as you have these three things in mind, the rest will be fine!
In the next section, we will look at the experiment.
This is where we start! About the Experiment
Thank you very much for reading this long explanation of the basics.Next, I will finally explain the most interesting part of the paper, the experiment.
Database used
The corpus UASpeech was used in the development of this system.
A unique feature of this corpus is that it includes not only the speech of normal people, but also that of people with dysarthria.
As a side note, there are various speech databases available in Japan, including those that read out the ITA corpus, but all of them are based on the voices of healthy people, and as far as I could find, I could not find any that recorded the voices of people with disabilities.
As I mentioned at the beginning of this article, it is extremely difficult to collect the speech sounds of people with dysarthria, and even more so to create a database, but I believe that research on the speech sounds of people with disabilities will not progress without a database that is accessible to all.
I sincerely hope that database research will progress in Japan as well.
experimental setup
Now let's talk about the experimental setup. In this experiment, two SSL models (wav2vec and HuBERT) are used to evaluate the generated pseudo-disabled speech. These models have been pre-trained and further fine-tuned. For thesemodels, we demonstrate theusefulness of data augmentation by comparing them with and without data augmentation.
What are the results of the experiment?
Let's review the experimental results one model at a time, (a) through (d).
(Result of (a)
This model was a DCGAN-based model, correct? In this approach, it performed better than the speed-performance variation model shown later; an examination of the word error rate using the SSL model led to very good results, with a maximum of 9.03%. The only bottleneck is the inevitable need for parallel data.
(Result of (b)
This was a combination of speaker-dependent speed performance changes and DCGAN. For this method, the stand-alone performance evaluation was not specified in the paper, but the combination with the method in (a) showed high performance.
(Result of (c)
This model was a spectral basis GAN model, right? This method performed better than no data expansion and normal speed performance changes, but slightly less than (a).
(Result of (d)
This was the effect of the GAN learned in (c) on the performance-altered normal speech for each speaker, right? It outperformed the previous methods by a wide margin for GAN-based data expansion methods, and even combined them to derive a very good final word error rate of 16.53%.
Summary of Dissertation
Thank you all for your hard work. The presentation was a comparison of four data expansion methods. For me, the results were very interesting, as the relatively simple model using DCGAN gave better results than the more complex methods such as SVC classification.
After all, you can't understand research unless you try. It was a very good paper that gave me the feeling that I could turn my hypothesis upside down.
The results of this study can be summarized as follows
- Data expansion method is an effective method to solve the lack of data for dysarthric speech
- The DCGAN-based method not only solved the data shortage, but also improved the word error rate
The two major results are
A little chat with a chick writer, Ogasawara
Not enough money.
I know it's early in the season, but I'm talking about money, but I'm talking about research money.
I am a master's student at a national university. When I was first assigned to a laboratory, I was optimistic that information technology would not require much money as long as I had a computer. But when I started working on my research, I found myself thinking, "I want a better GPU," "I don't want to give a presentation, but I want to go to that conference to gather information," and so on.
GPUs cost hundreds of thousands of dollars, and I would like to see more research funding for master's students as well as transportation and lodging costs to go to conferences!
See you in the next article.
This is Ogasawara, a newbie chick writer~.
See you later!
Categories related to this article