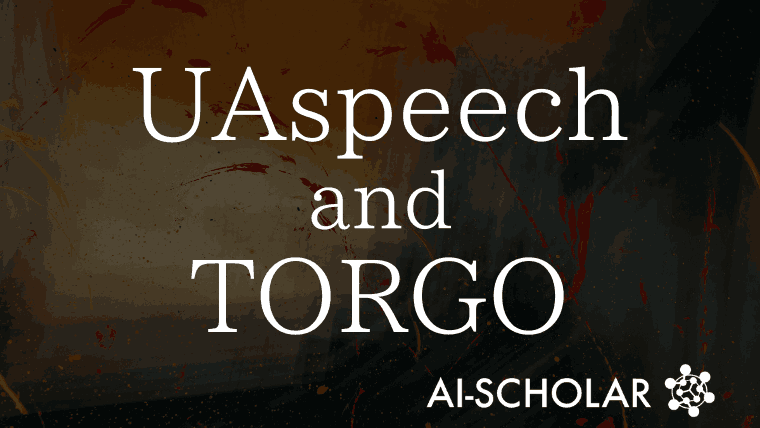
A Paper That Overturns Conventional Wisdom! The Classification Of Dysarthria Was Based On Noise, Not Characteristics!
3 main points
✔️ There are significant differences in the recording environment between the UAspeech and TORGO beta bases
✔️ Noise parts are learned more often than speech parts
✔️ Previous studies may have learned differences in recording environments rather than features of dysarthric speech
On using the UA-Speech and TORGO databases to validate automatic dysarthric speech classification approaches
written byGuilherme Schu, Parvaneh Janbakhshi, Ina Kodrasi
(Submitted on 16 Nov 2022)
Comments: Submitted to ICASSP 2023
Subjects: Audio and Speech Processing (eess.AS)
The images used in this article are from the paper, the introductory slides, or were created based on them.
Sometimes, by Questioning The Obvious, New Discoveries are Made...
Read at Least This Part! Super Summary of The Paper!
Do you know what dysarthria is? Dysarthria is a disorder in which a person understands language but is unable to speak correctly due to various factors. It is said that there are 3 million people in Japan with dysarthria, and it is one of the social problems that need to be solved.
Words are important when interacting with people. They make up a large part of our communication with others. However, because people with dysarthria have difficulty freely manipulating language, they often have difficulty communicating smoothly.
This article presents a paper on a system that automatically classifies such dysarthria. The key to this project is two datasets, UAspeech and TORGO. The two datasets have one thing in common: they both contain a large number of speech recordings of dysarthric people.They are very well-known datasets and are used in various papers in the field of dysarthria.
Now let's move on to the main issue. Dysarthria is a disorder often caused by brain damage or damage to the nervous system. A famous example is ALS. There are also dysarthria caused by congenital factors as well as acquired reasons.
The diagnosis of dysarthria was a very difficult and time-consuming process, as it was based on subjective judgment by the physician. Therefore, research and development of a system that can automatically diagnose dysarthria was conducted, and the above two data sets were widely used as evaluation indices for the system.
The question this study addresses is about the quality of these two data sets. In particular, how differences in the recording environment and recording settings between able-bodied and disabled people may affect the evaluation of the system.
The results show that there is a significant difference in SNR (the amount of noise in the speech) between the recordings of normal and disabled listeners in the two datasets. We also found that many state-of-the-art classification methods show better classification accuracy when using the non-speech (noise) part of the recording than the speech part.
In previous studies, differences in the recording environment of a data set were not considered to have a significant effect on system performance. However, the results of this study suggest that it is very likely that many systems are not actually learning features of dysarthria, but rather differences in the recording environment.
Now, here's a little additional information. Some of our readers may be wondering, " Why ? How does a different recording environment improve the accuracy of classifying it as dysarthric? And what does it mean that it learns the noisy parts? You may be thinking, "How can different recording environments improve accuracy in classifying dysarthria?
If we briefly review the characteristics of dysarthric speech, we find that their speech is slurred and irregular. What this paper points out is the "irregularity" of their speech. The muscles they use to speak are weakened, so their speech sounds very forceful to a normal person.
Therefore, it inevitably takes time before speech is produced. Therefore, the time it takes for the voice to be recorded becomes non-speech speech, or noise. The normal person's speech is smooth, so the non-speech segment is short.
In other words, the conventional system is: able-bodied = less noise.Disabled people = a lot of noise. In other words, the conventional system does not learn the unique characteristics of people with disabilities.
What are Some of The Classification Approaches...
Now let's go a little deeper into the paper here. Take a look at the diagram above. This diagram shows the approach used in this paper to classify dysarthria.
There are three main approaches taken in this paper.
- Support Vector Machine (SVM)
- CNN and SRL.
- Multilayer perceptron combined with wav2vec
SVM is a well-known classification algorithm mainly in the area of image recognition, and I am sure that everyone is familiar with CNN and multilayer perceptron, which are among the most well-known machine learning methods.
To explain a little more about wav2vec, this is a speech recognition model used mainly in the speech recognition field. One of the features of wav2vec is the use of a transformer mechanism. The transformer mechanism is a quite innovative technology, and before and after it was created, the level of speech recognition accuracy differed by one to three levels.
The major speech recognition models in use today all use this mechanism.
Well, now, what are the results of the demonstration? Let's take a look.
The Classification Results are Available at ....
Let's start with the UAspeech results.
To review again, what you wanted to show in this paper was not which classification method is better, but that dysarthric speech is classified by noise time, not by learning its characteristics.
Now, back to the diagram... Oh! It's true.
The numbers in this figure are percentages [%] of correct answers, so the higher the number, the better the result. For example, if we look at the top, SVM+openSMILE, we see that Speech is 81% and Non-speech is 84%.
Looking at the other lines of approaches, the classification accuracy is higher for Nonspeech.So, as was pointed out in the paper, noise time was used for classification, not the speech characteristics of dysarthria, which is what we originally wanted to use in the classification of dysarthria.
Next is TORGO. Overall, the accuracy of this one is lower than UAspeech's.
I have used both data sets, and overall, I felt that TORGO was not very good recording quality, with a lot of noise. This factor is also really reflected in the experimental results.
If the two data sets are tested and both are the same, then there is a very good chance that the hypothesis you wish to present in your paper is correct.
I have written this many times because it is important in this paper, but the bottom line is that it is very likely that systems that were previously thought to be designed to learn the characteristics of people with disabilities were in fact classified according to the length of noise time and other recording environment factors.
A Thesis is A Surprise Box.
This is another surprising paper. It is true that from our point of view, the feature extraction and classification flow of machine learning is like a black box.
It is only possible to make a hypothesis and show that the hypothesis is correct through experimentation. And it is even worse because, at first glance, it appears that the classification accuracy has been improved by learning the characteristics of dysarthria.
It will be necessary to study carefully what approach to take in order to learn the unique features of dysarthria and achieve a high degree of accuracy.
A Little Chat with A Chick Writer, Ogasawara
We are looking for companies and graduate students who are interested in conducting collaborative research!
His specialty is speech recognition (experimental), especially with dysarthric people.
This field has limited resources available, and there will always be a limit to what one person can tackle alone.
Who would like to join us in solving social issues using the latest technology?
Categories related to this article