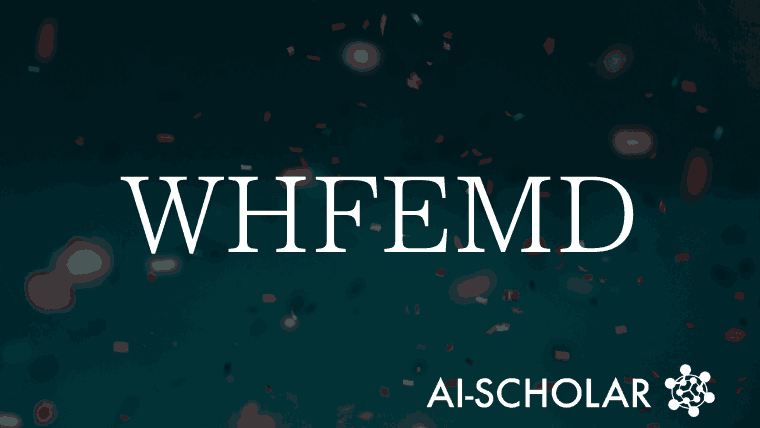
Classification Tasks - Extremely Difficult! Use The WHFEMD Algorithm To Accurately And Efficiently Capture And Classify Features Of Dysarthric Speech
3 main points
✔️ Proposal of a new feature extraction algorithm (WHFEMD) for dysarthria
✔️ Resistant to the features of slurred and unstable, which are unique to dysarthric speech
✔️ Improved accuracy in classifying severity of dysarthria compared to previous algorithms
Enhancing dysarthria speech feature representation with empirical mode decomposition and Walsh-Hadamard transform
written by Ting Zhu, Shufei Duan, Camille Dingam, Huizhi Liang, Wei Zhang
(Submitted on 30 Dec 2023)
Comments: Published on arxiv.
Subjects: Audio and Speech Processing (eess.AS); Artificial Intelligence (cs.AI); Signal Processing (eess.SP)
code:
The images used in this article are from the paper, the introductory slides, or were created based on them.
To Accurately and Efficiently Capture The Characteristics of Dysarthric Speech...
Read at Least This Part! Super Summary of The Paper!
In today's world, it is really convenient to do household chores and work by voice control of anything, including smartphones and smart home appliances.
This convenience is due to the development of artificial intelligence, which has greatly improved the speech recognition rate. Now, do you know what dysarthria is? Well, if you are reading this article, you are probably familiar with it to some extent, but for those of you who don't know anything about it, let me explain a little bit about it.
Dysarthria is a disorder in which a person understands language but is unable to pronounce it correctly due to abnormalities in the nervous system. The most famous examples of this disorder are those with cerebral palsy, such as ALS, but there are also congenital disorders caused by the nose and other factors.
Although it is not limited to the modern age, I think that voice information is extremely important in communicating with others. However, although people with dysarthria try to devise ways to communicate in their own way, many of them are unable to communicate smoothly with others and are uncomfortable interacting with others.
In this issue, we will introduce a paper that attempts to help those with such dysarthria.
As I mentioned at the beginning, voice operation of electronic devices is convenient - even those who are not good with machines can operate them with ease, making it a truly user-friendly technology. However, people with dysarthria, who have voice disorders, cannot enjoy this convenience.
This is because existing systems are designed for use by normal people and do not incorporate technology to accurately process dysarthric speech.
Therefore, this study focuses on the characteristics of slurred and unstable dysarthric speech and proposes an efficient method to capture it.
Taking it a bit further, the project aims to accurately capture the complex features of speech in dysarthric people and use them to classify the severity of the disorder, which will be useful for medical diagnosis and treatment planning.
The algorithm proposed in the paper is called WHFEMD. This algorithm can classify the severity of dysarthria with higher accuracy than conventional methods.
In previous studies, dysarthric speech was generally analyzed using acoustic features such as MFCC and LPC, which could not adequately capture their speech features. The algorithm in this study was able to accurately capture their complex speech features, resulting in improved classification accuracy.
Alright, here's a quick summary of the paper. Until now, classification of severity of illness has been done by physicians and speech-language pathologists because the conventional method lacks accuracy. However, human judgment is subjective and lacks objectivity, and above all, the burden on those who make the judgment must be considerable.
Are you curious about today's main dish, WHFEMD, what kind of algorithm and what kind of specific results were obtained?
In the next section, we will go into a more in-depth explanation! Please stay with us to the end if you are interested.
What is The Architecture of WHFEMD...
In the following figure, we have a conceptual diagram of the proposed algorithm. Here is a conceptual diagram of the proposed algorithm! It's full of abbreviations that I don't know... I don't know.
Don't worry, I'll break it down one by one and explain it as clearly as I can!
The first thing the audio goes through is the FFT, which is a signal processing called Fast Fourier Transform that transforms the audio signal into the frequency domain,
Next is EMD, which is empirical mode decomposition, which decomposes the signal into multiple intrinsic mode functions (IMFs). This allows us to capture opaque and unstable features of the signal.
To explain IMF in a very simplified manner, it is the breaking up of a complex signal into a collection of simple waveforms. To use a musical analogy, it is like taking an orchestral ensemble and breaking it down into instrumental performances.
FWHT is a process called Fast Walsh Adamar Transform, but since the theory is quite complicated, it is sufficient to just remember that it is also used for feature extraction.
Once feature extraction is complete. It is used for the dysarthria classification task and labels (degree of symptoms) are output for the input speech.
Now let's look at the performance evaluation of the method!
Can We Compete with Cutting-Edge mMethods? ....
Two corpora of dysarthric speech, UAspeech and TORGO, were used to evaluate the method.
Both are well-known corpora, so those interested in the field of dysarthric speech should keep them in mind. In comparison, TORGO has a small number of readings per speaker, and the recording quality is not flattering due to the noisy audio.
Well, this is inevitable since they were made in different eras.
Now, as a result, the performance was compared with the state-of-the-art classification task. The results showed that the proposed method performed as well as the state-of-the-art method!
I believe that the attention paid to the unique characteristics of dysarthric speech and the selection of a mechanism to match these characteristics may have contributed to this performance.
Researchers also Have A Personal Color Scheme...
Well, we have looked at classification task methods for dysarthric speech. I have read more than a dozen papers in the six months since I started this writer's work, and as I said, in the field of dysarthria, there is a lot of research on classification tasks.
I guess that's how much the technology is needed in the field. Personally, I'd like to see more progress made in voice recognition.
Even in the same classification task, each researcher has his/her own color, and so much thought has been put into the task that it would be a waste to make judgments based solely on performance.
Some of the papers I have read have also caused a stir in the way classification tasks are being studied today.
Future research trends in the field of dysarthric speech will also be closely watched!
I guess that's all for this issue. See you in the next article~.
A Little Chat with A Chick Writer, Ogasawara
We are looking for companies and graduate students who are interested in conducting collaborative research!
His specialty is speech recognition (experimental), especially with dysarthric people.
This field has limited resources available, and there will always be a limit to what one person can tackle alone.
Who would like to join us in solving social issues using the latest technology?
Categories related to this article