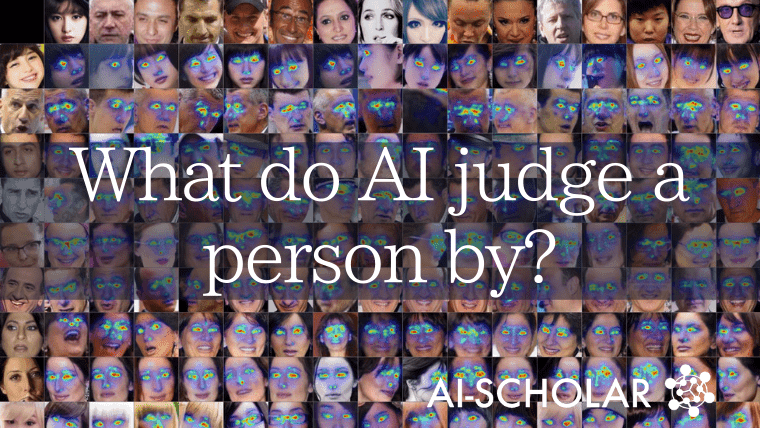
Explainable Face Recognition (XFR) On What Basis Did The Face Recognition Model Identify A Person?
3 main points
✔️ Provides a baseline for Explainable Face Recognition (XFR) to visualize the recognition basis of facial recognition models
✔️ Proposes a comprehensive methodology (Inpainting Game) and indicators for a quantitative and detailed evaluation of XFR, and provides a dataset
✔️ Two newly proposed algorithms (Subtree EBP and DISE) achieve higher performance than before
Explainable Face Recognition
written by Jonathan R. Williford, Brandon B. May, Jeffrey Byrne
(Submitted on 3 Aug 2020)
Comments: Accepted at ECCV2020
Subjects: Computer Vision and Pattern Recognition (cs.CV)
What is Explainable Face Recognition (XFR)?
Facial recognition has a task called 1:N (Identification). This task compares one person's photo with N registered photos and finds the person with the highest degree of similarity.
This task is used in criminal investigations and entry/exit points. In criminal investigations, the task detects faces from security cameras and compares them with a database of criminals in order to identify criminals more efficiently. In addition, when entering and exiting a building, the face is photographed at the entrance gate and checked against the employee database to enable hands-free and walk-through entry.
In recent years, breakthroughs in deep learning have greatly improved the accuracy of facial recognition, which has made it practical. However, on the other hand, there is a certain amount of risk involved in trusting the recognition results of the model because the model has become more complex and the criteria for making decisions is a black box.
Why did the face recognition model decide that the image was the most similar to other images? What is the basis for this?
Explainable Face Recognition (XFR) is the model that visualizes this rationale. Visualizing the decision-making basis for a model is one of the key elements in the safe use of face recognition technology.
In this paper, we propose a method to more accurately detect and evaluate this decision basis. The figure below is an overview of this paper. First, {Mate #1, Probe, Non-mate (Inpainted)} is entered into XFR as a pair. Next, calculate the pixels with the largest difference in probability in XFR with {Probe, Mate #1} and {Probe, Non-mate (Inpainted)} to create the XFR Saliency Map. Finally, the match between the XFR Saliency Map and Non-mate (Inpainted) (Mate #2) is calculated in pixels. The less the value of Red, the more the value of Green, the higher the value of Green, the more accurately the XFR Saliency Map recognizes subtle differences between the two, and the more reliable the model is.
This article will introduce the XFR algorithms (Subtree EBP, DISE), methods for quantifying performance (Inpainting Game), and the results of those evaluations.
To read more,
Please register with AI-SCHOLAR.
ORCategories related to this article