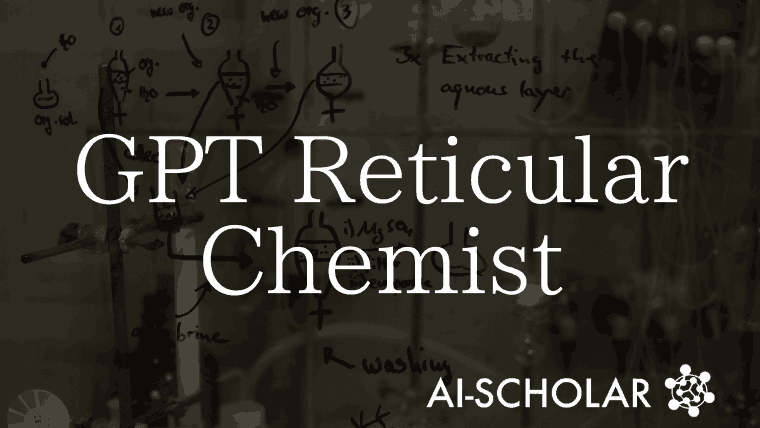
Potential For New Materials Development By GPT-4 In Reticular Structure Chemistry
3 main points
✔️ suggest that GPT-4 is useful for developing new materials in reticular structural chemistry, where knowledge of multiple sciences, including chemistry and physics, is essential.
✔️ New framework using GPT-4 proposed, working with researchers to accomplish tasks from experimental design to results analysis
✔️Systematically manages the research process in reticular structure chemistry,increasing research efficiency andproviding methods that can be applied by researchers inother fields
A GPT-4 Reticular Chemist for Guiding MOF Discovery
written by Zhiling Zheng, Zichao Rong, Nakul Rampal, Christian Borgs, Jennifer T. Chayes, Omar M. Yaghi
(Submitted on 20 Jun 2023 (v1), last revised 4 Oct 2023 (this version, v2))
Comments: 173 pages (9-page manuscript and 164 pages of supporting information) Submitted to Angewandte Chemie International Edition
Subjects: Artificial Intelligence (cs.AI); Materials Science (cond-mat.mtrl-sci); Chemical Physics (physics.chem-ph)
code:
The images used in this article are from the paper, the introductory slides, or were created based on them.
Summary
Reticular Chemistry (Reticular Chemistry) has become essential in the development of new materials and innovative strategies to exploit their properties. This fieldrequires extensive knowledge ofmultiple areas of chemistry (organic, inorganic, physical, analytical, and computationalchemistry) as well as physics, biology, materials science, and engineering. However, it takes an enormous amount of time, education, and training to develop reticular structural chemistry experts with such extensive knowledge. Therefore, the help of artificial intelligence (AI), especially GPT-4, a large-scale language model (LLM), is expected to be a solution to efficiently and quickly bridge the knowledge gap between different disciplines.
This paper demonstrates how GPT-4 can be integrated into a chemist's daily tasks and workflow in the form of reticular structural chemistry experimental design, review and summarization of research articles, educational introduction to various concepts and techniques, and assistance with data interpretation. GPT-4, with its excellent language comprehension, can assist with a variety of tasks through the use of carefully designed prompts that contain clear instructions. However, experimentation (observation, an iterative trial-and-error process based on a hypothesis) cannot be performed by GPT-4 alone.
Therefore, this paper proposes a new framework that integrates GPT-4 into the iterative process of chemical experiments and collaborates with researchers at all levels of expertise, with GPT-4 providing detailed procedures for the experiments and researchers performing them and providing detailed feedback on their observations and results, GPT-4 and the human researcher work together to learn and dynamically adjust their strategies to achieve the project's goals. This innovative approach is expected to be reciprocal, with GPT-4 learning from experimental results and humans accelerating their research under GPT-4's expert guidance.
This initiative not only increases the feasibility and efficiency of research activities in the field of reticular structural chemistry, but also holds great potential for scientific research in general.
Methods and Experiments
In this paper, we develop a unique framework that leverages human feedback and utilizes GPT-4 to speed the discovery of new metal-organic frameworks (MOFs). This new approach has led to the discovery of four new MOFs with specific chemical formulas through human and AI collaboration. Notably, everything from the design, synthesis, optimization, and characterization of these MOFs was done by GPT-4 and executed by human researchers. This innovative framework leverages prompt engineering and in-context learning to immediately applylarge-scale language models such as GPT-4 to reticular structural chemistry. The process is divided into three phases, with GPT-4acting as the Reticular Chemistry chemist in each phase, interacting with humans through customized prompts. These stages are named "Reticular ChemScope," "Reticular ChemNavigator," and "Reticular ChemExecutor" and each has its own purpose and supports the progress of the project. An overview is shown in the figure below.
First, in the "Reticular ChemScope" phase, GPT-4draws a blueprint of the projectbased on the information provided. Next, in the "Reticular ChemNavigator" phase, GPT-4 proposes specific tasks and sets the direction of the project based on human feedback. Finally, in the "Reticular ChemExecutor" phase, GPT-4 provides the details of the tasks to be performed and the framework for feedback, and the project proceeds based on the results.
This three-step process facilitates an organic dialogue between the human researcher and GPT-4 through the elaboration of prompts. The human researcher shares knowledge gained from previous experiments, and GPT-4 uses this knowledge as a "memory" to make optimal recommendations based on the current status of the project. This thorough interaction allows the best decisions to be made at each stage, and the project progresses efficiently toward its goals.
In particular, suggestions from GPT-4 provide valuable guidance in designing experiments, taking into account available resources and the level of expertise of the researcher. Throughout the entire process, GPT-4 not only provides direction, but also helps the human researcher document the results of the experiment and plan next steps based on them.
In this paper, we challenge you to discover new metal-organic frameworks (MOFs). Specifically, we focus on the creation of four new MOFs (MOF-521-H, -oF, -mF, and -CH3) as shown below.
Interestingly, MOF-521-H was an undisclosed compound that had been previously discovered incidentally, but GPT-4 took a unique approach to formulate a strategy for these MOFs without being distracted by existing knowledge.
The experiment begins with a thorough literature review, guided by Reticular ChemNavigator, to explore the synthetic route of the linker. We then proceed through the organic synthesis reaction to confirm the linker and identify the optimal conditions for MOF formation. During this phase, various experimental conditions (metal-to-linker ratios, temperatures, reaction times, modifying agents and their ratios, etc.) are adjusted, and microscopic observations and powder X-ray diffraction (PXRD) are used to report progress.
Of particular note is that throughout the process, GPT-4, in collaboration with human researchers, provides detailed guidance in the design and execution of the experiments, as well as in the interpretation of the results. As a result, after several optimizations suggested by Reticular ChemNavigator, single crystals of all compounds were obtainedand their structures were characterized by SXRD.
Through single crystal X-ray diffraction analysis of the MOF-521 series, we have discovered that these compounds crystallize in the P-62c (No. 190) space group with nearly identical unit cell parameters. These compounds are composed of unique rod-shaped secondary building units (SBUs), the details of which show how the AlO6 octahedra are linked together in a specific manner and further bound by BTB linkers and formates. This results in the formation of one-dimensional linear rod-like structures. The structures of these compounds also show that the carboxylates from the BTB linker lie almost in the same plane, despite the positional disorder in the surrounding phenyl rings.
In addition, this study delves deeply into the topology of the MOF-521 series, suggesting that this may be a new type of MOF not previously reported. Specific analytical methods were applied to the topological analysis, along with standard methods. This confirmed that these compounds exhibit a peculiar rod packing, which is a new structure for MOFs not previously reported. Interestingly, the presence of closely packed rod-shaped SBUs serves as a special SBU with inaccessible internal space, which is a unique structural feature of MOF-521.
This analysis was performed by humans due to its mathematical and analytical nature, which is beyond the capabilities of GPT-4, but ultimately shows that MOF-521 has a unique topology.
Through our work with GPT-4, we have also made the important discovery that all four MOF-521 compounds have the same topology. There is a remarkable agreement between the simulated powder X-ray diffraction (PXRD) patterns and the actual experimental data. This progress has led us to the third step, the verification of permanent porosity.
Guided by Reticular ChemNavigator, the porosity of the MOF-521 family is confirmed using nitrogen adsorption measurements. Surprisingly, the experimental results are consistent with the values predicted a priori in Material Studio. The Type I isotherms exhibited by each compound show no significant hysteresis, indicating a constant trend between porosity and the size of the functional groups in the framework.
In particular, MOF-521-H, the least substituted, recorded the highest BET surface area of 1696 m2g-1, while MOF-521-oF and MOF-521-mF also showed comparable surface areas of 1535m2g-1and 1562m2g-1, respectively. possible surface areas. In contrast, MOF-521-CH3 shows a surface area of1311m2g-1, significantly lower than the other compounds. These results suggest that substitution of the central benzene has a significant impact on the porous environment and can be used as a potential means of modulating it.
Proton NMR spectroscopy and elemental analysis were subsequently performed to confirm the composition and chemical formula of each compound. These analyses suggest that the MOF-521 compound is fully active. In addition, data analysis was conducted primarily by humans based on recommendations from GPT-4, and this interaction reflects a strategic approach designed to minimize the potential hallucinogenic effects of AI in scientific research.
Importantly, the number of prompt iterations was consistent throughout the project, which served as a measure of the efficiency and complexity of the study; the evolving in-context learning ability of GPT-4 was through fewer iterations with subsequent compounds than with the first compound, Further evidence.
This process represents a powerful example of the symbiotic relationship between humans and AI in scientific research: the GPT-4 was able to provide valuable guidance, similar to the insights and instructions that an experienced chemist might provide. It demonstrates the emerging role of AI in the scientific community and helps pave the way for new discoveries and developments, especially in the field of reticular chemistry.
Summary
This paperinnovates on traditional methods of chemical experimentation by incorporating a large-scale language model, GPT-4, to improve the efficiency of experiments, particularly in Reticular Chemistry (Reticular Chemistry). Focusing on symbiotic human-AI collaboration, we are developing a framework for efficient synthesis and analysis of novel MOFs (metal-organic frameworks). Even in areas where synthetic strategies and optimal conditions are similar, GPT-4 insights allow for more precise coordination and interpretation of results.
The methodology demonstrates how effective the GPT-4 guides are in the various phases of scientific research and shows the potential of the model to be scalable and applicable across scientific disciplines. It also provides a more manageable and systematic approach by dividing the research process into three phases, paving the way for full automation and robotization of experimental procedures. This progressive learning capability is also considered useful for researchers in different fields.
Categories related to this article