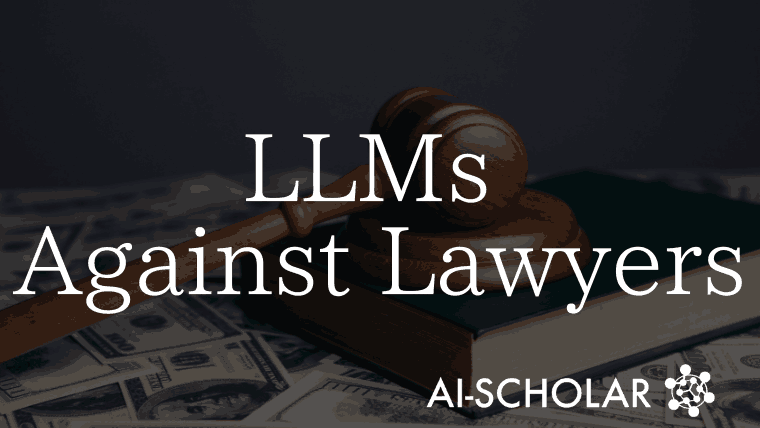
Transforming Legal Services With Large-Scale Language Models! Surpassing Humans In Speed And Accuracy?
3 main points
✔️ Large-scale language models achieve performance equal to or better than junior attorneys and outsourced legal practitioners
✔️ Large-scale language models can complete tasks faster and at lower cost than practitioners in contract review
✔️ Future work to evaluate performance in complex scenarios beyond contract documents, such as different contract types and contract negotiations
Better Call GPT, Comparing Large Language Models Against Lawyers
written by Lauren Martin, Nick Whitehouse, Stephanie Yiu, Lizzie Catterson, Rivindu Perera (Onit AI Centre of Excellence)
(Submitted on 24 Jan 2024)
Comments: Published on arxiv.
Subjects: Computers and Society (cs.CY); Computation and Language (cs.CL)
code:
The images used in this article are from the paper, the introductory slides, or were created based on them.
Summary
The advance of artificial intelligence (AI) in the legal industry is creating new possibilities for legal services. However, research on the use of generative AI and large-scale language models (LLMs) in solving and discovering legal problems still leaves much room for exploration. In particular, it is critical to understand how these advanced technologies work in accurately classifying and identifying legal problems that rely on the deep knowledge and expertise that human legal professionals have accumulated over the years.
To fill this gap, this paper is an experimental and exploratory study that delves deeply into the capabilities of large-scale language models in the legal field. The paper assesses how large-scale language models compare to human legal practitioners, particularly junior attorneys and outsourced legal practitioners, in actual legal work. Given the rapid development of large-scale language models, it provides deep insights into how effectively these technologies work in existing legal practices and how they may surpass human experts in expertise and efficiency.
This paper focuses on three questions in particular
- Are large language models better than junior attorneys and outsourced legal practitioners in their ability to identify and locate legal issues in contracts?
- Can large language models review contracts more quickly than junior attorneys or outsourced legal practitioners?
- Can large language models review contracts more cost-effectively than junior attorneys or outsourced legal practitioners?
Through this research, we aim to develop a comprehensive understanding of the potential capabilities and limitations of large-scale language models in the legal field and provide valuable insights for legal and AI professionals.
Technique
The papercompares the performance of the large-scale language modelwith the work ofjunior attorneys andoutsourcedlegal practitioners (LPOs). Senior attorneys are established as the basis for comparison, and their ability to identify and locate legal issues in contract documents is tested. This approach aims to replicate the process that real attorneys go through when reviewing contracts. In addition , we adhere to strict ethical standards set forth by Onit Inc. in the collection and analysis of data, as well as in the involvement of participants. Participants are informed in advance and in detail about the purpose of the study, how the data will be used, and their right to withdraw from participation at any time. In addition, personally identifiable information is removed from the data and the anonymity of participants is protected. The contract data used are also anonymized during the process and de-identified to allow for further detailed analysis, thus strictly protecting the privacy of the data. Ethical oversight and compliance are established through the Ethics Committee to ensure that research activities comply with data protection and privacy laws and regulations. This includes auditing research processes and verifying legal compliance to ensure that research is conducted under high ethical standards.
In addition, 10 procurement contracts selected from actual legal contracts were used as data sources. All of these have been anonymized to protect their confidentiality. Procurement contracts are the type of contracts that legal practitioners frequently work with and were selected based on their prevalence with confidentiality agreements. In selecting the contracts, consideration was given to ensure a balanced representation of different legal systems, such as the United States (US) and New Zealand (NZ). With this approach, we aim to make the results of the study applicable to a wider body of law.
Senior attorneys are also responsible for evaluating the extent to which contracts comply with defined standards and establishing baseline data. They determine whether the contract complies or deviates from the defined standards and identify the specific sections of the contract that are the reason for the deviation. They are also required to explicitly record any missing required information from the contract. These data are aggregated and serve as the basis for forming benchmarks against each of the evaluation criteria.
In addition, the average time required for contract review was also recorded and used as a basis for comparing the time typically taken by legal practitioners to review contracts with the time taken by junior attorneys, LPOs, and large-scale language models. In this way, the process from data collection to analysis is intended to enhance the credibility and transparency of the study.
As for setting attorney hourly rates and large language model costs, attorney hourly rates are based on in-house attorney rates determined by industry benchmarking reports, such as ACC's 2023 Legal Department Compensation Survey, and outside attorney rates determined by market data maintained by Onit Inc. Onit Inc. Costs for the large language model are determined through commercial pricing offered by service providers.
In addition, the paper considers multiple factors in selecting a model for a large-scale language model. These include preliminary test results testing the applicability and effectiveness of the model in the legal domain, as well as the limitations of the model's context window. In particular, we scrutinize the performance and applicability of models developed by leading companies such as OpenAI, Google, Anthropic, Amazon, and Meta.
Preliminary testing has examined how these models process and analyze sample contract documents. The analysis focused on how accurately the models identified and located the legal issues and the extent of their reasoning ability. Emphasis was also placed on identifying the optimal context window size to address the research questions, and selecting models that could handle the contextual information needed to understand the contract documents as a whole.
The analysis in this paper also reveals that the size of the context window has a direct impact on the performance of the model: models with context windows of less than 16,000 tokens, such as LLaMA2 and Amazon Titan, require the document to be split into multiple parts, found this to be inefficient. Such splitting compromised the ability to analyze the entire contract. Therefore, we have narrowed our focus to models with large context windows and have established criteria to representatively assess a model's ability to analyze legal documents.
This approach allows us to explore in depth the applicability and effectiveness of large-scale language models in legal analysis. By deepening our understanding of how these models work in the legal domain, we aim to provide insights that will be useful in advancing future research and practice.
In addition, prompt engineering is essential for the Large Language Model to complete the contract review task efficiently and accurately. This process involves having the LLM adopt a specific role and tasking them with evaluating the contract according to a defined standard. Specifically, the prompt consists of three main elements: role, task, and context.
- Role: Large-scale language models are instructed to adopt the role of a lawyer when performing tasks.
- Task: Large-scale language models are tasked with determining whether a contract follows or deviates from a defined standard and locating the problem.
- Context: mimics the instructions typically provided to attorneys, LPOs, or contract reviewers in large language models, including the target audience for the contract, background information about the contracting parties, and the specific scenario under which the contract was negotiated.
In this paper, we use these elements to help large-scale languagemodels replicate the work of attorneys in practice and to improve their understanding of the context in which they review contract documents. We alsocarefully consider how the contextual elements should be designed to achieve optimal results for each task that the large-scale languagemodel perform s. A specific example of prompt engineering is shown in the figure below.
Experiments and Results
This articleuses Cronbach's alpha to analyze the degree of agreement among three groups ofsenior attorneys, junior attorneys, andoutsourcedlegal practitioners (LPOs) to explore the applicability of the large-scale language model in the legal domain. The results of the degree of agreement are shown in the figure below.
The analysis reveals a very high degree of agreement among the participants as a whole, with a very strong alpha value of 0.923366. However, the level of agreement among senior attorneys only is the lowest at 0.719308, suggesting a more diverse approach to identifying issues within the contract among experienced practitioners. On the other hand, junior attorneys show a slightly higher degree of agreement with an alpha value of 0.765058, which may reflect more consistent training methods and adherence to the existing legal framework.
We also evaluate the accuracy of different large-scale language models in comparison tojunior attorneys andoutsourced legal practitioners (LPOs). This comparative evaluation is based on the judgment of senior attorneys as the baseline data. In particular, GPT4-1106 and LPO practitioners perform best in identifying legal issues with an F-score of 0.87. This indicates the high accuracy and reliability of these groups in identifying legal issues. Junior attorneys, on the other hand, performed slightly below, achieving an F-score of 0.86. These results indicate that the large language model outperforms both junior attorneys and LPOs in time efficiency in the task of reviewing legal contracts.
An analysis of time efficiency during the review of legal contracts was also conducted. This analysis showed that senior attorneys were the most efficient, but the large language models were notably more time efficient. In particular, GPT-1106 took the longest processing time, while Palm2 text-bison took the least time. This result indicates that the large language model is much more time efficient than junior attorneys and LPOs in the task of reviewing legal contracts.
In addition, a detailed cost comparison between attorneys, LPO practitioners, and LLMs is provided. This comparison is important for understanding the economic impact of introducing LLMs into the legal domain, particularly in tasks involving the identification and positioning of legal issues within a contract. Compared to the costs incurred by human practitioners, LLMs have been shown to have a significantly lower cost per document. This cost-effectiveness is a powerful incentive for expanding the use of LLMs in the legal field.
Particularly noteworthy is that agreement among LPO practitioners reached a perfect alpha of 1, with absolute agreement in their responses. These results provide valuable insight into how large-scale language models can complement and enhance the diversity of approaches and agreement among practitioners in the analysis of legal documents.
Summary
The papershows that large-scale language modelscan accurately identify legal issues within a contract as well asoutsourcedlegal practitioners (LPOs)and junior attorneys. Of note isthe speed oflarge language models in contract review. Their computational efficiency gives them the remarkable advantage of being able to process and analyze text faster than human practitioners. This speed has the potential to dramatically improve contract review productivity and response time. In addition, cost-related analysesconfirm thatlarge-scale languagemodels offer a much lower-cost alternative to contract review compared to junior attorneys and LPOs. High accuracy, fast processing speed, and low cost make large language models an attractive option for legal practitioners and law firms looking to streamline the contract review process.
However, based on the points identified from the study, further in-depth exploration is needed. In particular, extensive evaluation of the performance of large-scale language models through different contract types and the enrichment of the reference data set is required. We are also focusing on exploring the potential capabilities of large-scale language models in the area of contract negotiation, which requires understanding complex contexts beyond the text of the contract document.
It is hoped that these future studies will help to capture the full potential of large-scale language models in the legal industry and to move beyond the limitations found in the current study.
Categories related to this article