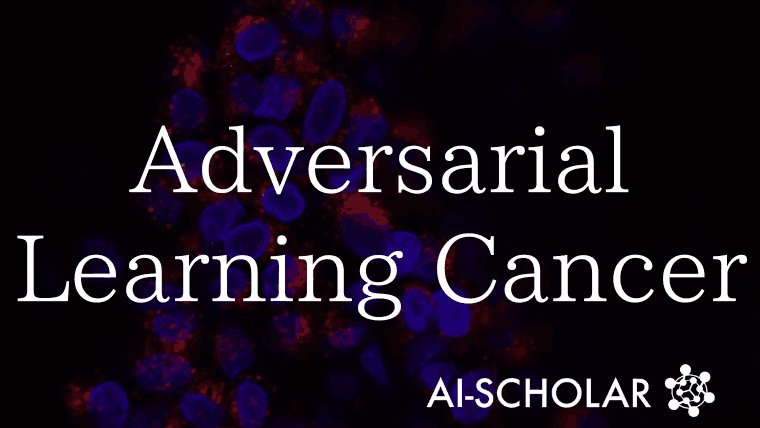
A Method For Predicting Cancer Drug Candidates Based On Hostile Domain Adaptation Is Proposed!
3 main points
✔️ New model proposed to estimate drug candidates associated with suppression of cancer stem cell function
✔️ Uses adversarial domain adaptation techniques to learn while eliminating bias in two different data sets
✔️ Drug candidates predicted by the model are validated by experiments using real cells Confirmation
The images used in this article are from the paper, the introductory slides, or were created based on them.
Introduction
Research Background and Key Points of the Method
Cancer, a disease that is expected to affect one out of every two Japanese people in the future, is one of the most intractable diseases for which no cure has been established in the modern era. Cancer is known to occur and progress when the mechanism for cell growth in animals, including humans, breaks down and the cells become specialized cellscalled cancer cells.
One of the most specialized types of cancer cells are cancer stem cells. Stem cells are specialized cells that have the ability to replicate themselves or change into cells with a wide variety of functions (this is called differentiation). Cancer stem cells combine the properties of a cancer cell, in which cell regulation is broken down, with the properties of a stem cell.
These cancer stem cells are known to have a significant impact on cancer metastasis andrecurrence. In particular, in recent years, drugs have been developed to induce cancer stem cells to change the way they differentiate and suppress cancer progression as a treatment for cancer.
This paper describes the development of a new model to identify new drug candidates using a technique called adversarial domain adaptation, with a particular focus on breast cancer among other cancers.
Model Structure
For hostile domain adaptation used in this model![]()
Study Workflow
Overall Model
About the Data Set
The source domain dataset is a dataset that systematically compiles information on human induced pluripotent stem cells (stem cells derived from adult somatic cells, abbreviated as hiPSCs ), which are analyzed using scRNA-seq, a technique to determine the function of genes derived from these cells.
The target domain dataset (LINCS L1000), on the other hand, is a large scale analysis and systematic compilation of how cells respond to all stimulibased on how genes affect their function.
The latter is a dataset that specifically details how a particular drug affectsfunction, e.g., how giving drug X (or Y) to cell A (or B) will ultimately affect its function, as shown in the figure. The data set shows in detail how the final function of cells A (or B) is affected by drug X (or Y).
In this study, domain adaptation is used to first learn the pattern of cell differentiation from the source domain and then use that knowledge to predict the ability of each drug to induce differentiation.
Diagram of Learning Progression
This figure shows how learning progress es, with the horizontal axis representing epochs in learning and the vertical axis representing losses. Changes in loss for a task classifier in the source domain are shown in blue, changes in accuracy for that classifier are shown in light gray, changes inaccuracy for the source domain in an adversarial classifier are shown in gray, and changes in accuracy for the target domain are shown in dark gray.
The accuracy of the task classifier (light gray) reaches 86.7% accuracy. As for the accuracy of the adversarial classifier, since the goal is to minimize as much as possible the ability to distinguish between the two types of data sets, the accuracy using the source domain (gray) and the accuracy using the target domain (dark gray) are expected to approach 50% each, and the figure certainly converges to that value The figure shows that they do indeed converge to that value.
Comparison before and after Domain Adaptation
In Figure c above, we show the results of the tSNE analysis before (left panel) and after (right panel) domain adaptation. Blue, green, yellow, and red show the distribution obtained by clustering thesource domaininto four, while black shows the distribution obtained from the data set used in the target domain.
While cells at different stages of differentiation are widely dispersed before domain adaptation, after domain adaptation they are more distinguishable, and furthermore, the distribution of target domains is spread throughout the distribution of source domains.
Experimental Results
This figureshows the results of chemical experiments performed on the drug candidates predicted by the model.
First, Figure a shows the DECODE scores in red for the top 10 drug candidates predicted by this model, indicating their characteristics as stem cells, and the scores in blue for the bottom 10 drug candidates.
The higher the DECODE score, the stronger the stem cell characteristics, and this figure predicts that higher priority drug candidates will have higher stem cell characteristics.
In Figure b, we show the ratio of values before and after drug treatment for three indicators: the number of stem cell populations, the total area formed by the populations, and their average. This shows that the use of the drug candidates predicted by the model makes a difference in various indicators related to the properties of the stem cells.
In addition, in the paper, the high priority drug candidates can be evaluated for their effects on breast cancer stem cells, showing that they inhibit the growth and self-renewal capacity of these cells. In other words, it is confirmed that each drug is highly effective.
Figures c through e are graphs showing the percentage of the 30 drug candidates predicted by the model to inhibit or promote processes related to cell function and cell structure. Inhibition of function is shown in red and promotion of function is shown in green.
These figures show that the drug candidates predicted by the model suppress the function of genes related to the cell cycle, but promote cell differentiation. In other words, the drug candidates are able to regulate the properties of cancer stem cells.
Figures a through c above illustrate how the model's predicted agents affect the ability of breast cancer stem cells to grow and replicate themselves.
For Figure a, the effect of the drug is visually represented.The top and bottom rows show representative examples of cancer stem cells used in the experiment. The leftmost of the five columns showshow much the stem cell characteristics are affected bygradually increasing concentrations of the drug"TRIPROLIDE" in the second and third columns from the left and "OTS-167" in the fourth and fifth columns,respectively,obtained by the model predictionsThe following table shows the results of the model.
The more we increase the concentration of the drug, the more we read that the percentage of clumps is decreasing (i.e., that the drug is suppressing the function of cancer stem cells or, in other words, that the drug is effective as a treatment ).
Figure b is a further quantification of this, showing how the properties of one cancer stem cell on the left half and another stem cell on the right half differ when three different drugs are used (you can safely assume that the horizontal axis in each figure represents the concentration of the drug and the vertical axis the strength of the cancer stem cell's properties ).(You can safely assume that the horizontal axis of each figure represents the drug concentration and the vertical axis represents the strength of the cancer stem cell characteristics .)
The case where no drug was added is shown inblue, the case where a small amount was added is shown in red, and the case where a large amount was added is shown in green. The figure confirms that the green color tends to score lower on the vertical axis than blue or red (i.e., that the cancer stem cell function can be inhibited, or in other words, that the drug predicted by the machine learning model is properly effective as a treatment ).
Summary
Categories related to this article