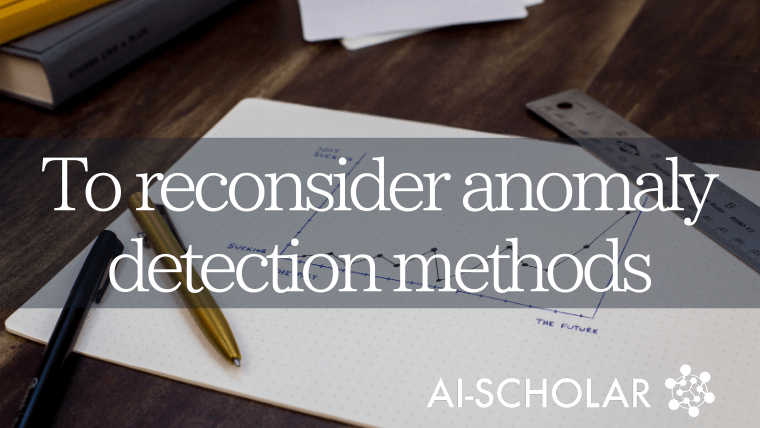
Supervised Learning With A Small Sample Of Anomalies Is Sufficient For Anomaly Detection! Rethinking Traditional Anomaly Detection Methods!
3 main points
✔️ Reconsider the anomaly detection method of training with normal data only
✔️ Supervised learning of a small number of abnormal and normal samples (binary classification task) to achieve SOTA
✔️ If the resolution of the anomaly sample is high, the method using the anomaly sample is effective
Rethinking Assumptions in Deep Anomaly Detection
written by Mingyi Zhou, Jing Wu, Yipeng Liu, Shuaicheng Liu, Ce Zhu
Comments: 22 pages, preprint
Subjects:Machine Learning (cs.LG); Machine Learning (stat.ML)
Introduction
Anomaly detection refers to the task of detecting abnormal data that is different from normal data and is a very widely used technology. For example, it is often used to detect defective products in the manufacturing industry, and will become increasingly important in security applications such as detecting suspicious behavior and objects.
Anomaly detection can be thought of as a binary classification task that classifies normal data and abnormal data. However, unlike general binary classification, anomaly detection is often approached by learning anomaly detection models with normal samples only, because anomaly samples are difficult to obtain in anomaly detection. However, even though anomaly data is hard to obtain, it doesn't mean that it is impossible to obtain the data at all, and it is generally expected that a small number of samples can be obtained. In this case, is the best approach to train a model using only the current normal data? In other words, is an approach that uses a small number of anomalous data neglected?
This paper will let us reconsider the current approach to anomaly detection. We have conducted experiments and measured the accuracy of each method with an approach that allows training with normal data only, an approach that considers anomalous data as supplementary data, and a simple binary classification task. The results show that SOTA can be achieved by solving as a simple binary classification task in situations where small amounts of anomalous data are available. In the following, we will introduce the conventional method, the proposed method, and the experiments we conducted.
To read more,
Please register with AI-SCHOLAR.
ORCategories related to this article