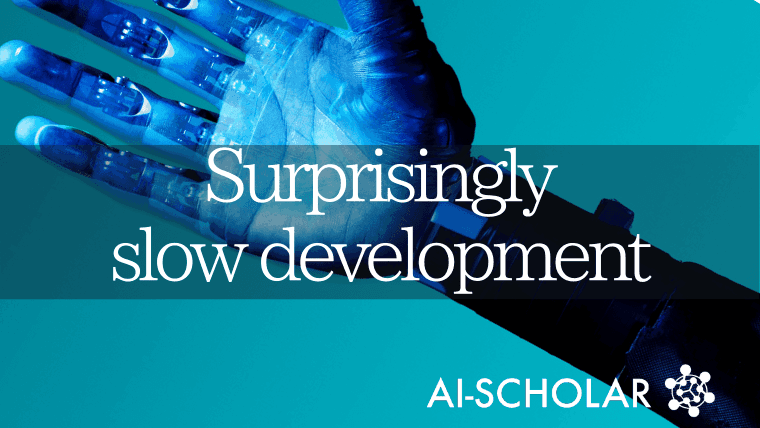
D2RL: A New Neural Network Architecture For Reinforcement Learning
3 main points
✔️ Proposal of RL-CycleGAN, a new sim2real method
✔️ RL-scene consistency loss enables the generation of images while retaining task information.
✔️ A high success rate was achieved in the object grabbing task.
D2RL: Deep Dense Architectures in Reinforcement Learning
written by Samarth Sinha, Homanga Bharadhwaj, Aravind Srinivas, Animesh Garg
(Submitted on 19 Oct 2020)
Comments: Accepted at arXiv
Subjects: Machine Learning (cs.LG)
Paper Official Code COMM Code Projects
Introduction
This article presents an in-depth study of the architecture of neural networks in reinforcement learning, which shows that the architecture of neural networks is important in computer vision and natural language processing, and has a significant impact on the accuracy of learning and other aspects of However, there has not been much research on reinforcement learning. In this study, we investigated the effects of deeper neural networks and dense connections on reinforcement learning, and the results showed that deeper neural networks and dense connections are very important in the reinforcement learning task. From experiments and other results, we found that this was the case. We have also proposed a new dense connection method, Deep Dense Architectures for Reinforcement Learning (D2RL). This article will introduce these in detail.
To read more,
Please register with AI-SCHOLAR.
ORCategories related to this article