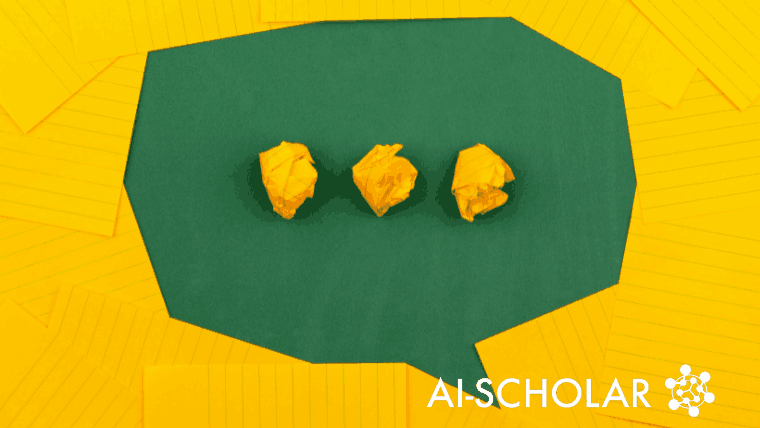
Controlling The Amount Of Medication With Messages! Using Reinforcement Learning To Investigate The Impact On Medication Adherence - And Treatment Motivation!
3 main points
✔️ Optimal control of medication therapy for diabetes requires patients' self-management behaviors in life - such as medication adherence.
✔️ This study describes a method for deriving messages, taking into account individual characteristics, in patients with type II diabetes using reinforcement learning, and an empirical experiment to investigate the impact of...
✔️ Empirical study utilizing a Randomized Controlled Trial (RCT) (REinforcement learning to Improve Non-adherence For diabetes treatments by Optimising Response and Customising Engagement (REINFORCE)).
REinforcement learning to improve non-adherence for diabetes treatments by Optimising Response and Customising Engagement (REINFORCE): study protocol of a pragmatic randomised trial
written by
(Submitted on 3 Dec 2021)
Comments: BMJ Open.
The images used in this article are from the paper, the introductory slides, or were created based on them.
background
Is it possible to promote behavior change through reinforcement learning?
This study proposes and demonstrates a method for deriving optimal messages considering individual characteristics using reinforcement learning to improve the effectiveness of medication adherence (i.e., patient behavior that promotes treatment, including medication compliance) for patients with type II diabetes. We are conducting a demonstration test.
Optimal control of diabetes requires daily self-management behaviors, especially medication adherence. Previous research has shown that text messages are effective in supporting adherence, but there are challenges in achieving higher effectiveness: one of them is that the content of the messages is generic and less effective in promoting behavior change in some patients; whereas reinforcement learning is based on the agent-environment, these interactions - the agent's behavioral choices and updates from feedback from the environment - can be used to optimize the system according to individual characteristics. Although such examples of reinforcement learning have been reported in dynamic regimes, there have been few examples of its application to improve the effectiveness of medication adherence.
This research aims to propose a method for deriving personalized text messaging based on reinforcement learning and to examine its impact on medication adherence in patients with type II diabetes: Specifically, it aims to develop a program for deriving messages and to test the effectiveness of the program through empirical testing. REinforcement learning to Improve Non-adherence For diabetes treatments by Optimising Response and Customising Engagement (REINFORCE) -The features of this research include The features of this research are as follows
(1)Randomized controlled trials (RCTs) in type II diabetes patients with poor glycemic control were introduced to ensure robustness and reliability.
(2) Designed to maximize internal validity and generality, and to periodically collect data
(3) A 6-month follow-up to assess both long-term medication adherence and clinical outcomes, such as glycemic control.
What is medication adherence?
Here is a brief description of medication adherence, which is addressed in this study.
Adherence refers to the patient's participation in decisions about treatment and receiving treatment accordingly - in particular, medication adherence refers to the patient's independent implementation of the treatment regimen for medication. Traditionally, health care professionals have evaluated patients based on a fixed concept of compliance - the degree to which patients follow the instructions of their health care providers; therefore, non-compliance - the failure to take medications regularly The problem of non-compliance is on the part of the patient. On the other hand, in medical practice, it has been pointed out that there are barriers to successful treatment that cannot be explained by the concept of compliance, and it has been increasingly reported that the key to successful treatment may be the patient's active participation in treatment - adherence. In other words, there is a need to move away from the idea that patients should be obedient to treatment. This patient-centered adherence is now being actively introduced: specifically, a policy of consultation with the provider to determine whether a treatment is feasible for the patient, what are the barriers to adherence, and what needs to be done to resolve them. The introduction of this policy is progressing.
What is Type II Diabetes?
In this section, we will discuss type II diabetes, which is the subject of analysis in this study.
Diabetes is a condition in which blood sugar levels in the blood vessels are high. The blood glucose level - the concentration of glucose or glucose in the blood - is an indicator of how much sugar is in the blood, which is used as energy for activity and rises sharply after eating and then slowly returns to normal. On the other hand, if the blood glucose level remains high - there is a lot of sugar in the blood - due to glucose intolerance, etc., vascular damage - the destruction of the blood wall, generation and rupture of blood clots, etc. - occurs, and organ damage occurs due to internal organ and brain functions, increased blood pressure, etc. In particular, the incidence of damage to organs with many capillaries - kidneys, brain, and liver - and organs with large blood vessels - heart, etc. - increases rapidly. Such a high blood sugar level is abnormal blood sugar - diabetes.
Diabetes mellitus has two main factors: decreased insulin secretion - type I diabetes mellitus - in which the pancreas produces less insulin to get sugar into the cells due to decreased function; insulin resistance - type II diabetes mellitus - in which the door to get sugar into the cells does not open properly; and insulin resistance -Type II diabetes mellitus-. Insulin is the so-called "key to the door" for getting sugar into the cells: in type, I, the production of insulin - the key to absorption into the cells - is reduced, and the concentration of sugar in the blood vessels increases. The cause is thought to be a decrease in insulin secretion in the pancreas, which is caused by heredity, etc. On the other hand, in Type II, excessive blood sugar elevation exceeds the tolerance level of the cell side, and the key that opens the door of the cell - insulin - does not function normally. Many cases of Type II diabetes are caused by lifestyle factors such as overeating and obesity. The control of diabetes is said to require self-management behaviors in daily life, such as physical activity and maintaining a healthy weight; in particular, adherence to medications that control blood sugar levels, such as insulin -medication adherence is emphasized.
purpose of one's research
In this study, we aim to develop and demonstrate a system that derives optimized messages for individuals using reinforcement learning to improve the effectiveness of medication adherence in such type II diabetes.
In medication adherence, several studies have reported the effectiveness of text messages, including reminder messages, in motivating patients to engage in treatment and promoting health behaviors; in diabetes, however, the effectiveness of text messages Such an approach predicts that tailoring content to individuals based on their past behavior could lead to greater behavior change. Therefore, this study aims to clarify the effectiveness of optimized messages by leveraging reinforcement learning to derive messages that take into account individual characteristics and evaluate the effectiveness of the intervention.
technique
This chapter describes the proposed method and the demonstration method - REinforcement learning to Improve Non-adherence For diabetes treatments by Optimising Response and Customising Engagement: REINFORCE- test.
First, we describe the REINFORCE test - design and analysis method - which is the demonstration method of the proposed method.
A two-arm randomized controlled trial designed to assess the impact of text messages tailored using reinforcement learning in the REINFORCE trial on medication adherence in patients with type 2 diabetes (see figure below).
The study aims to investigate the impact of regular text message delivery on medication adherence in patients aged 18-84 years who have been diagnosed with type 2 diabetes and are prescribed oral diabetes medication one to three times a day.
From the study protocol (see below), biweekly EHRs - electronic health records - will be used to identify eligible patients and select those who do not wish to receive a request to participate by opting out. Baseline questionnaires and electronic pill bottles for medication administration will then be mailed: electronic pill bottles have been used in many previous studies of adherence and are the dominant measurement method in adherence. Bottle data will be transmitted via an application on a mobile device. Subjects will then be randomly assigned in a 1:1 ratio to the intervention and control groups - this randomization will be based on the
(1) Baseline self-reported adherence levels, particularly less than one dose or missing more than one dose in the past 30 days; and (2) baseline HbA1c less than 9% or greater than 9%. At the end of the 6-month follow-up, patients are contacted by text message and asked to complete a follow-up questionnaire.
The study aims to investigate the impact of regular text message delivery on medication adherence in patients aged 18-84 years who have been diagnosed with type 2 diabetes and are prescribed oral diabetes medication one to three times a day.
intervention
This chapter describes the interventions that will be used in the demonstration.
At the core of the intervention is a reinforcement learning algorithm that personalizes daily text message outreach based on data from electronic pill bottles (see figure below).
The algorithm predicts which text messages a patient is most likely to take and derives the corresponding text messages - the effectiveness of each message is evaluated based on whether the patient took the medication the next morning. No text messages will be sent to the control group. A behavioral science-based questionnaire will be used to investigate how the text messages delivered to patients affect their behavior - do they improve medication adherence? From the feedback from these qualitative interviews, we have identified five elements that should be incorporated into the messages:
(1) Framing is classified as neutral, positive, and negative.
(2) Observed feedback: include in the text message the number of days - 0 to 7 - that the patient had evidence of taking medication in the previous week.
(3) Social reinforcement: mentioned in the text to acquaintances
(4) Nature of content: medication reminders, information about medications and lifestyle
(5) Introspection, e.g., design in texts that include introspective questions.
Based on these, we have designed text messages that incorporate various elements of the five factors selected from prior studies and other sources (see table below): specifically, text messages that include positive framing, observational feedback, social reinforcement, and reminder content, and do not include reflection, are 1 set of factors - Text 8 in the table below - is comprised. Each set of factors in the study included at least two text messages: a total of 128 messages containing 47 combinations of factors were developed.
Reinforcement Learning Algorithm
In this chapter, we discuss reinforcement learning algorithms in interventions.
This exam leverages HIPAA-Health Insurance Portability and Accountability Act-compliant Microsoft Azure to integrate the three elements:
(1) Electronic pill bottle data retrieved daily from Pillsy server
(2) REDCap patient data updated daily for predictors - age, gender, number of medications, baseline HbA1c, and patient activation
(3) A reinforcement learning predictive model algorithm publicly available on Microsoft Personaliser.
Predictors will update data on patients daily: new patient enrollment, discontinuation of diabetes medications using electronic pill bottles, the addition of new medications, and the number of medications required for adherence calculations. It also calculates medication adherence from the previous day by dividing the number of times the patient opened the pill bottle by the number of times it was prescribed - once or twice daily as assessed by REDCap data collection at baseline: adherence values from 0 to 1 represent reward The adherence values, ranging from 0 to 1, represent the reward - the feedback from the environment - and are trained to sum to the highest value, or, in the case of multiple drugs, the values are averaged together.
Reinforcement learning initiates random text message suggestions and observes individual feedback and subsequent medication adherence - over time, it begins to predict which factors should be included in the message the patient receives: in addition to rewarding medication adherence baseline characteristics, the number of days sent in each factor of the text message - so that similar messages are not sent in succession, whether the patient took the medication earlier - on the same calendar day and before the text message prediction for that morning in the same calendar day. If no adherence reward is received - no data is received from the patient's pill bottle - then the message is predicted based on the adherence reward to date. During the trial, 10% of the predictions will be randomly selected and targeted for model training. Text messages are sent daily using Microsoft Dynamics 365 SMS Texting, a HIPAA-compliant third-party platform managed by BWH.
In addition to the messages specified in the reinforcement learning, we send an introductory text on the day of randomization, a reminder text if the user has not been connected for more than 7 days and a final questionnaire at the end of the follow-up
Conclusion.
In this chapter, we describe the outcomes used in the demonstration.
The primary outcome (see table below) is medication adherence assessed during the first 6 months after randomization: measured as the mean daily medication adherence for each patient from the day after randomization until 183 days after randomization.
Secondary outcomes will include the change in glycemic control as assessed by HbA1c and self-reported medication adherence at the end of follow-up HbA1c values will be collected from routine measurements recorded in the EHR, and the closest value at the end of the 6-month follow-up for each patient up to 1 month after randomization will be used The closest value at the end of follow-up will be used - HbA1c is measured approximately every 3-6 months and is expected to contain missing values.
analysis
This chapter discusses the analysis in the demonstration.
We will divide the means and frequencies of the variables into intervention and control groups before randomization and compare these values with absolute standardized differences. Outcomes will also be assessed using the intention-to-treat-ITT principle for all randomized participants.
In the primary analysis, we will introduce a generalized estimation model for adherence and glycemic control using the same link function and normally distributed errors - for self-reported adherence, we will use the log link function and Poisson distribution errors to determine the relative risk of adherence relative risk to the proportion of patients in the intervention and control groups. The secondary analysis will adjust for any differences in baseline variables between the two groups despite randomization.
A sensitivity analysis - an analysis of the impact of a change in a variable on an outcome - will exclude patients who have stopped using the electronic pill bottle for more than 30 days. We will also assess the difference between the change in HbA1c from baseline and self-reported adherence for each of the three items that make up the self-report scale. Complete case analysis of glycemic control - HbA1c - and self-reported adherence will also be performed, with subgroup analysis stratified by age, gender, race/ethnicity, baseline HbA1c, baseline self-reported adherence, and the number of study medications. the number of study medications. At the end of the study, intervention patients will be clustered by their response to different text message factors, and the ability to predict these cluster phenotypes will be assessed using pre-randomization baseline information.
consideration
The purpose of this study is to examine the impact of reinforcement learning-based, personalized text messaging on medication adherence in patients with type II diabetes. Health behavior interventions need to be tailored to the needs and behavioral tendencies of the individual, and reinforcement learning is expected to be able to discover individual response patterns and personalize policies accordingly, thus deriving optimal communication for the individual; while Medication Adherence, on the other hand, there are few reports on the implementation of reinforcement learning, and its effectiveness is unclear. In this study, we developed a text derivation method and conducted an empirical study-REINFORCE-including an RCT, to examine the impact of reinforcement learning-based text messaging on medication adherence in patients with type II diabetes.
Prior research has introduced reinforcement learning to improve health outcomes: for example, different text message approaches have been utilized in patients with type II diabetes to examine the impact on exercise; and intervention in patients with diabetes has improved physical activity There is an increase in physical activity of more than 20% by utilizing a non-adaptive - not optimized for the individual - approach (p<0.001). On the other hand, these may be able to achieve higher effectiveness because the messages patients receive are not personalized. In addition, because text messages are less costly and can be implemented for patients with limited access to hospitals, optimized messages are expected to achieve relatively higher effectiveness.
Possible challenges for this study include deficits in secondary outcomes; the impact of electronic pill bottles; lack of generality. First, there may be deficits in secondary outcomes, such as self-reported adherence. In actual surveys, self-administered questionnaires are often used, and deficits in these outcomes can be expected due to forgetting to write them down; therefore, in this study, we use the imputation method - a type of missing value completion method - to In this study, we use the Imputation method - a kind of completion method for missing values - for completion. Another possible measure is to check for omissions by several people at the time of data collection. Secondly, the electronic pill bottles used in the intervention may have an impact. Because the electronic pill bottle can accurately measure the actual dose, monitoring may cause observers to be aware of the amount of medication taken, which may lead to bias in randomization and other processes; in this study, we are trying to solve this problem by introducing electronic pill bottles in both the control and intervention groups. Third, is the lack of generality: it may not be scalable to cases where text messages are hard to reach, such as patients with pre-diabetes only. Therefore, a possible solution for these patients would be to use a sequential dialogue system instead of messages.
Categories related to this article