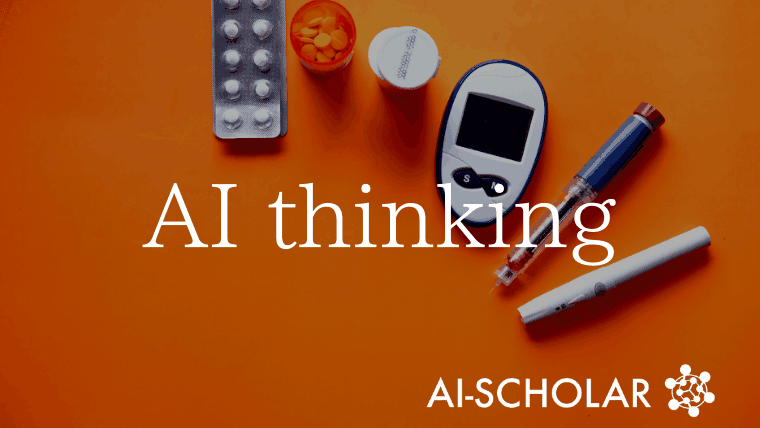
AI Thinking About Inequality! A Proposal For Simulating Dynamic Regimes Using Reinforcement Learning To Clarify Racial Differences.
3 main points
✔️ For Type 1 Diabetes (T1D), a type of diabetes, several studies have shown an association between ethnic and racial differences and outcomes.
✔️ Using reinforcement learning (RL) to derive a dynamic regime for T1D, we aim to clarify the effect of racial differences on treatment effects.
✔️ Mean HbA1c decreased by 0.33% in the non-white subgroup when they received the same diabetes treatment regime as the white subgroup, and about 35% of the estimated difference between the two subgroups was explained by racial differences.
The Impact of Racial and Ethnic Health Disparities in Diabetes Management on Clinical Outcomes: A Reinforcement Learning Analysis of Health Inequity Among Youth and Young Adults in the SEARCH for Diabetes in Youth Study
written by
(Submitted on 1 Jan 2022)
Comments: Diabetes Care.
PMID: 34728528 PMCID: PMC8753766 (available on 2023-01-01) DOI: 10.2337/dc21-0496
Readers may use this article as long as the work is properly cited, the use is educational and not for profit, and the work is not altered. More information is available at https://www.diabetesjournals.org/journals/pages/license.
The images used in this article are from the paper, the introductory slides, or were created based on them.
background
Can AI shed light on the impact of complex social factors on treatment?
In this study, we will use reinforcement learning to simulate a dynamic regime for type I diabetes and clarify the treatment effects of strong social factors such as racial differences.
Several studies have shown racial and ethnic differences in the symptoms of type 1 diabetes (T1D), a type of diabetes: In particular, black, American Indian, Hispanic, and Asian/Pacific Islander youth and young youth and young adults (YYA) have higher HbA1c (hemoglobin A1c, a measure of diabetes) and higher prevalence of early complications compared to non-Hispanic whites. One of the reasons for this is social One of the reasons for this is that it is difficult for non-Caucasian YYA to receive the same treatment regimes as Caucasian YYA, due to social disparities, and it is assumed that these health disparities lead to differences in treatment effectiveness and outcomes. On the other hand, it is not clear whether these differences in diabetes treatment regimes and technologies contribute to racial differences in T1D: that is, factors other than the diabetes regime - social determinants: differences in access to care and diabetes education; lower utilization of diabetes care; food insecurity - are challenges that have not been fully taken into account. This study used reinforcement learning to homogenize treatment strategies across ethnic groups and to identify racial differences in treatment effectiveness for T1D.
What is Type I Diabetes?
Here, we briefly describe type 1 diabetes, which is the subject of analysis in this study.
Diabetes mellitus is a disease of high blood glucose levels in the blood vessels. The blood glucose level - the concentration of glucose or glucose in the blood - indicates the amount of sugar contained in the blood, which rises sharply after a meal and then slowly returns to normal; on the other hand, when the blood glucose level is high - there is a lot of sugar in the blood, On the other hand, high blood glucose level due to glucose intolerance and so on - high sugar in the blood - causes blood vessel damage - breakdown of blood wall, generation and rupture of the blood clot, etc., and organ damage due to internal organs, brain function, increased blood pressure, etc.; especially, organs with many capillaries - kidney, brain, In particular, the risk of damage to organs with many capillaries - kidneys, brain, liver - and organs with large blood vessels - heart, etc. - increases rapidly. This high blood glucose level is called abnormal blood glucose - diabetes.
There are two main types of diabetes: hyposecretion of insulin, which is a decrease in the amount of insulin produced by the pancreas to bring sugar into the cells - type I diabetes; and insulin resistance, which is the failure of the door to open to bring sugar into the cells -Type II diabetes mellitus-. Insulin is the so-called "key to the door" for getting sugar into the cells: in type, I, the production of insulin - the key to absorption into the cells - is reduced, and the concentration of sugar in the blood vessels increases. In the case of Type II, excessive increase of blood glucose due to lifestyle, etc. exceeds the tolerance level of cells, and the key to opening the door of cells - insulin - does not function normally. The key that opens the cell door - insulin - does not function properly. In the United States, due to the ethnic diversity of the population, there is a strong awareness of the problem of Type I diabetes and high demand for solutions.
purpose of one's research
The purpose of this study was to clarify the influence of social factors on the difference in treatment effects by using reinforcement learning to derive a treatment regime for T1D that takes into account racial differences.
While previous studies have indicated that T1D outcomes differ by race, it has been difficult to clarify the effect of race on treatment effects because of the complex social factors involved. In this study, we use reinforcement learning to construct a model to estimate the outcomes of the same treatment for different races and to clarify the influence of social factors on the treatment effect: Specifically, we use statistical information-based distribution generation and propensity score matching to estimate the effect of non-Hispanic white YYA to estimate differences in glycemic control and diabetic complications in a hypothetical scenario in which YYA receive a T1D treatment regime equivalent to non-Hispanic white YYA. Such studies are expected to clarify the relationship between race/ethnicity, diabetes regime, and outcomes for T1D YYA, provide clarity on issues that need to be addressed and facilitate interventions for factors including social context.
technique
data set
In this section, we describe the data sets that were analyzed.
The dataset used in this study was collected in the SEARCH for Diabetes in Youth Study, one of the cohort studies on diabetes. This study included patients diagnosed with diabetes under 20 years of age using a registry network at five sites in the U.S. Of the 978 YYA with T1D, 47.3% were female, 77.5% were non-Hispanic white, and the mean age was 12.8±2.4 years. Compared with the Caucasian subgroup, the non-Caucasian YYA subgroup had lower rates of insulin pump use (non-Caucasian: Caucasian = 1.8% vs. 11.5%, 4 or more times per day). They were less likely to have a glucose test - non-whites: whites = 76.8% vs. 84.6%. Mean HbA1c was 9.2% and 8.2% for the nonwhite and white subgroups, respectively. Early diabetic complications investigated at the start of the cohort included: diabetic retinopathy; diabetic kidney disease (DKD); peripheral neuropathy
statistical analysis
In this chapter, we describe the statistical processing performed in this study.
This analysis was conducted to estimate the effect of two different presumptive T1D treatment regimes on outcomes: the YYA was categorized into a non-White race/Hispanic ethnicity-non-White subgroup and a non-Hispanic White race The YYA were categorized into two subgroups: non-white subgroups of non-Hispanic ethnicity and Hispanic ethnicity, and non-Hispanic white subgroups. Statistical analysis consisted of two steps: 1) estimation of the distribution of treatment regimes in the nonwhite and non-Hispanic white subgroups, and 2) estimation of outcomes under the assumption that nonwhite YYA received the treatment regime distribution of the white subgroup. In this study, the diabetes treatment regime was defined as the frequency of two types of insulin administration methods, SMG - self-monitoring of glucose, and glucose self-monitoring - before the survey and at the cohort visit -was composed of three variables.
Estimation by propensity score modeling
In this chapter, we describe the estimation of distributions for treatment regimes using propensity score matching. We use πWhite to denote the treatment regime-treatment distribution in the Caucasian subgroup and πnon-White to denote the treatment distribution in non-Caucasians. We model the propensity score from data from the SEARCH cohort and multiple variables -age, sex, SEARCH study site, SEARCH visit, and T1D duration- to control for and estimate the treatment regime distribution in the two subgroups. In the analysis, multinomial logistic regression was used to fit the probability of treatment choice for the aforementioned covariates, and outcomes were treatment regime-insulin modality and self-monitoring of glucose, SMG -(SMG). Here, we fitted separate propensity score models for insulin administration method and frequency of SMG - pre-cohort visit and cohort visit. In addition, different models were fitted for the pre-cohort visit and cohort visit in the frequency of SMG.
Estimating the Impact of Diabetes Treatment Regimes on Outcomes
Here, we describe the second step of the analysis, estimating the effect of diabetes treatment regimes on non-white YYA outcomes.
In this study, we used Q-learning, a type of reinforcement learning, to estimate the mean HbA1c and risk of complications - diabetic retinopathy, DKD, and peripheral neuropathy - for non-white YYA, assuming that they received the treatment regime estimated for the white subgroup. These complications include For these complications, we estimated the risk differences between different treatment regimes for the estimated distributions. The constructed model also accounted for racial/ethnic differences in age at diagnosis, sex, SEARCH site, T1D duration, highest parental education, type of health insurance, smoking status, physical activity, screen time, and aggregated non-Hispanic black-non-white subgroups -indicator variables were employed, and sensitivity analyses examined the rationale for modification for models stratified by gender.
result
In this section, we describe the evaluation and results of the model constructed in this study.
Estimation of Diabetes Treatment Regimes by Multivariate Propensity Score Modeling
In this chapter, we describe the estimation of dynamic regimes using propensity score modeling.
Propensity score modeling showed that YYA in the non-white subgroup differed in the distribution of treatment regimes and also in terms of SMG frequency (see below).
Consistent with population-level trends in propensity score ratios (see below), each individual in the nonwhite population was estimated to use an insulin pump or SMG four or more times per day more frequently in πwhite than in πnon-white.
The impact of racial differences on diabetes treatment regimes
In this section, we describe the results of our estimates of the effects of racial differences.
In the nonwhite subgroup of YYA, the white treatment regime was lower than the nonwhite treatment regime in mean HbA1c: nonwhite: 9.1±0.1%; white: 8.7±0.1%. The estimated difference for non-Caucasian YYA who received a simulated treatment regime that accounted for racial differences was 0.33%. The risk of developing complications in T1D - including diabetic retinopathy, DKD, and peripheral neuropathy - was all lower in the Caucasian treatment regime: diabetic retinopathy: 6.0%; DKD: 9.1%; peripheral neuropathy: 5.9%.
Comparison of Estimated Treatment Regime Differences and Outcome Differences Across Racial Groups
Here, we describe the impact of different treatment regimes on T1D outcomes.
Based on the difference between non-Caucasians and Caucasians in mean HbA1c - 0.9% - the estimated difference by treatment regime was about 35% - 0.95% of the difference in HbA1c - 0.33%. In addition, the risk differences by treatment regime in the prevalence of the three complications were as follows: diabetic retinopathy: about 269%; diabetic nephropathy - DKD - 71%; peripheral neuropathy - 81%.
consideration
This study aimed to estimate diabetes treatment regimes for T1D using reinforcement learning and to identify differences in treatment effects by race. multiple studies have reported race differences in T1D, but these factors are often associated with factors such as social background -While several studies have reported racial differences in T1D, these factors are often associated with social background and other factors such as differences in access to health care facilities and poverty disparities that obscure the impact on outcomes; therefore, this study uses RL to estimate and compare T1D outcomes under the assumption that individuals receive similar treatment regardless of race. We aimed to clarify the impact of biological differences by estimating and comparing outcomes. In the evaluation, the longitudinal cohort data homogenized the essential elements in treatment between races - Caucasian and non-Caucasian - and identified a 0.33% reduction in mean HbA1c: this difference was statistically significant and accounted for approximately 35% of the difference in HbA1c between the non-Caucasian and Caucasian subgroups c between the nonwhite and white subgroups. From this result, it was inferred that the differences in HbA1c and prevalence of complications reflect unmeasured related factors - institutional, interpersonal, and internal racism and marginalization: e.g., barriers to receiving specific diabetes treatment regimes, support.
One of the advantages of this study is the clarification of the contribution to health disparities. The proposed method quantifies the contribution of diabetes management to health inequalities by estimating outcomes under hypothetical treatment scenarios using distributions and RLs in the treatment regime - this allows us to identify the potential effects of treatment on outcomes at the time of measurement and allow adjustments to treatment regimes to be made.
Challenges also include a lack of analysis of dynamic factors in racial disparities; a small sample size in non-Caucasian YYA. Many of the social factors included in racial disparities are likely to influence diabetes management and outcomes, including ease of access to health care. In addition, many of the social factors are heterogeneous at the population level and change over time. The present study lacks data on these social factors from the perspective of a cohort study, and it is necessary to conduct analyses that compensate for these factors in the future. In addition, because of the small sample size of non-white YYA in this study, specific racial groups were combined into one group for analysis - therefore, it is highly likely that the effects of different forms of socialized and structured racial differences were not fully considered. In addition, due to the limitations of the sample size, we were not able to conduct analyses that took into account aspects of cross-sectionality, and thus may not have been able to adequately capture the effects between factors. As a solution to these problems, a more in-depth survey and the introduction of a learning method for situations with a small sample size - model-based learning - are considered.
Categories related to this article