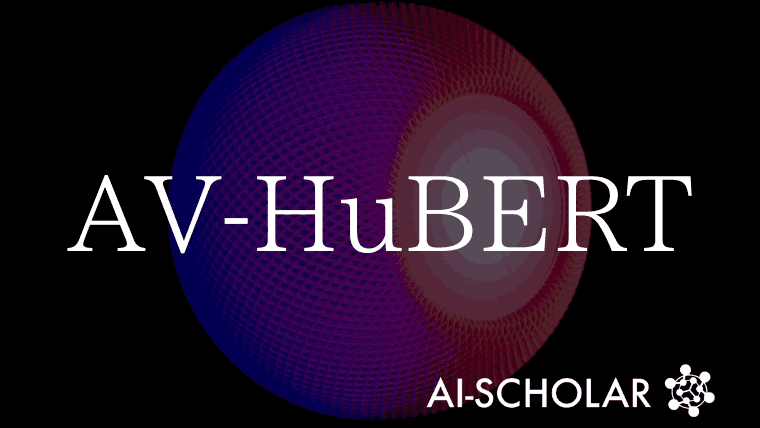
Fusion Of Speech And Image! Does The Multimodal Method "AV-HuBERT" Shine In Speech Recognition For The Dysarthric?
3 main points
✔️ First proposal for multimodal dysarthric speech using visual information
✔️ VGG and AV-HuBERT learning
✔️ Significant improvement in speech comprehension and naturalness
Exploiting Audio-Visual Features with Pretrained AV-HuBERT for Multi-Modal Dysarthric Speech Reconstruction
written by Xueyuan Chen, Yuejiao Wang, Xixin Wu, Disong Wang, Zhiyong Wu, Xunying Liu, Helen Meng
[Submitted on 31 Jan 2024]
comments: To appear at IEEE ICASSP 2024
subjects:Sound (cs.SD); Audio and Speech Processing (eess.AS)
The images used in this article are from the paper, the introductory slides, or were created based on them.
Introduction
Nice to meet you all!
I am Ogasawara, a new writer for AI-SCHILAR.
The paper presented here is this one
Multimodal restoration of dysarthric speech using AV-HuBERT with visual features.
It is.
As I summarized the main points at the beginning of this article, the goal seems to be to improve the accuracy of speech recognition by using visual information to improve dysarthric speech.
I wonder what kind of methods are being used!Let's learn together with me little by little~~!
I will try to introduce them in as concise a manner as possible, so please bear with me until the end.
What is dysarthria?
First of all, let us keep in mind what dysarthria is. Dysarthria is simply a defect in the articulatory system, either congenital or acquired, that prevents normal speech production.
This disorder is most often seen in patients with acquired cerebral palsy. Another important characteristic of dysarthria is that the characteristics and tendencies of speech production vary from person to person.
Because of these characteristics, research in the field of dysarthric speech recognition has been challenging. See the figure above for a flow chart of dysarthric speech recognition.
Outline of this study
Let's review the speech characteristics of dysarthric people.
- Irregular speech
- Nasal, muddled speech
- Speech features and tendencies vary from individual to individual
These three were the main characteristics of dysarthria, weren't they?
In this research, we aim to improve the accuracy of speech recognition by using multimodal technology that combines visual and auditory information to address this issue.
Proposed Method
The figure above shows the method proposed in this paper.
I know it is difficult to understand this all by looking at it, so let's unpack it one at a time.
The goal of this article is to be able to look at and understand this model after reading it.
I'm going to chew it up and explain it to you as I mentioned earlier, so please follow me to the end!
What is multimodal?
Let's hold on to this first. Multimodal refers to a technique that uses multiple pieces of information. For example, like this one, it is like audio information x video information.
Doesn't it seem strange to use video information for speech recognition? But, the accuracy of speech recognition will be improved. The researchers who were the first to devise this idea are amazing.
This technique is not only used for speech recognition, but also for generative tasks, which is a hot topic these days.
VG
It is one of the deep learning methods. The feature is that the convolution layers are unified as 3x3. This reduces the number of parameters.
I could go into more detail, but it is sufficient to remember that this is one of deep learning.
AV-HuBERT
This is a derivative model of HuBERT, developed by Meta. This is a multimodal model that uses video information, so it can perform tasks such as lip-reading as well as speech recognition. As a machine learning method, it is a self-supervised learning model,
voice restoration
Are you familiar with speech synthesis and voice quality conversion technologies? Both of these technologies use machines to produce speech. Although they are very innovative technologies, it is difficult to maintain the speaker's voice. This voice restoration technology has been developed with the aim of maintaining the speaker's voice.
After acquiring prior knowledge
Yes, I am. I have explained the preliminary knowledge so far, but have you all been able to keep up?
This paper compares three methods, but what I want you to keep in mind is the structure and model of AV-HuBERT, a derivative of HuBERT, so I will skip the other two methods. If you are interested, please read the original paper.
Now let me explain the methodology!
- Audio and image input.
- Audio is sent to the audio feature extractor and images are sent to the image feature extractor.
- Extracted features are docked
- Docked features are sent to pre-trained AV-HuBERT
- Processed by AR decoder
This is the flow using the AV-HuBERT model. Did you understand it? At first, some of you may have thought, "What the heck is this? I know some of you may have thought, "What the heck is this?
In this article, we aim to give you a rough idea of what we are talking about, so we have omitted detailed explanations of the mechanisms and mathematical formulas. We hope that you will get the gist of what we are talking about.
Do you understand? Reflection on the past
There are only three important things!
Let's just hold on to this!
- Multimodal method using both audio and image information
- This paper is the first speech recognition method using multimodal
- Verifying the cleanliness and naturalness of voice restoration.
As long as you have these three things in mind, the rest will be fine!
In the next section, we will look at the experiment.
This is where we start! About the Experiment
Thank you very much for reading this long explanation of the basics.Next, I will finally explain the most interesting part of the paper, the experiment.
Experimental setup
Now let's talk about the experimental setup. Three English speech data sets are used in this experiment. The experiment uses three English speech data sets, which also include speech for people with disabilities.
Here, the experiment in this study is validated by selecting four of the disabled voices in the data set and creating individual systems tailored to each of the four.
What are the results of the experiment?
Here are the results of this experiment, what I want you to pay attention to. It is the third column from the left.
This is the main feature of this issue, the character error rate result of the method using AV-HuBERT.
The result is a successful reduction in text errors! However, in my opinion, this result does not seem to be balanced with the difficulty of system development. I am a little disappointed. This seems to be an area where there is still a lot of room for research.
The reason why I am disappointed is that the text error rate improves even with the usual HuBERT method optimized for handicapped speech. Well, to put it simply, you don't have to go to such great lengths.
But that's only when looking at the text error rate only. What this method generates is speech. And it maintains the speaker nature. This makes the results of this experiment very valuable, and if it could be used for one-on-one communication, it would greatly improve the ease of communication.
The study used a listening test as a subjective comparative study; all of the AV-HuBERT models scored higher, and the more severely ill patients in particular showed that the system was more effective.
Summary of Dissertation
Thank you all for your hard work. What I introduced this time was a multimodal method using visual and audio information to generate speech that preserves the speaker's nature. For me, it was a very interesting research. The improvement of character recognition rate was not so good, but it is possible to generate speech that is easier to listen to while maintaining the speaker's identity.
The results of this study can be summarized as follows
- It is possible at this stage to generate speech that is easy to listen to while maintaining talkativeness.
- Multimodal methods are also effective in speech generation tasks
The two major results of the project are
A little chat with a chick writer, Ogasawara
The road to becoming a researcher is long and arduous.
You have to get a master's degree and then a doctorate, so you have a longer preparation period than the average person. Moreover, the more you advance, the more difficult it becomes. It is really long and steep.
And it was not easy to get a post after getting a doctorate. But I have already made up my mind.
Let's move on.
See you in the next article.
This is Ogasawara, a newbie chick writer~.
See you later!
Categories related to this article