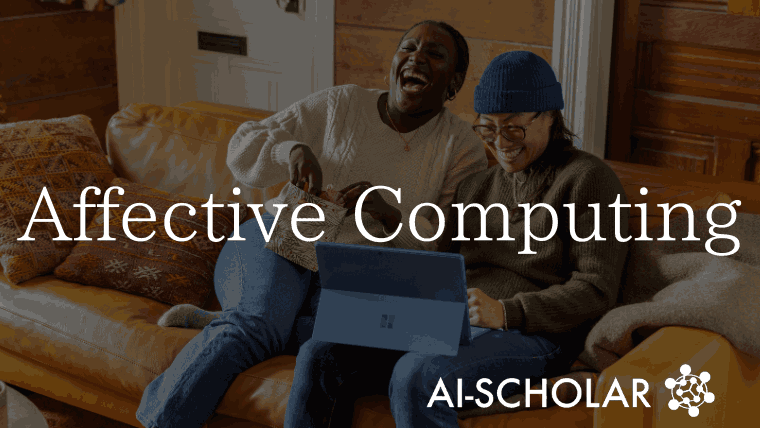
Models And Datasets For Affective Computing: A Survey Of Models And Datasets For Affective Computing
3 main points
✔️ classification of affective computing research
✔️ introduction to emotion recognizers and datasets
✔️ sorting out outstanding issues in affective computing research
A Comprehensive Survey on Affective Computing; Challenges, Trends, Applications, and Future Directions
written by Sitara Afzal, Haseeb Ali Khan, Imran Ullah Khan, Md. Jalil Piran, Jong Weon Lee
(Submitted on 8 May 2023)
Comments: Published on arxiv.
Subjects: Artificial Intelligence (cs.AI)
code:![]()
The images used in this article are from the paper, the introductory slides, or were created based on them.
Introduction
Affective computing refers to efforts to recognize human emotions, sentiments, and sensations, and has already been the subject of much research in the fields of linguistics, sociology, psychology, computer science, and physiology.
This paper will provide a bird's eye view of the importance of effective computing, its ideas, concepts, and methods.
Affective computing is an idea proposed by Picard in 1997, and many applications have been devised since then. On many social media platforms, affective computing is considered useful for understanding people's thoughts. Many researchers also believe that affective computing systems are important for the development of human-centered AI and human intelligence.
Three main methods have been investigated for identifying human emotions
There are three types of emotion recognition: visual emotion recognition (VER), auditory/speech emotion recognition (AER/SEER), and physiological emotion recognition (PER). These have been studied extensively in the context of machine learning. In addition, mixed reality (XR) technologies such as VR can improve people's subjective emotional experience, and several related studies have been conducted.
This survey paper provides a broad survey of state-of-the-art emotion recognition methods and their applications.
Case Studies in Emotion Recognition Research
This section presents various examples of emotion recognition research, including machine learning and deep learning.
Text-based emotion recognition
There are text-based sentiment recognition methods, many of which employ statistical or knowledge-based approaches. For example, methods have been developed to classify sentiment from large amounts of user-generated text data on online social media and e-commerce systems.
With the advent of deep learning techniques, it is now possible to automatically extract features from text data and train classifiers end-to-end. Research on text-based emotion recognition using deep learning and machine learning is summarized in the following table.
Voice-based emotion recognition
Methods also exist to recognize data such as utterances and identify emotions. Again, a large number of methods are based on machine learning and deep learning, and techniques such as support vector machines and neural networks are used. The following table represents speech-based emotion recognition research. As for the type of neural network, there are examples of using CNNs and RNNs.
Visual Information Based Emotion Recognition
There are also methods that use images and videos to recognize emotions from images of facial expressions. This one is summarized in the following table. We can see that the elemental technologies used are CNN, Attention, and autoencoder. We can also see that there is a wide variety of datasets.
Data-set
This section introduces the datasets used in text, auditory, and visual-based emotion recognition.
Text-based data set
The Multi-domain Sentiment database (MDS) contains over 100,000 phrases from Amazon reviews, categorized as positive or negative, and assigned five sentiment categories. The IMDB is a widely used dataset of 25,000 movie reviews for both training and testing.
Auditory information-based data set
There are two types of speech and voice databases: those that use intentionally uttered speech and those that use speech taken from natural speech. The former includes the Berlin Database of Emotional Speech (Emo-DB). However, the problem is that intentional utterances are often more exaggerated than natural ones, and the latter database has been proposed as a solution to this problem.
Visual information-based data set
There are some old examples of data sets for emotion recognition from facial expressions that were collected in the laboratory. For example, at JAFFE, image data of 7 different facial expressions were collected. Recently, datasets such as FER2013, a dataset of 35,000 facial images automatically collected by image search, have been proposed. The following figure shows an example of one of the various datasets. The following figure shows an example of the various datasets.
Challenges facing emotion recognition technology
Previous machine learning-based emotion recognition models had the problem of being difficult to share across domains, since the feature expressions were created as task-specific and domain-specific.
The general view is that CNN-based methods are effective when dealing with still images, while RNN-based methods are effective when dealing with time series data, and deep learning techniques are effective when dealing with facial expression images and physiological data. Other deep learning techniques such as adversarial learning, attention methods, and autoencoders are also used. Although many features are automatically learned by these deep learning techniques, the challenge is that they do not show such significant improvement over machine learning techniques in emotion recognition from physiological data.
Finally, the outstanding issues in affective computing research are summarized as follows
- Build technology to identify emotions more accurately and with greater confidence.
- Building large and diverse labeled datasets
- Creation of standardized emotion classification criteria for use in labeling
- Building Robust and Interpretable Machine Learning Models
- Building a personalized emotion recognition model
Summary
The paper presented in this issue surveyed a wide range of studies that proposed models and datasets related to effective computing, and it is expected that there are still many challenges in the field of effective computing and further developments will be expected in the future.
Categories related to this article