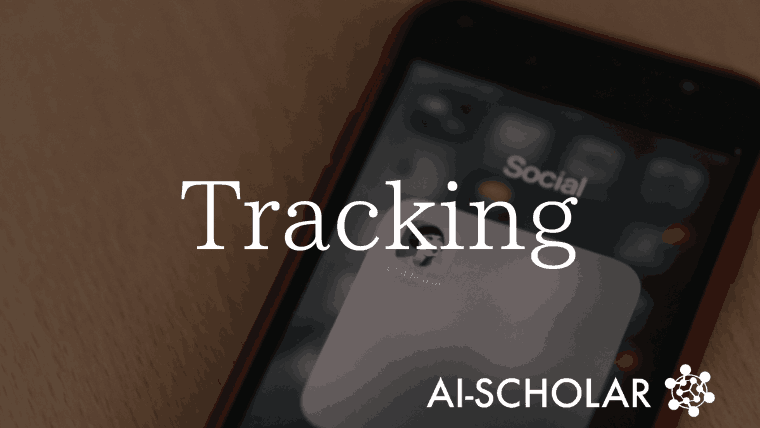
Tracking The Manipulation Of Information On Twitter In The Seven Months Leading Up To The Attack On The U.S. Capitol
3 main points
✔️ Visualize organized clusters of tweets on Twitter in the seven months leading up to the 2021 attack on the United States Capitol
✔️ Detect clusters of users from over 500 million election-related tweets and their hashtags
✔️ Visualize user relationships to tweets with high text similarity Discover the manipulation of information on Twitter that was behind the attacks by visualizing the relationship between users and tweets with high text similarity
Tracking Fringe and Coordinated Activity on Twitter Leading Up To the US Capitol Attack
written by Padinjaredath Suresh Vishnuprasad, Gianluca Nogara, Felipe Cardoso, Stefano Cresci, Silvia Giordano, Luca Luceri
(Submitted on 9 Feb 2023)
Comments: AAAI 2023
Subjects: Social and Information Networks (cs.SI); Human-Computer Interaction (cs.HC)
code:![]()
![]()
The images used in this article are from the paper, the introductory slides, or were created based on them.
Introduction
On January 6, 2021, supporters of then U.S. President Donald Trump attacked the U.S. Capitol building in a tragic incident that left five dead and many injured.
The case was subsequently deemed an attempted coup organized and promoted online, and the U.S. House of Representatives established a special committee to investigate the role of social media in relation to the case, which led to a renewed awareness of the impact of social networking sites.
This paper describes a paper that leverages a dataset of over 500 million election-related tweets collected between July 2020 and January 2021 to visualize the manipulation of information on Twitter in the seven months leading up to the Capitol attack and the relationships between them and the impact they had The paper will be discussed.
Dataset & Fringe Hashtags
In this paper, we collected a series of tweets from July 2020 to January 2021 that were directed to a series of politicians, including candidates for election, using election-related keywords.
We then created a large dataset based on the collected tweets, consisting of52% retweets, 19% replies, 16%original tweets, and 13% quotes.
Utilizing existing research and numerous reports on the Capitol attack, we also identified 19 hashtags (=Fringe Hashtags) formed by the core users and their surrounding communities.
Details and distribution of Fringe Hashtags are now as follows
The figure shows that Fring Hashtags can be divided into the following three main categories.
- US Election: hashtag encompassing comments inciting fraudulent elections
- QAnon: a hashtag associated with conspiracy theories and political movements based on them advocated by right-wing factions in the United States
- COVID-19: Hashtags related to conspiracy theories about coronaviruses
In addition, we see that #stopthesteal ( a hashtag used by Trump supporters to accuse Democrats of voting fraud) and #dobbs (a hashtag used in response to comments supporting political commentator Lou Dobbs) are by far the most shared.
Experiments
Using the dataset created, the following three experiments were conducted in this paper to visualize the manipulation of information on Twitter in the seven months leading up to the attack on the Capitol and the relationships among them.
- Visualize the interaction network of rapidly spreading retweets (Rapid Retweet Network)
- Discover clusters of users sharing tweets with similar content (CopyPasta Network)
- HTEMap (Hashtag Temporal Evolution Mapping), an extension of the existing model, to map hashtag relationships and temporal trends over the 7 months leading up to the Capitol attack
Let's look at them one by one.
Rapid Retweet Network
Retweets are the easiest way to share content on Twitter, but their simplicity can lead to malicious manipulation of information by organized movements.
Therefore, in this paper, we visualized the Rapid Retweet Network according to existing research in order to identify a network of users suspected of systematic information manipulation through rapid retweeting (Rapid Retweet ).
The figure below visualizes the Rapid Retweet Network in the dataset created, with nodes representing users, edges representing Rapid Retweets, and the size of the nodes representing the number of times a user was retweeted.
The figure shows that the Rapid Retweet Network consists of a star-shaped network structure, and that a single user is retweeted by many accounts.
In particular, recent literature has shown that such star-shaped interaction structures are likely evidence of organized online manipulation, and manual identification of the users at the center of such structures can identify highly influential users within this network structure It is believed that this is the case.
CopyPasta Network
Like retweets, highly similar tweets run the risk of being used for intentional manipulation of certain ideas or information.
The Internet term for such tweets with high text similarity is CopyPasta Tweet, and the network composed of them is called the CopyPasta Network.
In this paper, we constructed the CopyPasta Network using an undirected network that calculates a similarity score between each tweet and links them to each other if they are above a threshold value (= 0.7).
The figure below shows the CopyPast Network constructed through this experiment, with the left labeled based on hashtags and the right labeled for tweets with misleading content related to the election.
The nodes represent tweets and the colors represent hashtags embedded in the tweets, with green (76.25% of all tweets) on the left and purple (83% of all tweets) on the right being #stopthesteal and related content, resulting in the majority of these CopyPasta Tweets These CopyPasta Tweets accounted for the majority.
It is interesting to note that in this group of users, only a few (48) overlapped with the users involved in the Rapid Retweet Network described above, confirming that the experiment was not limited to a specific set of users but included a very diverse set of users engaging in organized behavior. This experiment confirmed that not only a limited number of specific users, but also a very diverse range of users are engaged in organized activities.
HTEMap (Hashtag Temporal Evolution Mapping)
Finally, we used HTEMap (Hashtag Temporal Evolution Mapping) to visualize the time series changes in Fringe Hashtags over the seven months prior to the Capitol attack.
HTEMap is an extension of the model proposed in Sato et al. (2021), which visualizes time-series changes in hashtags by mapping the relationship between tweets and hashtags in a time series and constructing a hashtag co-occurrence network.
The HTEMap visualized by this experiment is shown in the figure below.
Each node in the figure represents a Fringe Hashtags, the size of the node represents the frequency of hashtags, the thickness of the edge represents the frequency of co-occurrence of two hashtags in HTEMap, and the color of the node represents a time series.
From this experiment, we confirmed that two interacting communities (QAnon cluster and Election-related cluster) were formed on Twitter.
Most notably, the civilwar hashtag is interrelated with the QAnon cluster, which suggests that organized pressure may have been involved behind the attack on the Capitol.
In addition, considering the time frame, QAnon-related hashtags spread much earlier than election-related hashtags, again confirming the high likelihood that such organized manipulation of information influenced the election.
summary
How was it? In this article, we described a paper that leveraged a dataset of over 500 million election-related tweets collected between July 2020 and January 2021 to visualize the manipulation of information on Twitter in the seven months leading up to the Capitol attack and the relationships between them and the impact they had The paper was published in the Journal of Political Economy and Political Science.
Visualization using the Rapid Retweet Network, CopyPasta Network, and HTEMap uncovered multiple groups of users who may have engaged in systematic manipulation of information, suggesting a complex conspiracy behind the election.
While this paper focused only on Twitter, future progress is expected as more comprehensive analysis is possible by considering other platforms.
The details of the data sets and visualization methods presented in this article can be found in this paper for those who are interested.
Categories related to this article