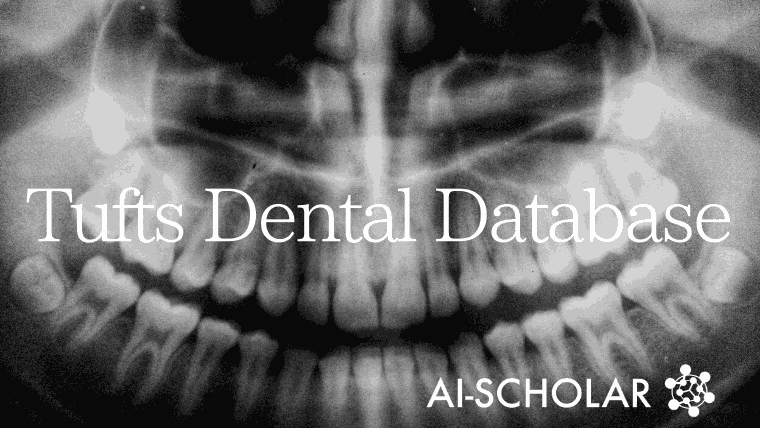
Even The Thoughts That Lead To Diagnosis Are Converted Into Data! What Is The Dental Panoramic Radiograph Data Set "TDD"?
3 main points
✔️ Introduction to the Tufts Dental Database (TDD) of dental panoramic radiographs
✔️ TDD is the world's first data set with complex information on tooth and jawbone position, presence of lesions, and degree of calcification
✔️ In addition to dental findings, the TDD includes eye-tracking information from the reader and textual data on the reasons for the diagnosis
Tufts Dental Database: A Multimodal Panoramic X-ray Dataset for Benchmarking Diagnostic Systems
written by
(Submitted on Oct 4 Oct 2021)
Comments: Published on IEEE J Biomed Health Inform.
The images used in this article are from the paper, the introductory slides, or were created based on them.
outline
Since dental panoramic radiographs contain a lot of information, they have been used in various machine learning applications, such as detection of tooth decay, detection of tumors, and risk assessment of osteoporosis. However, in some papers, tooth decay was detected, and in other papers, periodontal disease was evaluated, and the large amount of information contained in panoramic radiographs was not fully utilized.
The authors (Tufts University) created the Tufts Dental Database (TDD), a dataset of 1,000 panoramic radiographs that includes many dental findings such as anatomy, location, and extent of lesions, etc. The TDD is the world's first multimodal dataset TDD is the world's first multimodal dataset containing many dental findings such as anatomy, location, and extent of lesions.
Examples of radiographs used in dentistry
The three examples presented in this paper are as follows
From left to right, (a) periapical, (b) bitewing, (c) panoramic are written, which means dental, bitewing method, and panoramic.
Panoramic radiographs provide a comprehensive view of the upper and lower jaw, including the oral cavity, and provide a great deal of information in a single radiograph.
Issues surrounding dental AI
In this paper, we discuss the following reasons why AI technology is underutilized in dentistry
- The data cannot be accessed for privacy reasons.
- The data set is complex and multidimensional, with morbidity and health bias.
- The number is small compared to other data sets.
- There is no clear gold standard and experts are needed for annotation.
- There is no feedback on how or why the predictions were made.
In this paper, we present a dataset of 1000 panoramic radiographs, which in addition to the dental findings, is based on eye-tracking and interviewing of the dental radiologist's perception of the reasons for the diagnosis.
About Data Sets
Collection methods and imaging equipment
Photographs were randomly selected from panoramic radiographs obtained at Tufts University School of Dental Medicine Hospital from January to December 2014. However, high-quality images without blur or artifacts were selected. These 1000 images were annotated by a dental radiologist and a fourth-year dental student (who had completed the lectures and clinical training in oral and maxillofacial radiology and passed the examination).
The imaging equipment is an OP100 Orghopantomograph (Instrumentarimu Imaging / Kavo Kerr) and a Plammeca Promax 2D (Henry Schein), and the image density and contrast settings are automatically determined by the imaging equipment.
Segmentation Examples
The image below shows various masks for panoramic radiographs.
A single panoramic radiograph can contain multiple images: (a) the original radiograph, (b) the "abnormal" area, (c) a black-and-white image plotting the eye movement, (d) a color image showing the eye movement, (e) the tooth area, and (f) the entire upper and lower jaw including the oral cavity. (e) the tooth area, (f) the entire upper and lower jaw including the oral cavity.
Hierarchical Descriptive Method for Abnormalities
In this paper, the following hierarchical description of abnormal findings is designed to avoid ambiguity in expression and judgment criteria among raters.
- The first level (light blue) describes the anatomical location. It is divided into four categories: periapical, periapical, intraspinal, and areas unrelated to teeth.
- The second level (green) is a description of the area surrounding the abnormality. The two are clear or unclear boundaries.
- The third level (orange) is the radiological findings. These are permeability, opacity, and mixed septa, and calcification.
- The fourth level (indigo) describes the structures adjacent to the abnormal area. These are tooth displacement, root resorption, bone thinning or thickening, tissue degeneration, and soft tissue extension.
- The fifth layer (purple) is the classification of abnormalities. These were benign tumors and cysts, malignant neoplasms, inflammation, dysplasia, metabolic/systemic, traumatic, and developmental physiological.
Differences between dental radiologists and students
Below is a table showing the degree of agreement between the dental radiologist and the student on the location of the abnormal area noted.
Specialists have a larger number of abnormal findings to point out. On the contrary, students fail to find any abnormalities and give an evaluation of "None". Or, the diagnosis may be wrong.
3.5 Eye tracking and interviewing
We tracked the eye movements of raters as they evaluated panoramic radiographs. The time spent looking at an area (fixation time) is represented by the diameter of a circle, and the longer the eye gazes, the larger the circle becomes.
(b) is the segmentation mask of the abnormal area, and (c) and (d) show that the evaluator's gaze is fixed on the lesion area for a long time.
In addition, the audio data that the evaluator hears about the reasons that led to the decision is converted to text using speech recognition and added to the json file.
How to use the data set
The TDD is available on the website here, but users must submit a request form to obtain permission to download it.
The structure of the dataset is shown above and contains a total of 9000 images for 1000 cases. These include the aforementioned lesion segmentation masks and tooth segmentation masks as follows.
Examples of TDD usage
The authors use TDD to implement existing methods. For example, image enhancement (image processing to make the image easier to read) has yielded the following images.
AME and LogAME are metrics that are used to score the quality of a dataset and are inspired by subjective human evaluation. The smaller both of them are, the higher the contrast of the image is. In other words, the image is judged to be blur-free and easy to see.
The results of tooth segmentation are also shown below.
PA (pixel accuracy), IoU (intersection over union), and Dice coefficient are used as evaluation indices.
The purpose of this paper is to create a dataset, not to improve the performance of the machine learning model, so the numbers are not very significant, but the results are comparable to or better than existing reports.
summary
This paper presented the Tufts Dental Database, a dataset of 1,000 dental panoramic radiographs, which includes tooth segmentation, lesion segmentation, region of interest masking, eye tracking maps, and diagnostic reason text. The TDD contains data for tooth segmentation, lesion segmentation, masked regions of interest, eye tracking maps, and diagnostic reason text, making it a very useful dataset for the development of dental AI.
Categories related to this article