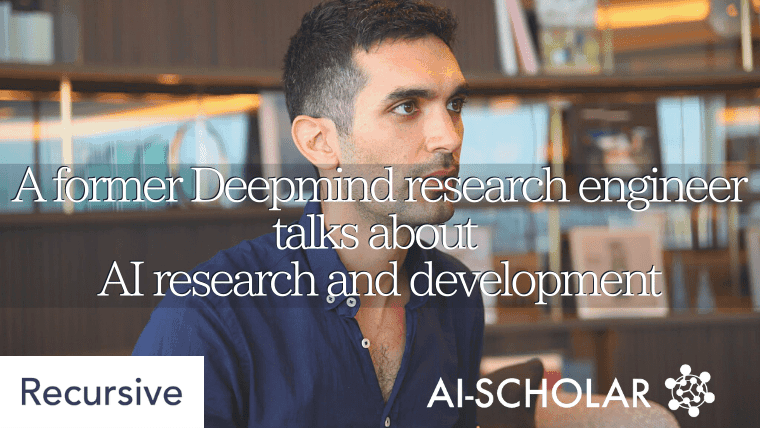
Learn From The Research Engineers Who Have Fought In The World!
It is said that Japan is lagging behind in AI. It is true that Japan is not advanced, but how is it lagging behind? Why the delay? What is the delay? We don't know for sure. But if we're going to be using AI more and more in the future and trying to fight the world, I think it's a good idea to ask people who have fought in the world.
In this interview, Tiago Ramalho, who worked as a research engineer at Google DeepMind, famous for creating AlphaGo, the go AI that made a big impression on the entire world, and who is currently founding Recursive Inc. in Japan to focus on supporting AI development to achieve the SDGs, told us about the atmosphere of AI development and the project.
About Us
Founded in August 2020, Recursive Inc. is a startup founded in August 2020 with the goal of achieving the SDGs through AI technology and business ideas, led by CEO Thiago from Deepmind and a team of AI-savvy professionals. Currently, our business operations are focused on collaborative research with companies and the development of AI systems.
Tell me about your background.
My doctoral research was in theoretical physics. Specifically, my research focused on developing statistical models of how stem cells communicate with each other to become organs in the body. After completing his Ph.D., he started working at Deepmind as a research engineer, working on various projects, and after three years at Deepmind, he spent two years as a lead research scientist at Japanese AI startup Cogent Labs, and then in August 2020, he started working at to establish Recursive Inc. to focus on supporting AI development to achieve the SDGs.
-What kind of company is Deepmind? I have an awesome image, but what kind of company are you really?
There may be some discrepancies with the external image because AlphaGo is famous for Product application research People tend to think of us as a company that focuses on applications, but in reality, we focus on more basic research. It feels more like an academic environment compared to other companies. Our research goals are geared towards developing superhuman-level AI. It's an exciting environment with a lot of talented people.
What is your area of expertise?
For the past five years between Deepmind and Cogent Labs, my research has been focused on deep learning. In particular, I am very interested in the areas of transfer learning and Few-shot Learning (FSL). The goal of these research areas is to develop models that can learn from very small amounts of data. In other words, we can solve one of the big problems of deep learning, which is that we need a lot of data to learn.
I have also been working in the area of robustness in deep learning. Robustness here means understanding that deep learning models might make mistakes and considering how to make them safer.
What is the composition of the team for your research?
Do you have any ideas for background and skill combinations?
Deepmind's team included a scientist, an engineer, and a project manager working together. The engineers would pay particular attention to the robustness of the code, while the scientists would suggest new ideas. The project manager's role is to make sure the research project is on track and to keep the scientists and engineers from being overly distracted by a flurry of new ideas.
The team I was part of came from a variety of backgrounds: physics, mathematics, and, of course, computer science. Their approaches to problem-solving were very different, which allowed them to generate more ideas. If you call it ingenuity, maybe that's where it's at.
How do you find your research ideas? How did the project begin?
Everyone on the team was welcome to propose new research ideas. Ideas were evaluated by a research committee made up of top researchers, and if approved, a team was formed and allowed to work on the research full time. If not, the team would continue to work on the idea in their spare time until it was further refined.
How do you conduct research and project discussions?
How will the research be conducted?
As you all know, deep learning research is essentially the result of a huge amount of experimentation. This requires implementing ideas into code and testing them repeatedly on new datasets and computer environments. Often, the first version of an idea fails, and you have to modify the code or rethink the entire idea to make it work. The team would discuss progress in weekly meetings and daily discussions via Slack. The way we go about this area may not be too different from other companies.
Is there an effective way to conduct research? Were there any innovations in that regard?
One thing that helped me was that every week there were many lectures by internal and external researchers explaining their research ideas. Being exposed to information from people who were working on different things than I was has helped me generate more ideas for my own research. Also, having access to Google's data centers made it very easy to do research, as I was not limited a lot by computational power resources.
-We have an environment where it is easy to come up with ideas, and we have people with backgrounds that can come up with all kinds of ideas, which is one of our strengths.
What are some tips on how to proceed with dissertation research, how to put it all together, and what to keep in mind when doing research?
I always advise students to keep a detailed record of their ideas and experiments, because, after a month, you can forget why you got the results you did or even why you did the experiment. If you keep a record, you can refer back to it and remember what you thought and what happened.
-It's a fundamental and important element.
Another point is to do a careful literature review before you start working on a new idea. Someone else had already researched the new idea I had! This has happened many times. Also, if you get stuck in your research, I would recommend that you work backward from your goals and try to understand if there are any gaps in your knowledge. If you can recognize those gaps, then you can learn more about the topic and then come back to your research.
-Again, it's basic. It's an important factor. Although each of them is basic, it's surprising that people don't actually do them.
What do you look for when evaluating AI researchers? I would like to know if there are any items to evaluate:
I believe that good AI researchers have two capabilities that people don't think are very important.
The first is the ability to immediately experiment and confirm. As I mentioned earlier, deep learning research is essentially an experiment. If you spend too much time thinking about an idea, it may not actually work and you may waste your time. It's important to experiment quickly, so those who can write code to test their ideas quickly will get more feedback and therefore be able to improve more quickly.
The second point is the ability to understand why it didn't work. I find that it's common for experiments to go wrong, but I find that a surprising number of people give up at that point. A good AI researcher will carefully examine the code and check it over and over again to make sure everything is correct and free of bugs. If there are no bugs, it's important to intuitively try to understand where you went wrong and what happened instead.
What technology are you most interested in right now?
That's confidential information (laughs).
However, I think the progress we made in unsupervised learning last year was very exciting.
What kind of people do you think are needed for AI projects?
As we've learned at Deepmind, it takes a diverse team with a variety of backgrounds to make an AI project successful. Not only do you need a scientist, but of course you need a good engineer as well. You also need people who have a deep understanding of the challenges to get the implications of the work we're doing right.
From another perspective, the issue of bias in AI algorithms has recently received a lot of attention. I believe that having a diverse group of people working on AI algorithms will make it easier to anticipate and fix potential problems before they are implemented in the real world. A team of engineers alone will likely not be able to figure out the problems that affect society. If you bring together people from similar backgrounds, you may be missing out on opportunities to apply AI. For example, an opportunity to improve the lives of people in minority communities or areas away from major cities.
Therefore, I would like to create a research team composed of people from different educational, ethnic, and socio-economic backgrounds to evolve AI in a fair and balanced way.
I'd like to know what to expect when AI fails in society.
As AI systems become more sophisticated and integrated into our lives, we must pay more and more attention to the possibility of perpetuating biased decisions in their training data. I think the only way to mitigate this problem is to build a variety of AI applications by diverse teams, focusing on solving specific problems for specific people and listening to their voices as they develop algorithms.
As with any powerful technology, it has the potential for great benefits and great harm, and the difference comes from the responsibility of who uses it. So as pioneers in this field, we have a responsibility to educate our customers and the public about how this technology can have a positive impact on society.
-In summary, here's what it looks like: let's take a look at one reference.
Notice
Recursive is looking for collaborative research partners and interns. Please feel free to contact us via the website if you are interested.
Categories related to this article