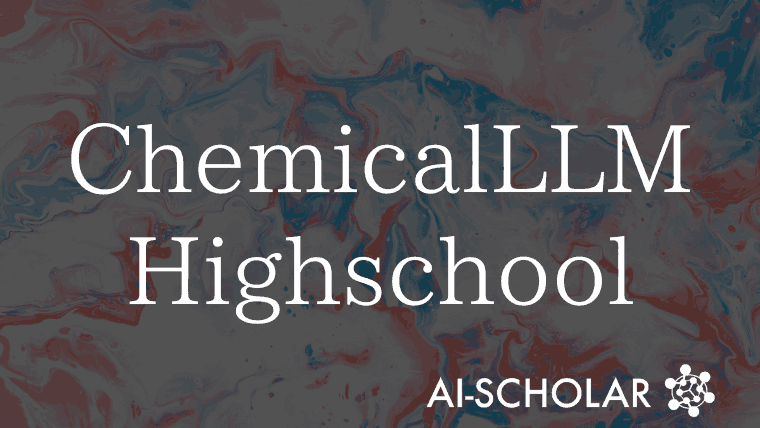
Limitations And Possibilities Of Large-Scale Language Models In Vietnamese High School Chemistry Exam Questions
3 main points
✔️ Comprehensive assessment of the performance of ChatGPT and BingChat, the most advanced language models in chemistry education at the high school level in Vietnam
✔️ Comparative analysis of ChatGPT and BingChat performance compared to Vietnamese students
✔️ Discusses potential benefits and challenges of implementing a large-scale language model in the field of chemistry education in Tonam
LLMs' Capabilities at the High School Level in Chemistry: Cases of ChatGPT and Microsoft Bing Chat
written by Dao Xuan-Quy, Le Ngoc-Bich ,Vo The-Duy ,Ngo Bac-Bien ,Phan Xuan-Dung
(Submitted on 20 Jun 2023)
Comments: Published on ChemRxiv.
Subjects: Chemical Education
The images used in this article are from the paper, the introductory slides, or were created based on them.
Summary
Artificial intelligence (AI) is playing an increasingly important role in education to enhance the student learning experience and improve teaching practices. ai-powered educational tools can provide a personalized learning experience, automate routine tasks, and provide real-time feedback and assessment They can also provide real-time feedback and assessments.
According to one study, AI is widely used in administration, teaching, and learning, and is employed in a variety of forms ranging from computer technology to humanoid robots and chatbots. and improve the quality of learning.
Another study proposes a way to automatically create video lectures with the instructor's voice and face using text-to-speech and voice-driven face technology to reduce the burden and increase learner engagement in online learning. This eliminates the need for recording and allows for easy revision. Experimental results demonstrate the effectiveness of this method.Another initiative proposes an online learning platform with a Vietnamese virtual assistant to assist the instructor in delivering lessons and evaluating learners. Lesson content is provided in the form of slides that combine synthesized audio and the instructor's face, and can be easily edited without the need for video recording.
Large-scale language models are a type of AI technology that can process and analyze vast amounts of natural language data. These models show great potential for applications as diverse as language translation, content creation, and education, including BERT, introduced by Google in 2018, RoBERTa, introduced by Facebook in 2019, T5, introduced the same year by Google researchers, and OpenAI's GPT-3 in 2020, each model has its own characteristics and has shown excellent results in natural language processing tasks.
Chemical data sets are essential for learning large language models to understand and predict various molecular properties. This is critical for drug discovery, materials design, and many other applications.Large-scale languagemodels help identify promising molecules from vast chemical spaces with high accuracy and speed.The growing interest inlarge-scale languagemodels forchemistryis increasing the need for large, diverse, high-quality chemical datasets that can provide sufficient chemical and structural information to effectively train these models. For example, MoLFormer has been trained on a SMILES sequence of 110 million unlabeled molecules and outperformed existing baselines.
As large-scale language models continue to evolve, their potential and challenges in the field of education are becoming clearer. However, in countries such as Vietnam, where Vietnamese is the primary language, itis important tocomprehensivelyassess theability of these models in order to effectively implement them in education.Particularly in the area of high school chemistry, there has been no research on this topic to date and few data sets exist to assess large-scale language models in high school chemistry.
To fill this gap, we are developing the VNHSGE Dataset, which includes data covering nine subjects from Vietnam's national examinations.The dataset contains 19,000 multiple-choice questions and 300 literature essays, both text and images.The paper is an in-depth exploration of the changes that futurelarge-scale languagemodels will bring to the field of education and its future.
Data-Set
The dataset used in this paper consists of official and practice exam questions obtained from the Vietnamese Ministry of Education and Training, high schools, and teachers. These questions were collected from examinations conducted between 2019 and 2023 and cover a wide range of subjects including mathematics, literature, English, physics, chemistry, biology, history, geography, and civic education. The questions are categorized into four difficulty levels: knowledge (easy), understanding (intermediate), application (difficult), and high application (very difficult), providing a comprehensive benchmark for assessing student ability and expertise.
In this issue, we use the Chemistry test in the Vietnam High School Graduation Examination as a benchmark.In Vietnam, the Chemistry Graduation Exam constitutes an important part of the annual high school graduation exam. The exam is classified as part of Natural Sciences and students have 40 questions and 50 minutes to solve them.
The VNHSGE dataset, built on this high school chemistry exam, contains questions of varying difficulty ranging from basic knowledge to complex problem solving requiring analysis and integration of information. To evaluate the performance of the large-scale language model,we categorized the problems into four levels asdescribed above: knowledge, comprehension, application, and high application. This approach provides a comprehensive understanding of the LLM's capabilities and limitations for a variety of issues in chemistry education.The examthenconsists of a total of 2,000 multiple-choice questions from 50 sets of exams. These questions cover a wide range of chemical areas, including metallurgy, alkali metals, alkaline earth metals, aluminum, iron, inorganic chemical synthesis, esters, lipids, amines, amino acids, proteins, carbohydrates, polymers, and polymer materials. The exam assesses knowledge of organic chemistry content, including synthesis, electrolysis, nitrogen-phosphorus chemistry, hydrocarbons, alcohols, and phenols.
The 2019-2022 Vietnam StudentScore Distribution is a way of showing the scores of examinees in a particular subject. Typically, these scores are presented in chart form, with one axis showing the score and the other axis showing the number of test takers who received that score. The chart belowshows an analysis of the 2022 National High School Graduation Examination Chemistry test results.
The mean score for the 327,370 test takers who took the chemistry exam was 6.7, with a median score of 7.0. The most common score was 8.0, with 43 test takers (0.01%) scoring below 1 and 49,900 (15.24%) scoring below the mean score. The score distribution is published annually by the Vietnamese Ministry of Education and displayed as a chart for each subject. This score distribution is used to categorize the proficiency and ability of test takers and to evaluate them based on predetermined criteria. It is also used to assess the quality of the exam based on the difficulty of the exam questions. This paper collects score distributions for the years 2019-2022. By comparing the results of the large-scale language model with the results of Vietnamese students, we can assess the proficiency of the large-scale language model.
In the VNHSGE dataset, formulas, equations, and diagrams are converted to text format in order to adapt to language models such as BERT and GPT. file in text format so that people without programming knowledge can evaluate the performance of large language models. However, symbols, tables, and images are also converted.The VNHSGE dataset is also suitable for JSON format to ensure compatibility with multiple large-scalelanguagemodels and to aid in the development of more reliable language models.JSON is ideal as input data for large-scale language models because it efficiently handles both syntactic and content-related information in text. Its flexibility and extensibility allow it to store a wide variety of textual data, including mathematical expressions, equations, tables, and images.
Here are some Vietnamese questions. We use ChatGPT and BingChat to translate the questions and answers into English. The first one is at the knowledge (EASY) level and does not require reasoning to find the answer.
The following questions are at the understanding (INTERMEDIATE) level and require a bit of reasoning to come up with an answer.
The next problem is at the applied (DIFFICULT) level and requires reasoning to find a solution.
Finally, problems at the high application (very difficult) level require deep reasoning to solve the problem.
Experiment
We evaluate the performance of ChatGPT and BingChat using the VNHSGE dataset, which includes five practice tests (total of 200 multiple-choice questions) provided by the Vietnamese Ministry of Education and Training from 2019 to 2023. Here we present the results of the evaluation of ChatGPT (February 13 version) and BingChat (March 28 version) against this sub-dataset.
Here are the ChatGPT and BingChat answers obtained from the aforementioned sample. First, for knowledge (easy) level questions, ChatGPT outputs the correct answer, while BingChat provides only partial support; BingChat does not output a solution, but does provide support that points in the direction of problem solving.
The next questionshows that ChatGPT and BingChat could not find the correct answer, although it is a common chemical reaction formula.
The applied (difficult) level questions require comprehensive knowledge to arrive at the correct answer, and both ChatGPT and BingChat have not been able to find a solution.
Neither ChatGPT nor BingChat can provide useful information on problems that require deep reasoning, and their approaches are not at all reasonable.
The order of the questions is also related to their difficulty level. The questions are categorized as follows. Questions 1-20 are at the Knowledge level, Questions 21-30 are at the Comprehension level, and Questions 31-40 are at the Applied and Highly Applied levels.The table below shows the results obtained by ChatGPT and BingChat according to the order of the questions.
In addition, it introduces two values, Max and Min, where Max represents the best-case scenario for ChatGPT and BingChat, i.e., the model's ability to provide the correct answer. Min, on the other hand, represents the worst-case scenario, i.e., the model's ability to provide the wrong answer (1-Min).
These Max and Min values can be usedto evaluate the best and worst performance oflarge languagemodelsfor the VNHSGE data set.For example, if ChatGPT provides the correct answer to question "x" and BingChat provides the incorrect answer, Max is true (value "1") and Min is false (value "0"). Since the order of the questions is linked to their difficulty level, one can evaluate the accuracy of the answers based on the question order and determine the ability of the large-scale language model based on the difficulty level of the questions.
According to the five-year average results shown in the figure below, ChatGPT is able to provide more than 50% accurate responses for questions 1-21. For questions 20-40, however, ChatGPT's correct response rate drops significantly. On the other hand, BingChat, Min, and Max provide more than 50% correct responses for questions 1-24, 1-16, and 1-27, respectively.
However, after question 24, Min's correct response rate drops to nearly zero percent; an analysis of the accuracy of the answers provided by ChatGPT and BingChat shows that both models are able to answer only knowledge and comprehension level questions and struggle with applied and high application level questions.
The table below also shows the performance of the large language models and their average values for each year; ChatGPT achieves the highest score of 62.5 in 2021 and the lowest score of 40 in 2019. On the other hand, BingChat achieves the highest score of 57.5 in 2020 and the lowest score of 47.5 in 2022. ChatGPT outperforms BingChat only in 2021.
The figure below shows the consistency of ChatGPT and BingChat responses to the VNHSGE data set. The results show that BingChat exhibits higher stability than ChatGPT. This observation is expected given that ChatGPT has a more creative approach while BingChat employs a search engine mechanism.
The figure below also compares the performance of ChatGPT and BingChat against the VNHSGE dataset with ChatGPT's performance on the AP Chemistry dataset provided by OpenAI; OpenAI reports that ChatGPT achieves a score range of 22% to 46% on the AP Chemistry dataset, achieving a score range of 22% to 46%. On the VNHSGE dataset, on the other hand, ChatGPT and BingChat score 48% and 52.5%, respectively, achieving a maximum score of 67.5% and a minimum score of 33% in the test cases by this paper.
Comparisons were also made with Vietnamese students.To evaluate the performance of the large-scale language model, we compared its results with those of Vietnamese students. The table below shows the ChatGPT and BingChat conversion scores, the average score (AVNS), and the score of the best Vietnamese student (MVNS).
The average scores for ChatGPT, BingChat, Min, and Max are 4.8, 5.25, 3.3, and 6.75, respectively; the average scores for Vietnamese students in 2019-2022 are 5.35, 6.71, 6.63, and 6.7, respectively. This indicates that the ChatGPT and BingChat scores are lower than the average score of Vietnamese students. However, Max shows better results than the average score of Vietnamese students, but not as good as the score of the best Vietnamese students.
The chart below compares the scores of ChatGPT, BingChat, Min, and Max with those of Vietnamese students. The graph further highlights that the performance of ChatGPT and BingChat in high school chemistry is inferior to that of Vietnamese students.
Summary
To evaluate large-scale language models in high school chemistry,this paperdevelops the VNHSGE dataset, which includes data covering nine subjects from Vietnam's national highschool chemistry examinations from 2019 to 2023. We then evaluate the performance of ChatGPT and BingChat. Results show that both modelshave limited performance onapplied (DIFFICULT) and high application level(VERY DIFFICULT) problems, with deficiencies in reasoning and knowledge application. In addition, a comparison of ChatGPT and BingChat shows that BingChat is generally more accurate. Comparing the scores of both models with those of Vietnamese students, the scores of the large-scale language model are lower than the average student scores, indicating that there are limitations in replacing human intelligence in chemistry education.
Nevertheless,large-scale languagemodels have the potential to support educational activities, such as providing students and teachers with immediate feedback and personalized learning experiences.In addition, they could help generatequestions andmaterials forpractice and assessment.Large-scale languagemodels could be further improved by incorporating more specialized knowledge and enhancing reasoning and application skills. Overall,large-scale languagemodels show promise in the field of education, but there are still challenges to overcome. Future researchis expected to investigate ways to improve the reasoning and knowledge application capabilities oflarge-scale languagemodels and their effectiveness in improving student learning outcomes.
Categories related to this article