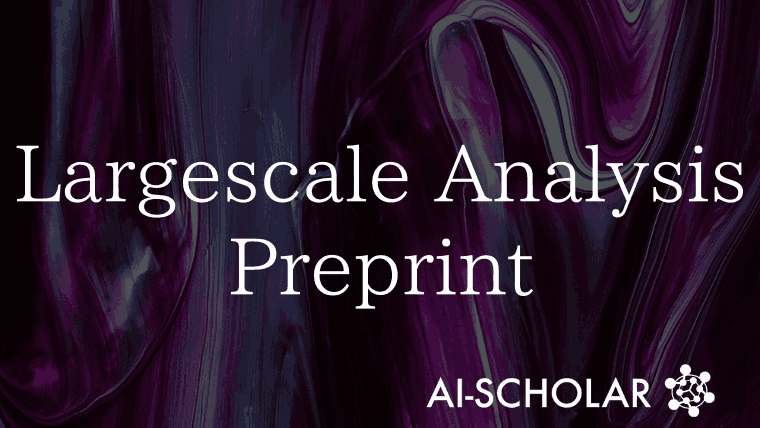
Use And Impact Of ChatGPT In Scientific Articles, Analysis By Binoculars
3 main points
✔️ Use of large-scale language models in scientific papers surges after ChatGPT release
✔️ Analysis using Binoculars scores confirms increase in detection of generated text and citations
✔️ Explicit usage bias by discipline and country and diversity of impact on content types
Have AI-Generated Texts from LLM Infiltrated the Realm of Scientific Writing? A Large-Scale Analysis of Preprint Platforms
written by Huzi Cheng, Bin Sheng, Aaron Lee, Varun Chaudary, Atanas G. Atanasov, Nan Liu, Yue Qiu, Tien Yin Wong, Yih-Chung Tham, Yingfeng Zheng
(Submitted on 30 Mrr 2024)
Comments: Published on bioRxiv.
Subjects: Scientific Communication and Education
The images used in this article are from the paper, the introductory slides, or were created based on them.
Summary
Advances in AI technology are transforming the landscape of digital content production and consumption. Of particular note isthe rapid evolution ofgenerative AI, includinglarge-scale language models, such as ChatGPT, a large-scale language model based on GPT-3 that emerged in 2022 and is capable of generating text of a quality very close to that of human text. These modelsare widely used in content creation because theycan freely generate text that takes into account usage, sentence tone, and context.
At the same time, however, this proliferation has raised concerns about the reliability, originality, and quality of content generated by large-scale language models. Andthe issue of information overload caused by the rapid generation of large amounts of content by these technologies is also being discussed.
As large-scale language models become more prevalent in the scientific community, their use in scientific papers is a natural progression.Scientific papers are held to strict standards for accuracy, clarity, and conciseness, and large-scale language models are expected to assist in these tasks. However, the human inquiry, insight, observations, and reflections that are essential to scientific papers are difficult to achieve with current large-scale language models. It can be said that scientific papers are truly at a crossroads in their use with large-scale language models.
This paper investigates the current state of large-scale language modeling in the scientific literature, particularly in the area of preprint articles. Utilizing large open datasets and advanced detection tools such as Binoculars LLM-detector, the paper provides a complete picture of the impact of large-scale language models on scientific articles. The research in this paper spans a variety of disciplines and correlates the surge in content generated by large-scale language models, search trends, discipline-specific impacts, and author demographics.
It also investigates the relationship between the use of large-scale language models and the impact of papers, andshows thatthe use of large-scale language modelsis positively correlated with the number of citations. The paper provides insight into how large-scale language models are changing the conventions of scientific writing and makes recommendations for the safe use of large-scale language models in academic research.
Methodology and Data Sets
Publishing a paper takes time, sometimes more than a year. On the other hand, large-scale language model-based text generation tools such as ChatGPT have been rapidly gaining popularity since the end of 2022. Since it is difficult to analyze the impact of scale language models in the formally published literature in a short period of time, this paper analyzes papers submitted to preprint platforms.
The preprint platform allows authors to be the first to know the latest research results, as many authors upload preprint versions of their papers before submitting them to journals. In addition, many papers are submitted even in a short period of time, allowing for in-depth analysis. In addition, the preprint platform can be accessed in large volumes, allowing for large-scale analysis.
This paper collects articles in PDF format from three major preprint platforms: arXiv, bioRxiv, and medRxiv.
These cover a wide range of disciplines from mathematics and engineering to biology and medicine. Manuscripts from all platforms are downloaded from January 1, 2022 to March 1, 2024. This period includes one year before and after the ChatGPT release in December 2022.
Up to 1000 random papers/month from each platform are downloaded using the API. After cleaning and preprocessing, invalid documents are removed, and finally 45,129 papers are used for analysis. These papers fall into the following areas: biological sciences, computer science, economics and finance, engineering, environmental sciences, mathematics, medicine, neuroscience, and physical sciences.We also use data from Google Trends to study the impact and usage of ChatGPT. We collect and analyze daily and weekly Google Trends data for the keyword "ChatGPT" worldwide.
Experimental Results
Texts generated by conventional LSTMs and GRUs were easily distinguishable and often unnatural, so they had not yet reached the practical stage. However, since the reporting of transformer-based models and the construction of large-scale language models, the text generatedhas becomeindistinguishable from that produced by humans, making itsdetection much more difficult. In particular, with the release of ChatGPT at the end of 2022, detection has become even more difficult.In this situation, detectors that take advantage of hidden statistical patterns are needed to distinguish text generated by large-scale language models. These detectors donot requireknowledge of a specific large-scale language model andrequirelittletraining.
A commonmethod is to analyze the perplexity of a given text. This method is based on the idea that texts generated by large-scale language models generally have lower perplexity. However, this is only valid for texts generated by large-scale language models alone. In the case of scientific papers, authors are likely to use large-scale language models for content revision, rather than using large-scale language models to generate the entire paper.
A tooldevelopedspecifically for this problem is the Binoculars score: a high Binoculars score indicates that the text is likely to have been generated by a human, while a score below a certain threshold indicates that the content is likely to contain text generated by a large-scale language model.By using not one, but two large-scale language models, Binoculars is able to detect text that may contain mixed prompts. This feature allows Binoculars to outperform other large-scale language model detectors such as Ghostbuster, GPTZero2, and DetectGPT in many benchmark tests. We use Binoculars as our primary detector in this paper.
Because the papers are long for a single pass through the Binoculars detector, each paper is split into chunks of equal size, and each chunk is input to the Binoculars detector. Traces of the large-scale language model within a paper are a sequence of corresponding Binoculars scores. We see that the mean, variance, and minimum of this sequence are important for detecting the generated text in this paper.We computethe mean, variance, and minimum of theBinocularsscore on a per-paper basis for all papers in the datasetand use a 30-day moving average of these three scores to compute the three Binoculars scores for the years 2022 through 2024. Thisassumes thatthe current use of ChatGPTwill take time to be reflected in thepapersbeing submitted, as papers take a relatively long time to be published.
These three indicators are then compared to the weekly Google trends for the keyword "ChatGPT". This is used to indirectly measure the usage and popularity of the large-scale language model in writing. The gray line in the figure below shows that the search trend for ChatGPT has increased since its release on November 30, 2022.
The trend shows that the three Binoculars scores correlate with the trend:the mean and minimumBinoculars scoresare higher before the release of ChatGPT, and the variance is higher after the release.The decrease in the mean Binoculars scoreindicates an overall increase in content containing ChatGPT-generated text. The decrease in the average Binoculars score indicates an overall increase in content containing ChatGPT-generated text.
We also examine whether this relationship holds true at finer time scales. Similarly, we compare Google Trends on a daily basis to Binoculars scores at the same resolution. However, we limit ourselves to the period after the release of ChatGPT. The results in the figure above show that this correlation persists and is consistent with the weekly unit analysis. A closer look at the significance of the correlation reveals that the minimum and variance are more dominant compared to the mean of the Binoculars score.
Next, we investigate the differences in the utilization of ChatGPT in different domains.Based on the results in the figure below, we are examining how the utilization of ChatGPT and other large-scale language models differs across different domains. Several factors may influence this. For example,bias in the distribution of data used to trainlarge-scale languagemodels may result in differences in performance in different domains. Domains that make heavy use of abstract descriptions and highly contextualized symbols, such as mathematics, may have difficulty using ChatGPT directly. Reliance on and affinity for modern digital tools may also affect the use of large-scale language models. For example, the computer science domain may be more open to integrating ChatGPT into their workflow.
The experiment classifies all papers into several domainsandanalyzes the distribution ofmean and minimumBinoculars scores before and after the ChatGPT release.
The figure below also shows that the minimum Binoculars values dropped significantly after the release of ChatGPT in the Biological Sciences, Computer Science, and Engineering domains, suggesting that ChatGPT is being used more. In particular, theaverageBinoculars scorealsodropped significantly in theEngineering and Computer Science domains.This trend may be attributed to the abundance of data in these domains in the ChatGPT study data. In all other domains, themean or minimumBinoculars score alsodeclined, confirming the widespread use of ChatGPT.
We also investigate differences in the use of ChatGPT by country and languageAnother important factor influencing the use of ChatGPT is likely to be the native language spoken by the authors of the articles. Since many articles are published in English, it is likely that authors whose second language is English will rely on ChatGPT. However, it is difficult to analyze this directly because we do not have all the data on authors' nationalities and native languages. Therefore, we devised an alternative for each platform and assigned a country/region to each manuscript in the dataset. The eight countries with the highest number of submissions were selected for analysis, and the other countries/regions were assigned as "other.
Similar to the figure below,we analyze the distribution ofmean and minimumBinoculars scores before and after the ChatGPT release.
The figure below also shows that almost all countries experienced a decrease in the minimum Binoculars score and a decrease in the mean Binoculars score, but not significantly. In particular, countries such as China, Italy, and Indiashow larger differences inmean and minimumBinoculars scores after the release of ChatGPT. This may be related to the fact that the native language of these countries does not include English.
To test this hypothesis, we categorize each country/region by official language. Results show that while Binoculars scores have decreased in all countries/regions since the release of ChatGPT, the overall level of average and minimum Binoculars values is still higher in countries/regions where English is one of the official languages. This finding is consistent with several previous studies that found that texts written by non-native English speakers are more likely to be recognized as LLM-generated.
These experimental results indicate that the use of ChatGPT varies by domain and country/language. In particular, we found that its use was more pronounced in certain domains and among authors for whom English is a second language.
We are also investigating the impact of content type.We are examining how content types are affected by text generated by large-scale language models. Intuitively, content that contains a lot of existing information or introduces past discoveriesis likely to be influenced by thelarge-scale languagemodel. On the other hand, content that is specific or about new discoveriesmay not be suitable for generation by alarge-scale language model.To test this, we used an NLI-based zero-shot text classification model toclassifyeach articleinto10 content types (description of phenomenon, formulation of hypothesis, description of methodology, presentation of data, logical reasoning, interpretation of results, literature review, comparative analysis, summary of conclusions, and suggestions for future research).
First, in the left panel below, we check to see if the distribution of content types is stable between texts with high Binoculars scores and texts with low Binoculars scores. The texts are divided into two sets based on the average score for the entire data set (1.02). The results show that literature reviewshave very lowBinocularsscores, while data presentations containing new information and descriptions of phenomena have the highest scores. The distribution of content types in the high and low scoring collections is relatively stable, with small percentage fluctuations.
Next, we examine the differences in Binoculars scores for each content type before and after the ChatGPT release. As can be seen in the right-hand side of the figure above, most content types saw a decrease in scores, but there is no significant decrease in the literature review. There is a large drop in scores for content considered new, such as hypothesis formulation, summary of conclusions, description of phenomena, and future research proposals.
Finally, we investigate the relationship between Binoculars scores and the influence of papers.We also investigate the potential for content quality to be "contaminated" by the use oflarge-scale languagemodels. Since this assessment is subjective, we use citation counts as a measure of a paper's influence: we use Semantic Scholar's API to collect citation counts for nearly all papers in our dataset and compare the correlation between the average Binoculars score and citation counts before and after the ChatGPT release. The results showed that the correlation between the average Binoculars score and the number of citations before and after the release of ChatGPT was significantly higher than that of the average Binoculars score. The results show that the correlation was not significant before the ChatGPT release (0.004214, p=0.56), but after the release the correlation changed to -0.018911 with a p-value of 0.002566. This change in correlation is significant (p-value=0.007994), suggesting that the more ChatGPT is used (the lower the average Binoculars score), the more likely it is to increase citations.
The results of these experiments show how the influence of text generated by large-scale language models manifests itself in content type and article influence. In particular, a trend toward increased citations has been observed after the release of ChatGPT.
Summary
An analysis of approximately 45,000 papers submitted to thethree preprint platforms (arXiv, bioRxiv, and medRxiv) over the past two yearsreveals a significant increase in the use of large-scale language models in scientific papers after the release of ChatGPT at the end of 2022 The results show that the use of large-scale language models in scientific papers increased significantly after the release of ChatGPT at the end of 2022.
By examining the Binoculars score statistics for each paper,we found thatafter November 30, 2022,the averageBinoculars scoredropped significantly, and that this drop correlates with the Google Trends data for the keyword "ChatGPT". This is indicative of the widespread presence of text generated by large-scale language models in scientific papers. Furthermore, the study also revealed a bias toward the use of large-scale language models in various fields and countries. In particular,the use oflarge-scale languagemodels is high inthe fields of computer science and engineering, and similar trends are observed in countries where English is not the official language.The impact of large-scale language models oncontent typesis also skewed, with texts containing new information showing a greater decrease in Binoculars scores than literature reviews.
Analysis of the monthly correlations betweenaverageBinoculars scoresandcitation counts also revealed an unexpected reversal of trends: before the release of ChatGPT, the correlations were weak and negligible; after the release, the correlations turned negative, indicating that papers containing text generated bythe large-scale language modelpapers containing text generated by the large-scale language model are more likely to be cited.
However, there are several challenges to this effort. First, it is not possible to completely determine whether a text was generated by a large-scale language model; the Binoculars score relies on statistical patterns common to texts generated by large-scale language models, which can be compromised by improper use, for example. In addition, the Binoculars score is not a reliable indicator of the quality of the text. Other statistical tools, such as zero-shot text classification models, can also commit similar errors. Second, while many authors tend to upload their papers to preprint platforms, these platforms do not cover all scientific papers, and different disciplines have different tendencies to use preprints. Therefore, the datasets used do not give a complete picture. Furthermore, due to limitations of platforms such as the arXiv, we do not have direct access to information on authors' country/region/native language. The introduction of a nationality estimation service may result in errors in certain papers. Also, as mentioned above, papers may include contributions from people who speak different languages, which may lead to inaccurate country/region analysis.
Despite these challenges, however, this paper is the first attempt to identify quantitatively and on a large scale the impact of large-scale language models on the writing of current scientific papers.
Categories related to this article