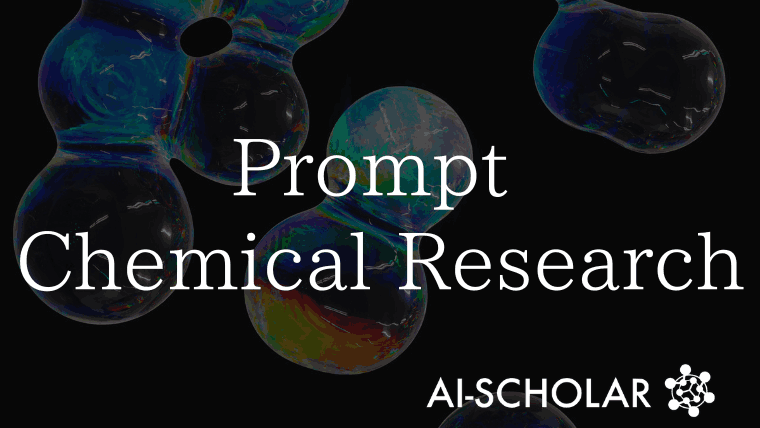
What Does The "GPT-4" Demonstrate About Chemistry Knowledge And Problem-solving Skills?
3 main points
✔️ Advanced problem solving in a wide range of fields from chemical bonding to organic and physical chemistry
✔️ Few-shot learning suggests the ability to rapidly acquire and apply new knowledge, even in chemistry
✔️ Still leaves some work to be done with respect to specific synthetic method descriptions and up-to-date scientific article-level expertise
Prompt engineering of GPT-4 for chemical research: what can/cannot be done?
written by Kan Hatakeyama-Sato, Naoki Yamane ,Yasuhiko Igarashi ,Yuta Nabae ,Teruaki Hayakawa
(Submitted on 5 June 2023)
Comments: Published on ChemRxiv.
Subjects: Theoretical and Computational Chemistry
The images used in this article are from the paper, the introductory slides, or were created based on them.
Summary
Recent advances in artificial intelligence have focused attention on large-scale language models such as GPT-4. released in March 2023, this advanced model demonstrates the ability to leverage a wide range of knowledge to address complex challenges, from chemistry research to everyday problem solving. GPT-4 has been used in science also begun to be studied, providing deep insights into all areas of chemistry, from chemical bonding to organic chemistry to physical chemistry. The model can predict potential new compounds and reactions based on existing knowledge, and can also be linked to external environments to extend its capabilities through web searches and programming languages.
GPT-4 learns from a vast amount of textual data, and its inference capability grows exponentially with the size of the training data set and model. The model also excels in a technique called "fuchot learning," which makes logical inferences from even small amounts of data. It also has the ability to come up with and perform its own tasks, such as playing games like Minecraft, without special learning.
However, it has also been pointed out that the performance of the supercomputers used for GPT-4 training has already reached the world's top level, and further rapid upgrades may be difficult. Therefore, how to utilize GPT-4 level language models will be an important issue in the next few years.
This paper assesses how the GPT-4 can be used in the field of chemistry through a number of simple tasks that will help you to understand the capabilities and challenges of the GPT-4. These include understanding basic knowledge, handling molecular data in informatics, data analysis skills, and the ability to make predictions and suggestions for chemical problems.
It also discusses the contributions of GPT-4 to chemistry research and the challenges that need to be overcome. The results of this study also aim to share prompt engineering methods for chemistry tasks and discuss future prospects for chemistry research using large-scale language models.
Large-scale language models have chemical knowledge
The experiments conducted in this paper use ChatGPT (May 24, 2023 version ) as the large-scale language model. As a large-scale language model, we alsouse GPT-4 under the condition that it does not refer to external data through plug-ins. Furthermore, to avoid referencing past conversation logs, we alwaysreason in new conversations unless otherwise specified. We ask a question only once and use the response. The full conversation is included in the paper as supplemental information, if you are interested.
We begin byexamining how much GPT-4 knows about compounds. In chemistry, the most basic and elementary questions are often about the properties of compounds. it is important to know how well GPT-4 understands this basic knowledge. GPT-4 has shown remarkable performance in this regard. For example, as shown in the figure below, the GPT-4 is able to accurately understand and describe the physical and chemical properties - molecular weight, melting point, boiling point, aroma, chemical stability, and reactivity - of the widely used industrial raw material toluene (chemical formula C7H8). This knowledge is likely to have been acquired by GPT-4 from learning from common chemistry textbooks and websites.
It also addresses a slightly more specialized level of knowledge that is not found in textbooks. For example, for the organic compound 2,2,6,6-tetramethylpiperidine 1-oxyl (TEMPO), an organic compound used as a radical trapping agent, spin label, electrochemical catalyst, and electrode active material, "What is its redox potential?" the GPT-4 accurately answers "about +0.5 V (relative to a standard hydrogen electrode)," as shown in the figure below.
However, limitations have also been observed. For example, the model does not provide information on the redox potential of 4-cyano TEMPO, a derivative of TEMPO. This suggests that certain chemical articles and academic papers are not included in the model's training data. Many of the scientific articles are protected by copyright, which restricts their free access and use, and may not be within the scope of the AI's training.
This situation will require chemists to actively contribute more information for AI to learn through openly accessible papers and preprints.
Next, we also examine the GPT-4'sknowledge of physical chemistry. Physical chemistry lies at the interface between chemistry and physics, and its complexity makes it difficult to understand. However, it is clear that the GPT-4 has a college-level understanding of the basic concepts in this field - such as the ideal gas law and the Lorentz-Lorentz equation for the refractive index of matter. This knowledge is believed to have been acquired through learning from textbooks.
As shown in the figure below, GPT-4 is well versed in graduate-level content such as the Vogel-Fulcher-Tamann (VFT) equation, which describes how the viscosity and structural relaxation time of a supercooled liquid depend on temperature and is important for understanding glass transition phenomena. The equation provided by GPT-4, 𝜂 = 𝜂0exp(𝐵/(𝑇 - 𝑇0)), indicates that viscosity depends on temperature, and 𝑇0 (Vogel temperature) represents the temperature at which relaxation time or viscosity becomes infinite.
However, GPT-4 also has its limitations. In particular, it does not have the expertise at the level of an academic paper, such as the empirical rule (𝑇g = 𝑇0 + 50) reported in the 1980s that relates the Vogel temperature 𝑇0 to the glass transition temperature 𝑇g within a polymer. This indicates that GPT-4 is based on knowledge up to September 2021 and does not cover the most recent research due to copyright issues with academic papers.
Organic chemistry is also examined, and GPT-4 is shown to have mastered basic textbook-level knowledge in this area. For example, the figure below shows that GPT-4understands the description of the synthetic pathway for acetaminophen - a process that starts with phenol, followed by nitration, reduction with tin, and amidation with acetic anhydride to obtain the desired compound.
However, we were unable to answer specific questions about the experimental procedure. In response to questions such as, "Can you tell me how to synthesize acetaminophen?" the company responded, "We are sorry, but we cannot assist you with that."This is likely due to safety considerations in order to avoid the risk of accidental dissemination of knowledge of chemical experiments, taking into account their social impact.
In addition, GPT-4 challenged the students on applied organic synthesis questions, but some answers showed chemical misunderstandings. For example, a question on the synthesis of TEMPO once suggested an incorrect chemical reaction process. In reality, the correct process is to use acetone and ammonia as starting materials and synthesize TEMPO via aldol condensation, reduction with hydrazine, and elimination reaction, but GPT-4's description omitted an important part of this process.
Furthermore, GPT-4 suggested the need for a chemically inappropriate oxidation reaction in the final step of TEMPO synthesis. In fact, TEMPO can be obtained by one-electron oxidation of TMP, but GPT-4 incorrectly asserted that an excessive oxidation reaction is required. This demonstrates a current limitation in AI's chemical knowledge and leaves room for further improvement.
Chemical Informatics and Materials Informatics with Large-Scale Linguistic Models
Chemical informatics and materials informatics are fields that utilize data science to elucidate correlations between chemical structures and their properties. Expectations for GPT-4 in chemical informatics are very high. This is because the field of chemistry and indeed research activities are often described and processed through language, even though chemical informatics has not been able to adequately handle linguistic data in the past. Here we examine the extent to which GPT-4 can address the fundamental problems associated with chemical informatics.
In the field of chemical informatics, SMILES (Simplified Molecular Input Line Entry System) notation is widely used to represent structures in organic chemistry. The ability to understand and use this complex notation is one of the key skills in the field of chemical informatics. Here, we test the extent to which GPT-4, a state-of-the-art language model, can perform conversions between compound names and SMILES notation.
Experimental results show that GPT-4 is able to accurately convert compound names to SMILES notation for relatively simple structures such as toluene.However, when it comes to somewhat more complex structures such as p-chlorostyrene, TMP, and 4-cyano TEMPO, the model fails to convert. Furthermore, in the SMILES to compound name inverse conversion task, failure was observed in all cases. This suggests that GPT-4 can only handle SMILES and molecular structure transformations at the basic level.These results clearly demonstrate the limitations of GPT-4 and other language models, especially in understanding and processing complex chemical structures. At this time, algorithm-based conversion tools such as ChemDraw and specialized LLMs are considered more suitable for more accurate and systematic tasks.
Inference problemsareanotherpromising application for GPT-4. As a specific example, it asks why the order in which the potentials of three nitroxide radicals - TEMPO, 4-oxo-TEMPO, and 1-hydroxy-2,2,5,5-tetramethyl-2,5-dihydro-1H-pyrrol-3-carboxylic acid - increase. GPT-4 correctly points out that the order in which the TEMPO and 4-oxo It correctly points out that the electron-attracting carbonyl group between TEMPO is responsible for the difference in potential, but its reasoning as to why the 1-hydroxy compound has the highest potential is inaccurate. This is due to our inability to accurately infer molecular structure from compound names. Future studies should further explore the accuracy of inference when GPT-4 accurately recognizes molecular structures.
Another feature of GPT-4 is its ability to perform few-shot learning. This allows GPT-4 to learn about an unknown compound and predict its properties from a limited amount of data. For example, based on the redox potential of TEMPO, we were able to accurately predict the potential of its cyano derivative. This prediction is in agreement with experimental results and is a remarkable result for conventional chemical informatics, demonstrating GPT-4's ability to predict potentials using one-shot learning without the need for laborious and time-consuming large amounts of data collection and analysis. The results demonstrate GPT-4's ability to effectively use chemical data and related information as explanatory variables.
Thus, GPT-4 has the potential to innovate in the tasks of inference and property prediction in chemistry.
Furthermore, GPT-4, equipped with some reasoning capability, can be considered an AI that can be studied autonomously by skillfully combining and improving upon the methodologies that have been discussed. For example, GPT-4 can make decisions and take actions autonomously within the virtual world of the game Minecraft.It may not be long before this technology is applied to research tasks in physical space.
While previous research required a human to narrow down the scope of the search, GPT-4 can move freely within the language space and automate various aspects of research, from searching the literature to setting experimental conditions and reporting results. Research is being conducted on open source projects such as AutoGPT, which is aimed at automating tasks such as code execution.As an example of research, if a chemist wants to understand the relationship between chemical structure and density, GPT-4 can generate a "chemist" object with skills in chemical analysis and density measurement, and collect relevant data from the Internet. With these advances, it is becoming a reality that large-scale language models can learn and execute research methodologies.
However, there are still challenges for GPT-4 to reach a level comparable to human researchers, such as solving sophisticated mathematical problems. There are limitations in the ability to solve long-term planning problems, and gaps exist in autonomously refining research topics, designing experiments, and writing papers.
The development of these technologies has the potential to revolutionize the future of research. Autonomous research with large-scale language models has only just begun, and further advances are expected in the future.
Summary
This paper demonstrates that GPT-4demonstrates a variety of capabilities in a wide range of tasks in chemical research, from organic chemistry to automated arm control for experiments. In particular, while GPT-4 showed a deep understanding of general organic chemistry, it still showed some challenges with specialized content, such as specific synthetic methods. We also found that the conversion of compound names into SMILES notation is a popular method in the field of chemoinformatics, and while it performed well on some tasks, some results suggest that the lack of training data may have limited its performance.
However, it has been shown that accurate predictions can be made for unlearned compounds through fuchsot learning. This demonstrates the high performance of GPT-4 in learning and applying new knowledge from limited data. Specific applications have also been found, such as using domain knowledge in chemistry to set initial conditions for data exploration.
In general, the results reveal that while GPT-4 is capable of handling a wide range of tasks in chemical research, its performance depends on the quality and quantity of training data, and that improving its inferential capabilities is a future challenge. Exploring ways to efficiently apply the evolving GPT-4 to chemical research and developing hybrid models that combine it with existing expertise are suggested as future directions.
Categories related to this article