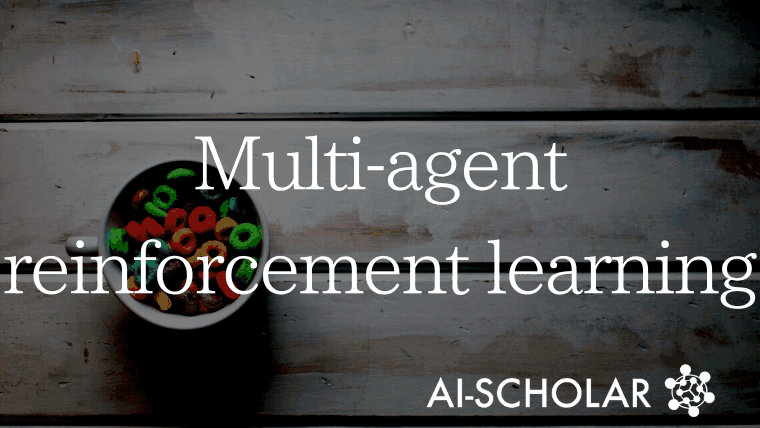
Multi-agent Reinforcement Learning Algorithm That Can Handle Increasing Or Decreasing Number Of Agents
3 main points
✔️ Proposed a multi-agent reinforcement learning algorithm "MA-POCA" that can handle increasing and decreasing the number of agents in the environment.
✔️ Support variable-length input to Critic by using Attention
✔️ Significantly outperforms existing methods on tasks where agents are created and destroyed in an episode and on standard multi-agent coordination tasks
On the Use and Misuse of Absorbing States in Multi-agent Reinforcement Learning
written by Andrew Cohen, Ervin Teng, Vincent-Pierre Berges, Ruo-Ping Dong, Hunter Henry, Marwan Mattar, Alexander Zook, Sujoy Ganguly
(Submitted on 10 Nov 2021 (v1), last revised 7 Jun 2022 (this version, v2))
Comments: AAAI 2022
Subjects: Machine Learning (cs.LG); Artificial Intelligence (cs.AI)
code:![]()
The images used in this article are from the paper, the introductory slides, or were created based on them.
In many real-world scenarios, agents need to cooperate to achieve a common goal. In these settings, single-agent reinforcement learning (RL) methods may exhibit suboptimal performance or worse as the number of agents increases. Multi-agent reinforcement learning (MARL) methods address these issues by using centralized training and decentralized execution. In this, the agents act using local observations, but globally available information is used during training.
So far, existing MARL methods have assumed that the number of agents in the environment is fixed for training. However, this is often unsuitable for many practical applications of MARL. For example, agents in a team-based video game may "spawn" (i.e., be created) or "die" (i.e., disappear before other agents) within a single episode. Also, in addition to games, any of the robots working in a team may run out of battery power and terminate before their teammates (i.e., other robots). In general, existing algorithms handle such situations by placing inactive agents in an absorbing state.
*Absorption state: A state where once you enter, you cannot leave (like the rightmost or leftmost state in the figure below).
Agents remain absorbing until the entire group of agents reaches the termination condition, regardless of their choice of action. While the absorbing state allows learning to occur with a fixed number of inputs to the Critic, it can also be viewed as a waste of information, which becomes more pronounced as the number of agents increases.
The key challenge posed by premature termination of agents is what we call Posthumous Credit Assignment. Agents who are excluded from the environment cannot experience the rewards given to the group after early termination, and thus cannot know whether their actions before termination were valuable to the group. To solve this problem, we propose a MARL algorithm that propagates value even if the agent terminates early. Specifically, for the existing MARL algorithm COunterfactual Multi-Agent Policy Gradients (COMA ), we propose a novel architecture Multi-Agent We proposed a novel architecture Multi-Agent POsthumous Credit Assignment (MA-POCA ), which uses Attention instead of a full-coupling layer with absorbing states, within the framework of centralized training and decentralized execution. The attention mechanism can be extended to an arbitrary number of agents by applying it only to the active agent information before input to the Critic.
First, let's talk about distributed - partially observed Markov decision processes. This is a multi-agent extension of the partial observation Markov decision process familiar with single-agent reinforcement learning. The symbolic definitions used are as follows.
- Number of agents N( ≥ 1)
- State space of environment S
-
:= $O_1$ × ... × $O_N$
COunterfactual Multi-Agent Policy Gradients (COMA) which was introduced in a paper proposing the MARL method called "COunterfactual Multi-Agent Policy Gradients (COMA)". The baseline is introduced so that the advantage function reflects "how much an individual agent contributed to the shared reward (group reward)". Specifically, we use a state action-value function that marginalizes the actions of individual agents (*As the name counterfactual implies, we are calculating the state action value of an agent "if it had taken a different action from the one it took") and
, and the advantage function
the gradient of agent i's measure
is calculated. This allows us to calculate how much each agent has contributed to the group's shared reward.
Posthumous Credit Assignment
, which addressing the problem of posthumous credit assignment without using states. This approach addresses the problem of posthumous credit assignment.
the output of value function) is
The following is the result. where $g_i(o^i_t)_{1\leq i \leq k_t}$ is the encode observations of all active agents and RSA is ResidualSelfAttention. Then, we define the objective function as
The training will be performed as follows. $k_{t+n}$ is the number of agents active at time t+n. The $k_{t+n}$ can be larger or smaller than $k_{t}$. This is because at time step t, any number of agents may be terminated early or new agents may be created.
We learn the Here, we consider observations and observation-action pairs as separate entities and, as in the Critic's update, we use RSA blocks and observation and observation-action encoders to learn the baseline of agent j as
then the advantage function of agent j is defined to be
$y^{(\lambda)}$ is the same as the one used to update Critic.
) MA-POCA is proposed. In conventional MARL, this problem is handled by applying an absorbing state to agents that terminate early. MA-POCA, however, can train agents without using an absorbing state by using Attention. In our experiments, we demonstrated that MA-POCA outperforms COMA and PPO on the MARL task. In the future, we plan to investigate possible forms of other algorithms in the distributed POMDP framework for problems where the maximum number of agents N is unknown.
Categories related to this article