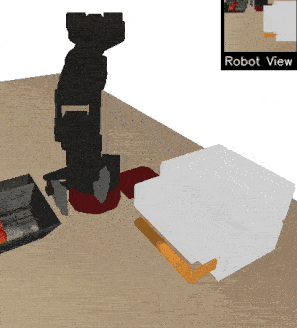
COG: A Framework For Learning Versatile Robots Using Past Experience!
3 main points
✔️ Proposed COG to learn more generic policies using Prior data and Task data
✔️ Propose a simple and effective method using Offline RL
✔️ Achieves higher task success rate compared to other methods
COG: Connecting New Skills to Past Experience with Offline Reinforcement Learning
written by Avi Singh, Albert Yu, Jonathan Yang, Jesse Zhang, Aviral Kumar, Sergey Levine
(Submitted on 27 Oct 2020)
Comments: Accepted to CoRL2020, Source code and videos available at this https URL
Subjects: Machine Learning (cs.LG); Robotics (cs.RO)![]()
![]()
![]()
first of all
In this article, we introduce a paper titled "COG: Connecting New Skills to Past Experience with Offline Reinforcement Learning". the problem with RL (reinforcement learning) is its lack of versatility. For example, if a robot learns a task to retrieve an object from a drawer, and the drawer is open, it will not be able to solve the task if the drawer is closed at test time. If we could learn policies for various cases, we might be able to solve these problems, but this would increase the learning cost. In this paper, we focus on the question of whether the robot can open a drawer and take out an object even if the drawer is closed at the time of the test if there is interaction data collected in the past. This is the focus of this paper. In this article, I will show how offline RL can combine large amounts of historical data with task-specific data to learn a more general policy, a method called Connecting Skills via Offline RL for Generalization (COG) This presentation will introduce
To read more,
Please register with AI-SCHOLAR.
ORCategories related to this article