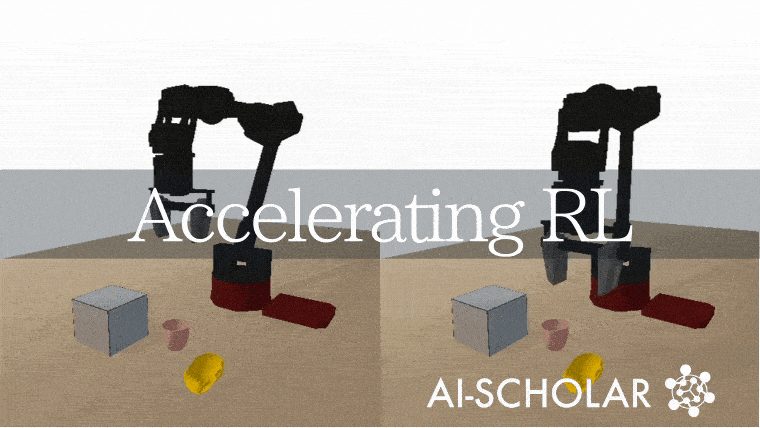
Parrot: Improving Sample Efficiency For Reinforcement Learning Through Prior Learning With Diverse Data
3 main points
✔️ Proposed PARROT to accelerate learning of RL by learning Behavioral Prior from diverse datasets
✔️ Learning Behavioral Prior enables more efficient exploration
✔️ Higher task success rates in samples with fewer PARROTs compared to the baseline
PARROT: Data-Driven Behavioral Priors for Reinforcement Learning
written by Avi Singh, Huihan Liu, Gaoyue Zhou, Albert Yu, Nicholas Rhinehart, Sergey Levine
(Submitted on 19 Nov 2020)
Comments: Accepted at arXiv Under review as a conference paper at ICLR2021
Subjects: Machine Learning (cs.LG); Robotics (cs.RO)![]()
![]()
![]()
Introduction.
In this article, we present the paper "Parrot: Data-Driven Behavioral Priors For Reinforcement Learning". The problem with reinforcement learning is that when given a new task, you have to collect a large number of samples on that task. However, for natural language processing and image tasks, pre-training on large datasets allows the data about the new task to be learned at least effectively. Therefore, in this paper, can we perform equally effective pretraining in reinforcement learning? and proposed a method called Prior AcceleRated ReinfOrcemenT (PARROT).
So, what kind of representation is effective for reinforcement learning? This is because, when you are given a new task, the representation is
- Giving you an effective search strategy.
- Simplify the learning of policy
- Allow full control of RL agent to the environment
In this paper, we list the following. To overcome these challenges, this paper performs a mapping from noise vectors to high-dimensional action space, the Trains the invertible function. By learning this invertible function, the original MDP (Markov Decision Process) can be converted to a simpler MDP when the structure of the original MDP is covered by the structure of the MDP included in the training data, and the training can be trained to It can be simplified. In addition, because this mapping is reversible, the RL agent obtains the property of total control over the original MDP, that is, for every possible action, there is a point on the Gaussian distribution that maps to that action, and the It is.
The point of this thesis is to propose PARROT, a framework that can accelerate learning to acquire new skills by learning behavioral prior to learning from multiple multi-task datasets. In the next section, we will describe the methodology in detail.
To read more,
Please register with AI-SCHOLAR.
ORCategories related to this article