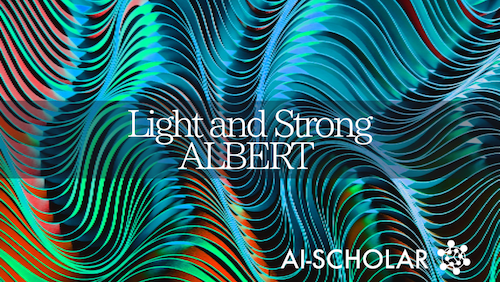
BERT Is Still Evolving! The Lighter, Stronger ALBERT Is Here!
Three key points
✔️Two improvements to the structure of BERT, resulting in significant parameter reduction
✔️Improved learning tasks that were previously considered ineffective in BERT, resulting in more grammar-capturing learning
✔️Improved performance as well as speed through parameter reduction
ALBERT: A Lite BERT for Self-supervised Learning of Language Representations
written by Zhenzhong Lan, Mingda Chen, Sebastian Goodman, Kevin Gimpel, Piyush Sharma, Radu Soricut
(Submitted on 26 Sep 2019 (v1), last revised 9 Feb 2020 (this version, v6))
Comments: Published by ICLR 2020
Subjects: Computation and Language (cs.CL); Artificial Intelligence (cs.AI)
first of all
One of the current trends in natural language processing is to use pre-training with language model-based mechanisms such as ELMo, BERT, and XLNet to improve the performance of various tasks. We have been following this trend and have introduced BERT and its improved versions, ERNIE, XLNet and RoBERTa.
Pre-training using such methods can contribute to significant performance gains in tasks such as QA tasks. On the other hand, BERT-based models are known to have a very large number of parameters and take a huge amount of time to train. In addition, BERT is structurally difficult to train, and its performance decreases as the number of parameters increases. Furthermore, the need for Next Sentence Prediction (NSP), which is included in BERT training, has been discussed for a long time as it does not contribute to the performance improvement.
ALBERT (A Lite BERT) solves these problems by significantly reducing the number of parameters and correspondingly increasing the learning speed. These parameter reductions also act as constraints on task learning, making BERT more efficient at learning, and ultimately improving performance. Furthermore, by incorporating new task learning alternatives to NSP, we have achieved more effective acquisition of " understanding of contextual coherence ", which was originally attempted to be learned by NSP.
To read more,
Please register with AI-SCHOLAR.
ORCategories related to this article