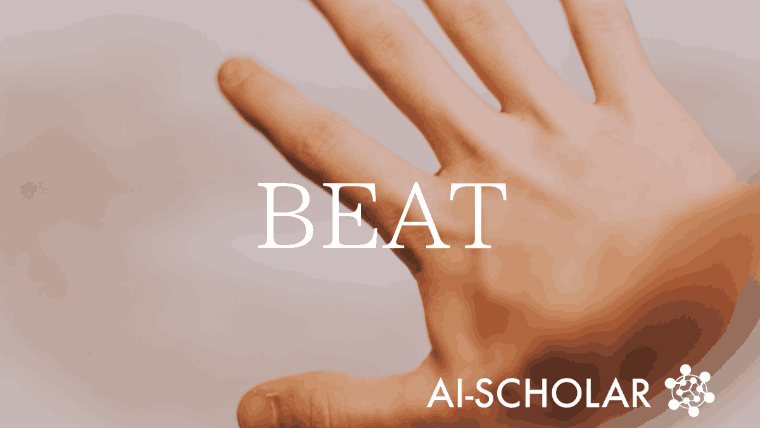
BEAT, A Large Data Set For Generating More Human-like Realistic Gestures, Is Now Available!
3 main points
✔️ Construct BEAT (Body-Expression-Audio-Text Dataset), a large-scale multimodal dataset for more human-like gesture generation
✔️ Proposed CaMN (Cascaded Motion Network), a baseline model for gesture generation using BEAT
✔️ Introducing SRGR (Semantic Relevance Gesture Recall), a metric to evaluate the diversity of generated gestures
BEAT: A Large-Scale Semantic and Emotional Multi-Modal Dataset for Conversational Gestures Synthesis
written by Haiyang Liu, Zihao Zhu, Naoya Iwamoto, Yichen Peng, Zhengqing Li, You Zhou, Elif Bozkurt, Bo Zheng
(Submitted on 10 Mar 2022 (v1), last revised 19 Apr 2022 (this version, v4))
Comments: ECCV 2022
Subjects: Computer Vision and Pattern Recognition (cs.CV); Computation and Language(cs.CL); Graphics(cs.GR); Machine Learning(cs.LG); Multimedia(cs.MM)
code:![]()
The images used in this article are from the paper, the introductory slides, or were created based on them.
first of all
Realizing more human-like gesture generation using multimodal data is a very important technology in the fields of animation, entertainment, and VR, and various methods have been proposed.
To achieve such realistic gesture generation, various factors such as voice, facial expression, emotion, and speaker identity need to be considered in the design of the model.
Although there has been extensive research on speech- and text-based gesture generation, such gesture generation has been an open problem due to the lack of available large datasets, models, and standard evaluation metrics.
This paper describes a paper that successfully solves the above problem and performs more human-like gesture generation with the following contributions.
- Building BEAT (Body-Expression-Audio-Text Dataset ), a large-scale multimodal dataset for more human-like gesture generation
- A baseline model for gesture generation using BEAT, CaMN (Cascaded Motion Network ), is proposed.
- Introducing SRGR (Semantic Relevance Gesture Recall ), a metric for evaluating the diversity of generated gestures
Let's take a look at each of them.
BEAT: Body-Expression-Audio-Text Dataset
As mentioned above, the lack of large, high-quality multimodal datasets with semantic and affective annotations is an obstacle to achieving human-like gesture generation, and The methods in existing research are trained on limited motion capture datasets and pseudo-label datasets, and thus lack robustness.
To address these data-related issues, this paper presents a 76-hour high-quality multimodal dataset of 30 speakers, acquired from 30 speakers conversing in four different languages with eight other emotions in four different modalities of Body-Expression-Audio-Text, called theBEAT (Body-Expression-Audio-Text Dataset ) was constructed.
The details of BEAT are shown in the figure below.
- A 16-camera motion capture system was employed to record data from the conversation and self-talk sessions, as shown in (a)
- In the conversation session, gestures are classified into four categories: Talking, Silence, Reaction, and Asking, as shown in (b)
- In the self-talk session, seven categories of emotions, Neutral, Anger, Happiness, Fear, Disgust, Sadness, Contempt, and Suprise, are set in equal proportions, as shown in (c)
- The dataset also contains data in four languages, mainly English, with different recording times, by 30 speakers from 10 countries, as shown in (e)
The table below also compares BEAT(Ours) to the existing dataset, with green highlights indicating the best values and yellow highlights the second best values.
Thus, it can be seen that the dataset in this paper is the largest motion capture dataset containing multimodal data and annotations.
Multi-Modal Conditioned Gestures Synthesis Baseline
In this paper, we propose a multimodal gesture generation baseline, CaMN (Cascaded Motion Network ), with all modalities as input for more human-like gesture generation.
CaMN encodes the weights of text, emotion labels, speaker ID, speech, and facial blend shapes (one of the animation methods), which are reconstructed into body and hand gestures by two cascaded LSTM + MLP decoders, as shown in the figure below.
The network selection of text, speech, and speaker ID encoders is based on existing research and customized for better performance.
The weights of the gesture and face blendshapes are downsampled to 30 FPS, and the word sentences are inserted with padding tokens to correspond to the silence time of the audio.
Metric for Gesture Diversity
In this paper, we propose a new metric for evaluating gesture diversity, called Semantic-Relevant Gesture Recall (SRGR ). SRGR utilizes semantic score as a weight of Probability of Correct Keypoint (PCK) between generated gestures and ground truth gestures. Here PCK represents the number of joints successfully recalled for a given threshold δ. SRGR can be calculated as follows.
The authors believe that SRGR, which emphasizes gesture recall, is more consistent with human subjectivity about gesture diversity than L1 Diversity, which is an existing metric. The authors believe that the SRGR, which emphasizes the recall of gestures, is more consistent with human subjectivity about gesture diversity than the existing L1 Diversity measure.
Experiments
In this paper, we first validate a novel evaluation index, SRGR, and then verify the data quality of BEAT based on subjective experiments and compare the proposed model with existing methods.
Validness of SRGR
To validate the effectiveness of the SRGR, a user study was conducted under the following conditions
- Motion sequences were randomly cut into clips of around 40 seconds and participants were asked to rate each clip based on gesture variety
- A total of 160 participants each scored 15 random gesture clips based on the gesture itself, not the content of the speech
- All the questionnaire items were on a 5-point Likert scale, and users' subjective scores for gesture variety and attractiveness were calculated respectively
The results are shown in the left figure below, indicating a strong correlation between the attractiveness of a gesture and its diversity.
More interestingly, the graph on the right side of the figure shows that SRGR is more similar to human senses in assessing gesture diversity compared to L1 Diversity.
Data Quality
In this paper, to evaluate the quality of BEAT, a novel dataset, we used Trinity, a dataset widely used in existing research, as a comparison target. Each dataset was split into a 19:2:2 ratio and each was used as training/validation/comparison data and compared using the existing methods S2G and audio2gestures.
Body Correctness (accuracy of body gestures), Hands Correctness (accuracy of hand gestures), Diversity (diversity of gestures), and Synchrony (synchrony of gestures and speech ) for each dataset. and the results are shown in the table below.
The table shows that BEAT(Ours) is highly rated in all aspects, demonstrating that this dataset is far superior to Trinity.
Evaluation of the baseline model
To validate the performance of CaMN, the model proposed in this paper, a comparative verification with the existing methods, Seq2Seq, S2G, A2G andMultiContext, was performed under the following conditions.
- Train the entire dataset for 15 hours with a learning rate of 2e-4 using the Adam optimizer
- Since L1 is unsuitable for gestureFGD was adopted as the evaluation index because it has been shown that L1 is not suitable for evaluating the performance of gestures.
- Besides, to evaluate the diversity of gestures and synchronization with speech, we employed the SRGR proposed in this paper and an existing index, BeatAlign
The verification results are shown in the table below.
Thus, it was demonstrated that CaMN scores the highest on all evaluation metrics.
An example of a gesture generated by CaMN is shown below.
The man on the right shows a sample of a ground truth gesture (above) and a CaMN-generated gesture (left), and you can see that it generates a very plausible gesture.
More interestingly, CaMN also allows the stylistic transformation of gestures by emotion. The man on the left shows an example of stylistic transformation from a neural gesture (top) to a gesture with a near-emotion (bottom).
summary
How was it? In this article, we explained the paper that proposed BEAT, which is a large-scale dataset for more human-like gesture generation, CaMN, which is a novel baseline model using BEAT, and SRGR, which is its evaluation index. This paper enables more realistic gesture generation compared to existing methods and is expected to be applied to various fields such as animation and VR.
On the other hand, since SRGR is calculated based on semantic annotation in this study, there are some issues such as its limitation in unlabeled data sets.
The details of the architecture of the datasets and models introduced in this article can be found in this paper if you are interested.
Categories related to this article