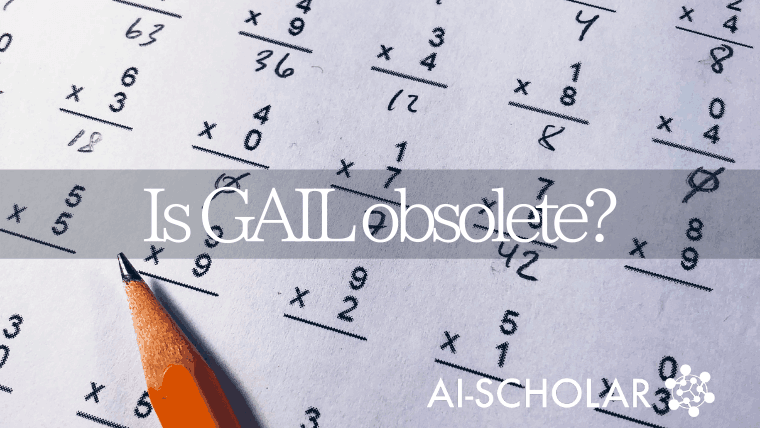
Task-Relevant Adversarial Imitation Learning: Is GAIL Obsolete?
3 main points
✔️ Causal Confusion causes GAIL to perform poorly
✔️ Propose TRAIL that constrains the discriminator to use task-relevant features
✔️ Shows better performance on various manipulation tasks than other comparative methods
Task-Relevant Adversarial Imitation Learning
written by Konrad Zolna, Scott Reed, Alexander Novikov, Sergio Gomez Colmenarejo, David Budden, Serkan Cabi, Misha Denil, Nando de Freitas, Ziyu Wang
(Submitted on 2 Oct 2019)
Subjects: Machine Learning (cs.LG); Artificial Intelligence (cs.AI); Robotics (cs.RO); Machine Learning (stat.ML)![]()
![]()
First of all
Here is a paper that was accepted for CoRL 2020. In recent years, Generative Adversarial Networks (GANs) have attracted a lot of attention in image generation. Using a similar mechanism, a method called Generative Adversarial Imitation Learning (GAIL) is an imitation learning method in which a discriminator learns to distinguish the behavior of an expert from that of an agent. By using GAIL, we can solve the problem of search, which is necessary in ordinary RL (reinforcement learning), and we can solve the task. However, GAIL does not give as good results as GAN. However, GAIL does not give as good results as GAN. Especially, it is said that it is difficult to learn control policies for robots from images.
So what is the problem? Causal Confusion is thought to be the reason. Causal Confusion is a phenomenon in which a system acts on a false causal relationship, and is likely to occur when there is a lot of information in the environment, for example, when there are many unnecessary objects. In particular, when the number of expert data is small, it is said that false causal relationships are often learned. The figure below illustrates this phenomenon. The vertical axis shows the features related to the task, and the horizontal axis shows the features not related to the task. At the beginning of the training, both features are used to classify the expert and agent data, but as the training progresses, we can see that the data is classified using features that are not related to the task.
To read more,
Please register with AI-SCHOLAR.
ORCategories related to this article