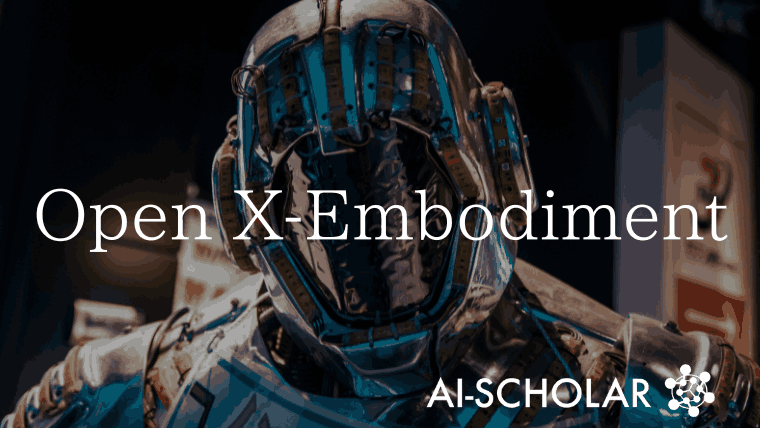
Open X-Embodiment: Towards A Generic Robot Learning
3 main points
✔️ Aiming to learn a versatile robot
✔️ Learning based on data from 22 different robots from 21 different institutions
✔️ Creating a dataset that can be used to train robots in the future
Open X-Embodiment: Robotic Learning Datasets and RT-X Models
written by Open X-Embodiment Collaboration, Abhishek Padalkar, Acorn Pooley, Ajay Mandlekar, Ajinkya Jain, Albert Tung, Alex Bewley, Alex Herzog, Alex Irpan, Alexander Khazatsky, Anant Rai, Anikait Singh, Animesh Garg, Anthony Brohan, Antonin Raffin, Ayzaan Wahid, Ben Burgess-Limerick, Beomjoon Kim, Bernhard Schölkopf, Brian Ichter, Cewu Lu, Charles Xu, Chelsea Finn, Chenfeng Xu, Cheng Chi, Chenguang Huang, Christine Chan, Chuer Pan, Chuyuan Fu, Coline Devin, Danny Driess, Deepak Pathak, Dhruv Shah, Dieter Büchler, Dmitry Kalashnikov, Dorsa Sadigh, Edward Johns, Federico Ceola, Fei Xia, Freek Stulp, Gaoyue Zhou, Gaurav S. Sukhatme, Gautam Salhotra, Ge Yan, Giulio Schiavi, Gregory Kahn, Hao Su, Hao-Shu Fang, Haochen Shi, Heni Ben Amor, Henrik I Christensen, Hiroki Furuta, Homer Walke, Hongjie Fang, Igor Mordatch, Ilija Radosavovic, Isabel Leal, Jacky Liang, Jad Abou-Chakra, Jaehyung Kim, Jan Peters, Jan Schneider, Jasmine Hsu, Jeannette Bohg, Jeffrey Bingham, Jiajun Wu, Jialin Wu, Jianlan Luo, Jiayuan Gu, Jie Tan, Jihoon Oh, Jitendra Malik, Jonathan Booher, Jonathan Tompson, Jonathan Yang, Joseph J. Lim, João Silvério, Junhyek Han, Kanishka Rao, Karl Pertsch, Karol Hausman, Keegan Go, Keerthana Gopalakrishnan, Ken Goldberg, Kendra Byrne, Kenneth Oslund, Kento Kawaharazuka, Kevin Zhang, Krishan Rana, Krishnan Srinivasan, Lawrence Yunliang Chen, Lerrel Pinto, Li Fei-Fei, Liam Tan, Lionel Ott, Lisa Lee, Masayoshi Tomizuka, Max Spero, Maximilian Du, Michael Ahn et al. (83 additional authors not shown)
(Submitted on 13 Oct 2023 (v1), last revised 18 Dec 2023 (this version, v4))
Comments: Published on arxiv.
Subjects: Robotics (cs.RO)
code:![]()
The images used in this article are from the paper, the introductory slides, or were created based on them.
Introduction
In the field of language and vision models, models have been developed for a variety of tasks using pre-learning models.
In the field of robotics, on the other hand, learning methods that are specific to a particular application, robot, or environment have been the norm. Therefore, the challenge is to develop learning models, such as language and vision models, that can be universally accurate for different robots and environments.
The goal of this study was to improve the accuracy of the robot's movements in a generic manner using RT-X models trained on large data sets collected from multiple robots and environments.
As a result of this study, the RT-X model successfully leveraged data collected on different robots and demonstrated highly accurate behavior on multiple robots. This demonstrates the potential in robotics to create highly accurate models for any robot, environment, or task.
Overview of Open X-Embodiment
In this study, data collected from 22 different robots from 21 institutions were combined to create the Open-X Embodiment Dataset. This dataset contains 527 different skills and 160,266 different tasks. As a result, the dataset reflects a very wide range of robot operation scenarios, allowing for diverse and comprehensive robot learning.
The goals of this study are twofold
- Show that using an integrated dataset of data from several different robots and environments performs better than models trained on individual pieces of data.
- Build a dataset that can be used to train future large-scale robots.
The RT-X model is based on the Open-X Embodiment Dataset created in this study and uses a Transformer-based architecture that allows knowledge learned on one robot to be applied to another robot. The RT-X model uses a Transformer-based architecture that allows knowledge learned on one robot to be applied to another robot.
Here, RT-1 (Robotics Transformer 1) and RT-2 (Robotics Transformer 2) are further trained on RT-X.
RT-1 is a model learned by imitation learning of a large-scale demonstration of a task of grasping various objects, using images and verbal instructions as input.
RT-2 is a vision-language-action (VLA) model trained by simultaneous fine-tuning with web data and robotics data.
In the following, we will refer to RT-1 and RT-2 trained in the Open X-Embodiment Dataset as RT-1-X and RT-2-X, respectively.
For more information about RT-1, please see this article.
Experimental results
In the experiment, 3600 evaluation tests were conducted on six different robots to observe how the performance of each model differed.
Results of performance evaluation at different scales
First, let's look at the case of small data sets. The figure above compares the performance of the model on each dataset.
The performance of RT-1-X outperformed the methods trained on each of the robots' datasets on four of the five datasets. Additionally, the average success rate of RT-1-X was 50% higher than RT-1 and the other models.
The experimental results show that for small data sets, co-training in X-Embodiment Data yields significant results.
Next, let's look at the case of large data sets. The table above compares the performance of each model on large data sets. On the large data set, the RT-1-X model did not outperform the RT-1 in accuracy.
However, the performance of the larger RT-2-X model outperformed both the model trained on the respective data set and RT-1. These results suggest that a sufficiently large architecture can improve performance in areas where there is sufficient data to train the robot.
Improved response to tasks not in the data
Next, we will look at how the training model using the X-Embodiment Dataset responds to settings that are not in the data and to newer, more complex instructions. In this experiment, we will use the RT-2-X model, restricting it to the large data domain. The results are shown in the table above.
Generalization performance for unknown objects and backgrounds can be determined from the RT-2 Generalization Evaluation values on the right side of the table. From rows (1) and (2) of the table, we see that RT-2 and RT-2-X are almost equal, with 62% and 61%, respectively.
On the other hand, let's look at how well they can handle tasks they have never learned. This can be determined from the Emergent Skills Evaluation entry in the table above. Comparing rows (1) and (2), RT-2-X outperforms RT-2 by about three times in the Emergent Skills Evaluation.
With about three times the performance, RT-2-X is now able to determine even the smallest differences in prepositions. For example, RT-2 could not grasp the difference between prepositions such as on and near in instructions, but RT-2-X is now able to distinguish even such slight differences in prepositions. The results of this experiment indicate that by incorporating data from other robots into its training, RT-2-X may be able to handle tasks that it could not handle in the past.
Elsewhere, the table reveals the following
- Comparison of (4) and (5) shows that generalization performance is better when history is included.
- Comparison of (4) and (6) shows that pre-training on Web data improves generalization performance.
- A comparison of (2) and (4) shows that the larger the model size, the better the Emergent Skills Evaluation.
The results of these experiments suggest that the use of large data sets that integrate data from different robots can improve the performance of each individual robot.
Summary
The study presented an integrated dataset containing 527 skills and 160,266 tasks collected from 22 different robots from 21 institutions, and evaluated models that use the data.
The results showed that RT-1-X had a 50% higher success rate than the methods from the respective data sets provided by other institutions, and RT-2-X, a larger vision-language model-based model, had approximately 3 times better generalization performance than RT-2.
Experimental results with the RT-X model show the potential for robot learning to improve accuracy in a generic way, but there are some challenges at this stage.
For example, in this case, we have not taken into account robots with very different sensors and actuators.
It is hoped that a general-purpose robot learning method will be established while solving these issues one by one.
Categories related to this article