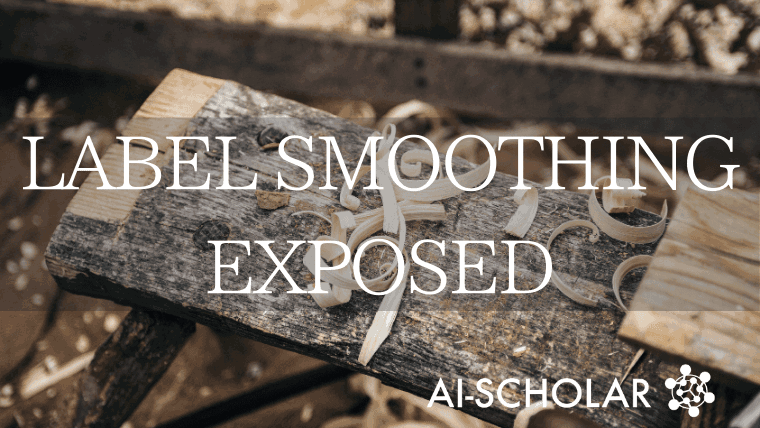
The Truth Behind Label Smoothing!
3 Main Points
✔️ Relationship between label smoothing and loss-correction techniques
✔️ Effect of label smoothing on label noise
✔️ Applications of label smoothing in knowledge distillation with noisy labels
Does label smoothing mitigate label noise?
written by Michal Lukasik,Srinadh Bhojanapalli,Aditya Krishna Menon,Sanjiv Kumar
(Submitted on 5 Mar 2020)
Comments: Accepted to arXiv.
Subjects: Machine Learning (cs.LG); Machine Learning (stat.ML)![]()
Introduction
To put it simply, label smoothing is the process of mixing a uniform vector to the available training labels and making them “softer”. It is a technique commonly employed while training neural networks in the presence of noisy labels. Neural networks can fit noisy labels easily. So it is natural to think that label smoothing tackles noisy labels by reducing overconfidence in a particular example. Nevertheless, it can also worsen the problem by injecting additional uniform noise to all the labels! A double-edged sword. Well then, does label smoothing really affect the robustness of deep networks? If it does, how so? This paper tries to explain how and when label smoothing can improve your model's performance.
To read more,
Please register with AI-SCHOLAR.
ORCategories related to this article